Generalized Graph Spectral Sampling With Stochastic Priors
2020 IEEE INTERNATIONAL CONFERENCE ON ACOUSTICS, SPEECH, AND SIGNAL PROCESSING(2020)
摘要
We consider generalized sampling for stochastic graph signals. The generalized graph sampling framework allows recovery of graph signals beyond the bandlimited setting by placing a correction filter between the sampling and reconstruction operators and assuming an appropriate prior. In this paper, we assume the graph signals are modeled by graph wide sense stationarity (GWSS), which is an extension of WSS for standard time domain signals. Furthermore, sampling is performed in the graph frequency domain along with the assumption that the graph signals lie in a periodic graph spectrum subspace. The correction filter is designed by minimizing the mean-squared error (MSE). The graph spectral response of the correction filter parallels that in generalized sampling for WSS signals. The effectiveness of our approach is validated via experiments by comparing the MSE with existing approaches.
更多查看译文
关键词
Graph signal processing, spectral domain sampling, minimal reconstruction error, Wiener filter
AI 理解论文
溯源树
样例
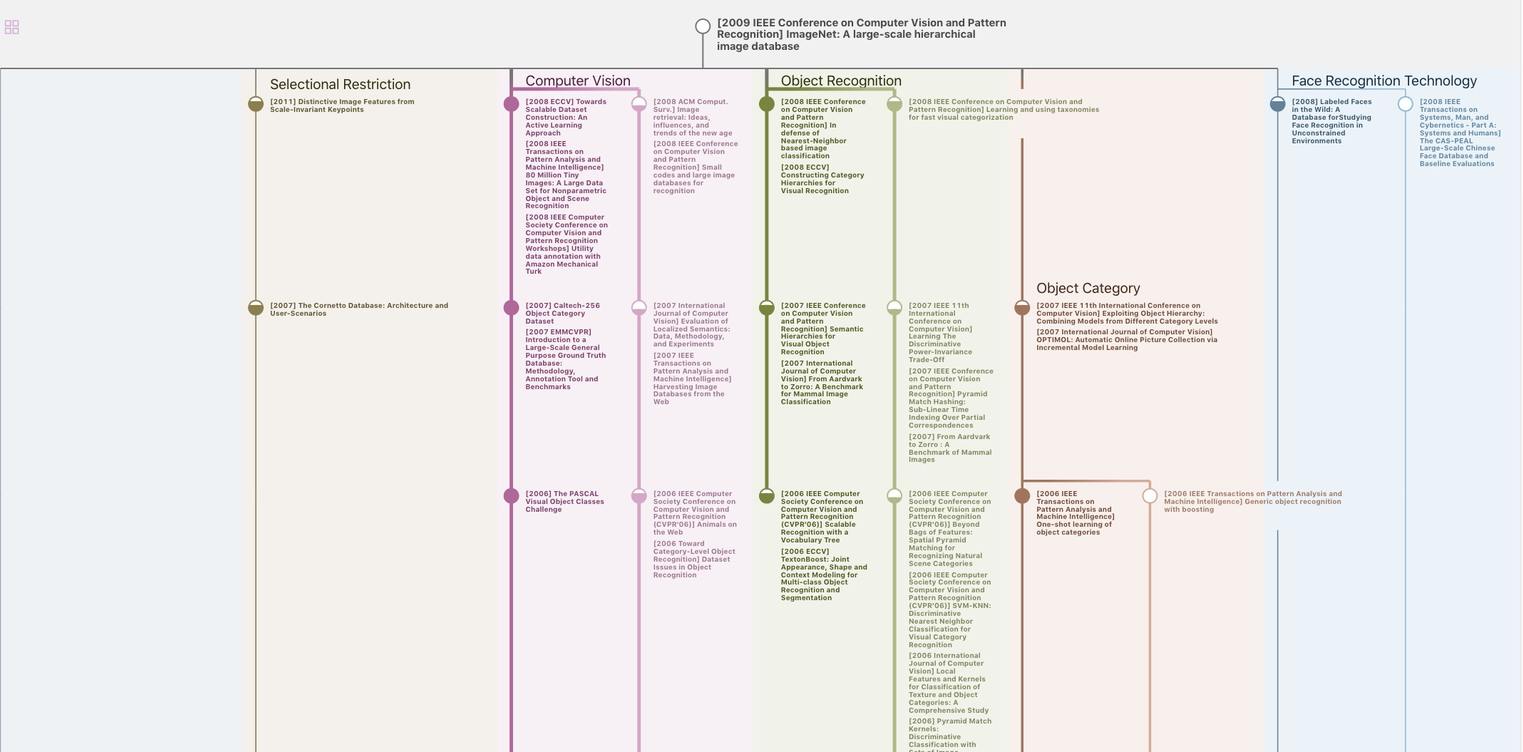
生成溯源树,研究论文发展脉络
Chat Paper
正在生成论文摘要