Improving Spoken Question Answering Using Contextualized Word Representation
2020 IEEE INTERNATIONAL CONFERENCE ON ACOUSTICS, SPEECH, AND SIGNAL PROCESSING(2020)
摘要
While question answering (QA) systems have witnessed great breakthroughs in reading comprehension (RC) tasks, spoken question answering (SQA) is still a much less investigated area. Previous work shows that existing SQA systems are limited by catastrophic impact of automatic speech recognition (ASR) errors [1] and the lack of large-scale real SQA datasets [2]. In this paper, we propose using contextualized word representations to mitigate the effects of ASR errors and pretraining on existing textual QA datasets to mitigate the data scarcity issue. New state-of-the-art results have been achieved using contextualized word representations on both the artificially synthesised and real SQA benchmark data sets, with 21.5 EM/18.96 F1 score improvement over the sub-word unit based baseline on the Spoken-SQuAD [1] data, and 13.11 EM/10.99 F1 score improvement on the ODSQA data [2]. By further fine-tuning pre-trained models with existing large scaled textual QA data, we obtained 38.12 EM/34.1 F1 improvement over the baseline of fine-tuned only on small sized real SQA data.
更多查看译文
关键词
contextual word representation, spoken question answering, supervised pre-training, BERT
AI 理解论文
溯源树
样例
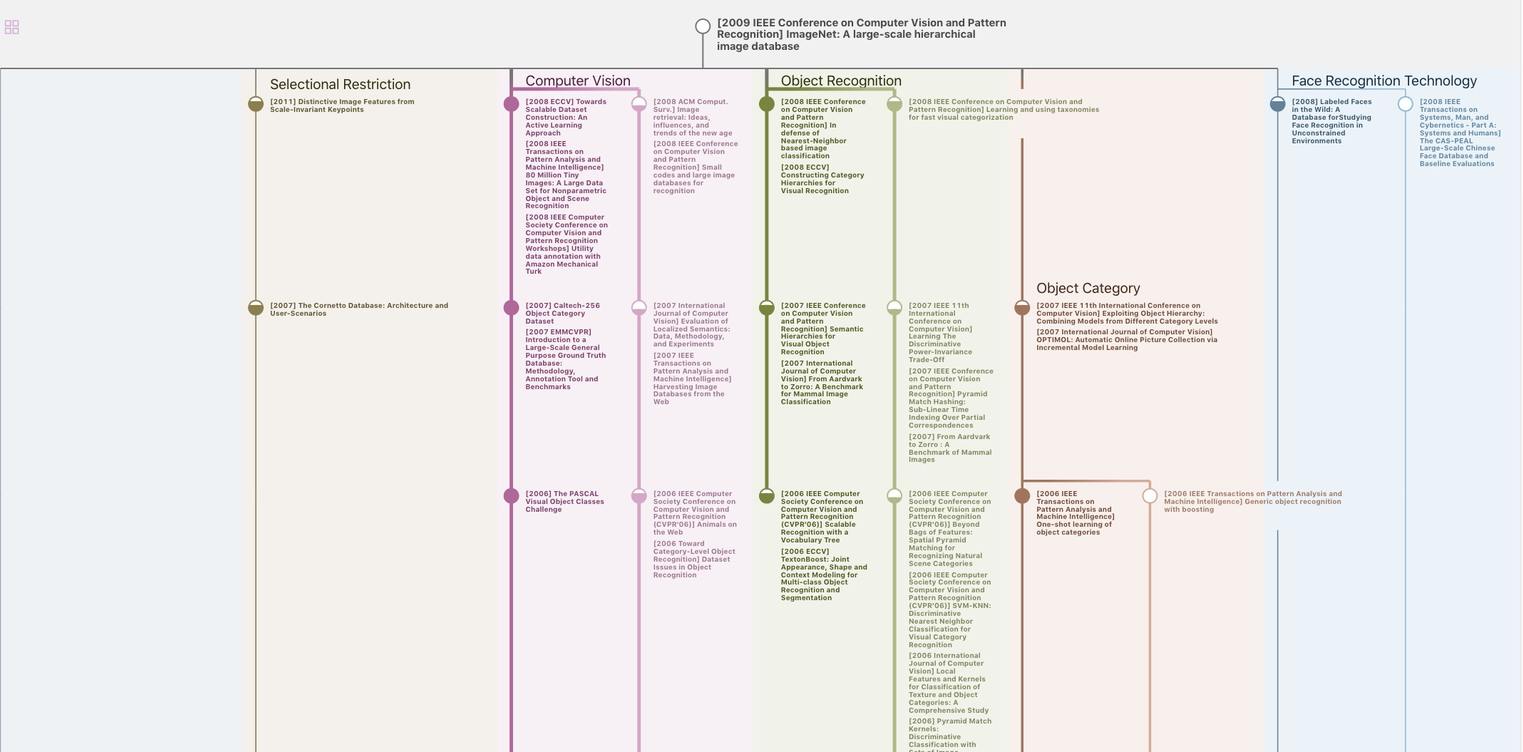
生成溯源树,研究论文发展脉络
Chat Paper
正在生成论文摘要