Towards An Ai-Driven Real Time Verification Of Radiotherapy Treatments
2019 IEEE NUCLEAR SCIENCE SYMPOSIUM AND MEDICAL IMAGING CONFERENCE (NSS/MIC)(2019)
摘要
Monolithic Active Pixel Sensor (MAPS) devices are an effective tool for upstream verification of Intensity Modulated Radio Therapy treatments. It is crucial to measure with high precision the positions of the Multi Leaf Collimators (MLC) used to shape the beam, in order to enhance the quality and safety of treatments. This work describes a two-step procedure applied to the problems of leaf detection and position reconstruction. This procedure represents the building block towards the definition of a real-time verification device capable of detecting and reconstructing the position of the MLC used in the everyday radiotherapy treatments. A Fully Convolutional Neural Network model is used to analyse the high-resolution images produced by Lassena MAPS devices in order to automatically detect the leaves. A Random Forest regression model is then applied to estimate the positions of the detected leaves. The dataset used in the experiments contains 1200 single-leaf images, referring to four leaf positions in the range 1 divided by 25 mm, split in training and test sets with the 80% and 20% of the total samples, respectively. Performance have been evaluated using the Dice loss coefficient for leaf detection, and mean squared error (MSE) as for leaf position estimation. The proposed approach obtained an average dice loss coefficient of 0.85 +/- 0.03 on the images in the test set; the MLC positions are estimated with a MSE of 0.04, and a resolution of 68 +/- 2 mu m on the same images.
更多查看译文
关键词
random forest regression model,single-leaf images,leaf position estimation,intensity modulated radiotherapy treatments,multileaf collimators,position reconstruction,fully convolutional neural network model,high-resolution images,Lassena MAPS devices,monolithic active pixel sensor devices,Dice loss coefficient
AI 理解论文
溯源树
样例
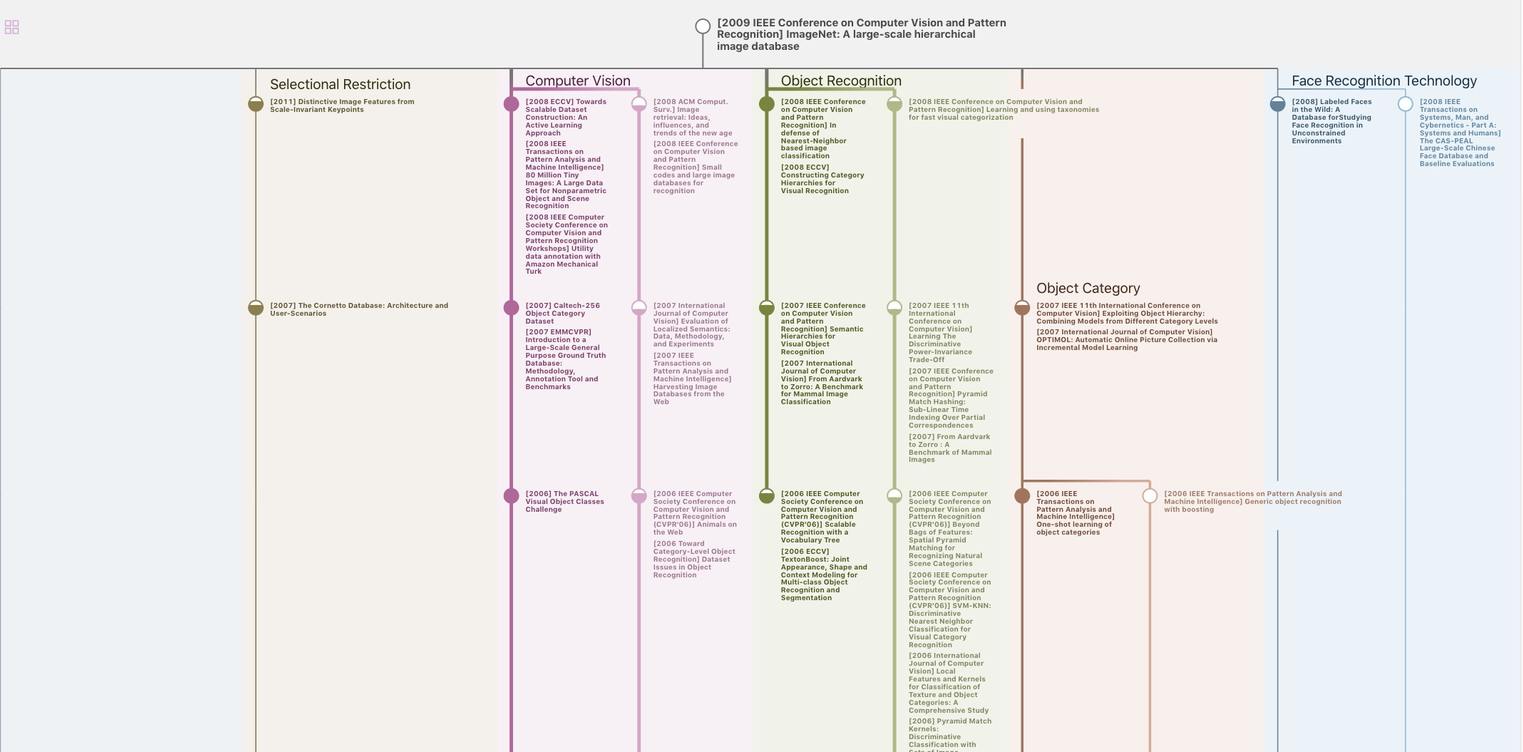
生成溯源树,研究论文发展脉络
Chat Paper
正在生成论文摘要