Optimizing the Spatial Configuration of Mesoscale Environmental Monitoring Networks Using a Geometric Approach
JOURNAL OF ATMOSPHERIC AND OCEANIC TECHNOLOGY(2020)
摘要
The ability of mesoscale environmental monitoring networks to collect spatially unbiased observations and to detect mesoscale environmental phenomena is radically determined by the spatial configuration of the network. However, there is lack of an objective, practical, and amenable method for guiding the spatial configuration of multifunctional, long-term mesoscale networks. The objective of this study is to present and demonstrate the application of a new method based on computational geometry that identifies the optimal location of future monitoring stations by finding the largest unmonitored area of the network. The computation of the method is first illustrated using the spatial distribution of the Kansas Mesonet as a case-study scenario and is then applied to several statewide and nationwide mesoscale networks in the United States. The proposed geometric method was effective to generate a long-term road map with the location of future monitoring stations. The geometric method seamlessly integrated with georeferenced data to identify the largest unmonitored areas with frequent occurrence of wildland fires and severe drought and to identify underrepresented soil types. Spatially dense statewide mesoscale networks with >120 stations across the studied U.S. states resulted in largest unmonitored areas of about 60(2) km(2), whereas nationwide networks had largest unmonitored areas of 500(2)-600(2) km(2). The proposed method based on the geometric arrangement of network stations can be used by scientists, network managers, and state climatologists to improve the spatial representability of existing networks, better plan the allocation of limited resources, and increase the preparedness potential of mesoscale networks.
更多查看译文
关键词
Mesoscale processes,Mesoscale systems,Mesoscale systems,Soil moisture,In situ atmospheric observations,Surface observations
AI 理解论文
溯源树
样例
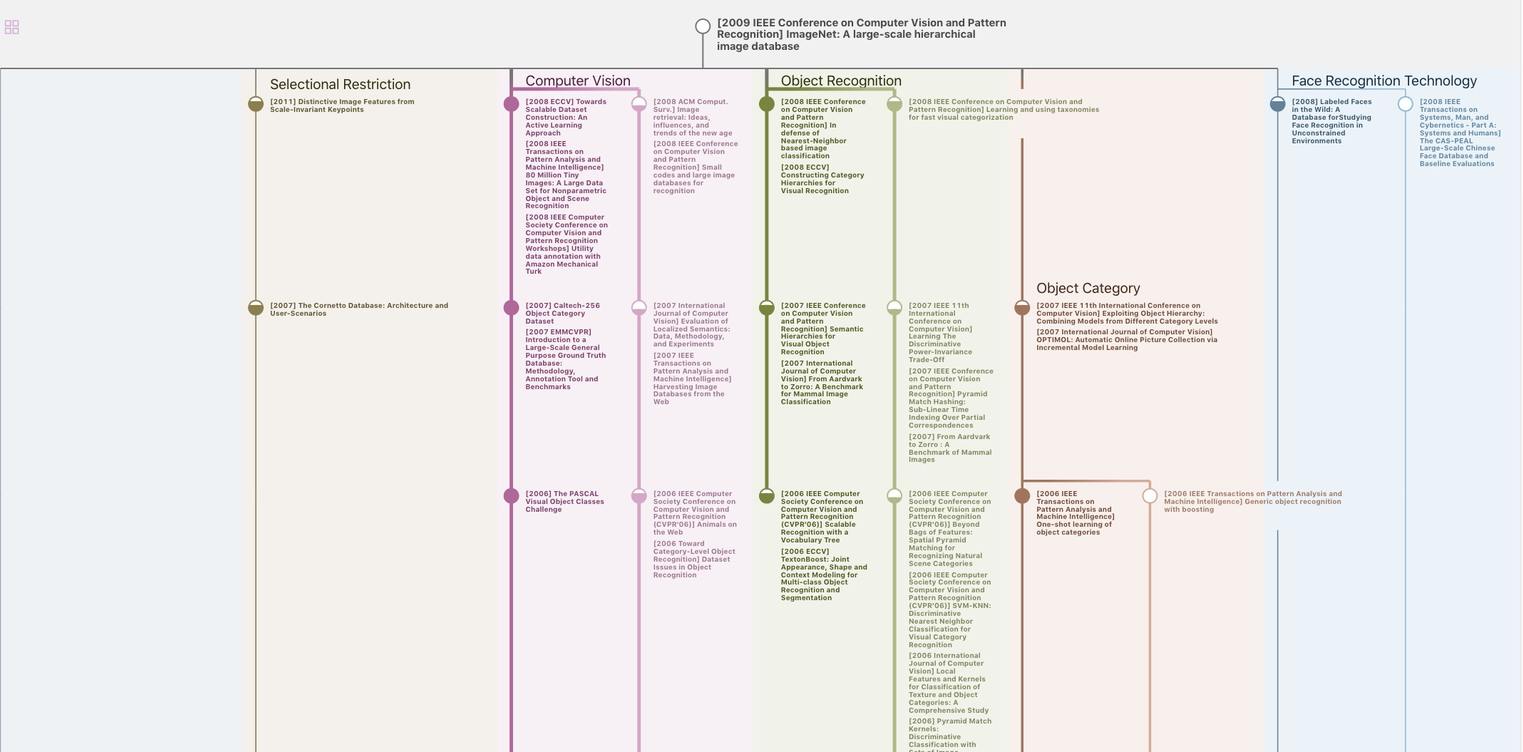
生成溯源树,研究论文发展脉络
Chat Paper
正在生成论文摘要