Multivariate Feature Extraction Based Supervised Machine Learning For Fault Detection And Diagnosis In Photovoltaic Systems
EUROPEAN JOURNAL OF CONTROL(2021)
摘要
Fault detection and diagnosis (FDD) in the photovoltaic (PV) array has become a challenge due to the magnitudes of the faults, the presence of maximum power point trackers, non-linear PV characteristics, and the dependence on isolation efficiency. Thus, the aim of this paper is to develop an improved FDD technique of PV systems faults. The common FDD technique generally has two main steps: feature extraction and selection, and fault classification. Multivariate feature extraction and selection is very important for multivariate statistical systems monitoring. It can reduce the dimension of modeling data and improve the monitoring accuracy. Therefore, in the proposed FDD approach, the principal component analysis (PCA) technique is used for extracting and selecting the most relevant multivariate features and the supervised machine learning (SML) classifiers are applied for faults diagnosis. The FDD performance is established via different metrics using data extracted from different operating conditions of the gridconnected photovoltaic (GCPV) system. The obtained results confirm the feasibility and effectiveness of the proposed approaches for fault detection and diagnosis.(c) 2020 European Control Association. Published by Elsevier Ltd. All rights reserved.
更多查看译文
关键词
Supervised machine learning (SML), Principal component analysis (PCA), Photovoltaic (PV) systems, Feature extraction, Fault diagnosis, Fault classification
AI 理解论文
溯源树
样例
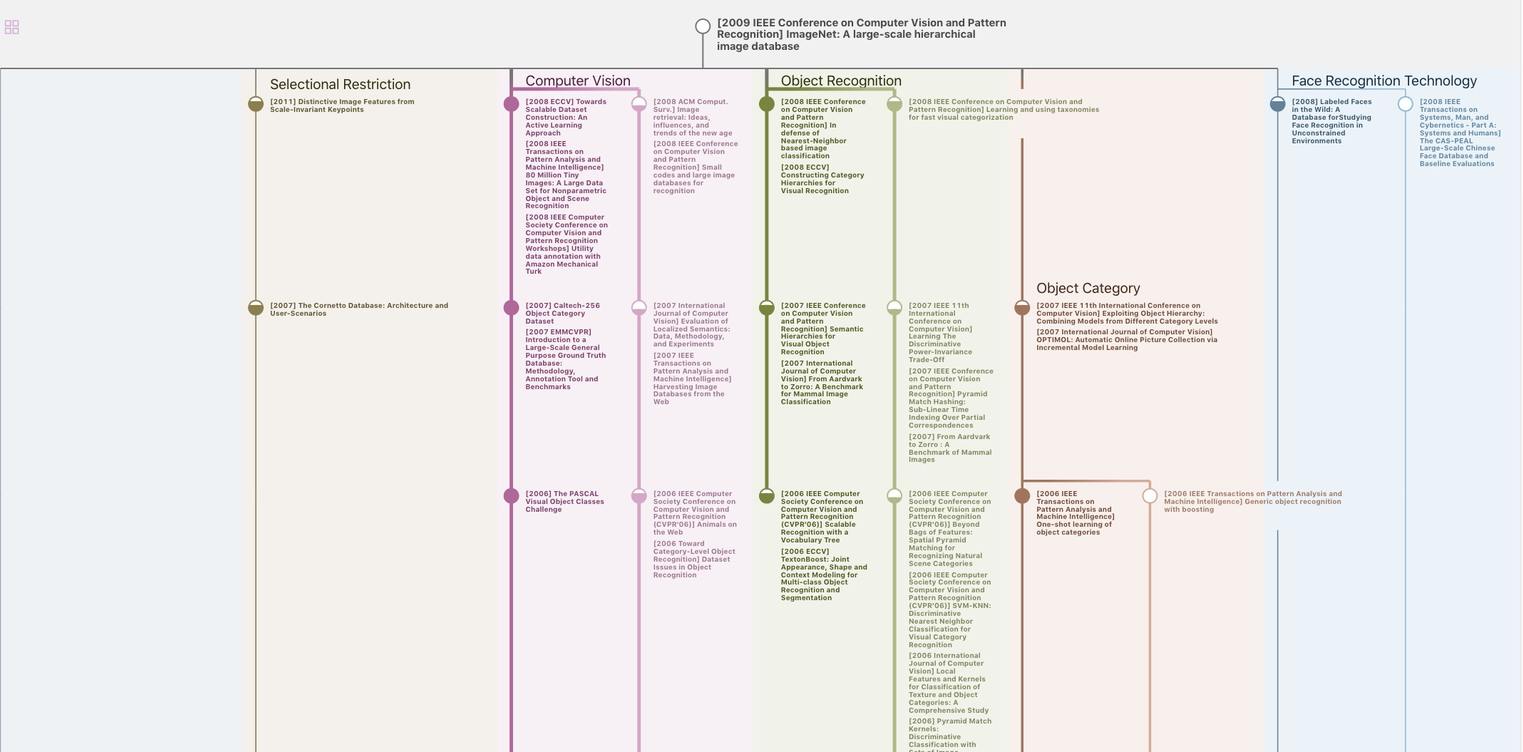
生成溯源树,研究论文发展脉络
Chat Paper
正在生成论文摘要