Improving Sample-Efficiency In Reinforcement Learning For Dialogue Systems By Using Trainable-Action-Mask
2020 IEEE INTERNATIONAL CONFERENCE ON ACOUSTICS, SPEECH, AND SIGNAL PROCESSING(2020)
摘要
By interacting with human and learning from reward signals, reinforcement learning is an ideal way to build conversational AI. Concerning the expenses of real-users' responses, improving sample-efficiency has been the key issue when applying reinforcement learning in real-world spoken dialogue systems (SDS). Handcrafted action masks are commonly used to rule out impossible actions and accelerate the training process. However, the handcrafted action mask can barely be generalized to unseen domains. In this paper, we propose trainable-action-mask (TAM) which learns from data automatically without handcrafting complicated rules. In our experiments in Cambridge Restaurant domain, TAM requires only 30% of training data, compared with the baseline, to reach the 80% success rate and it also shows robustness to noisy environments.
更多查看译文
关键词
model-based reinforcement learning, sample-efficiency, spoken dialogue systems
AI 理解论文
溯源树
样例
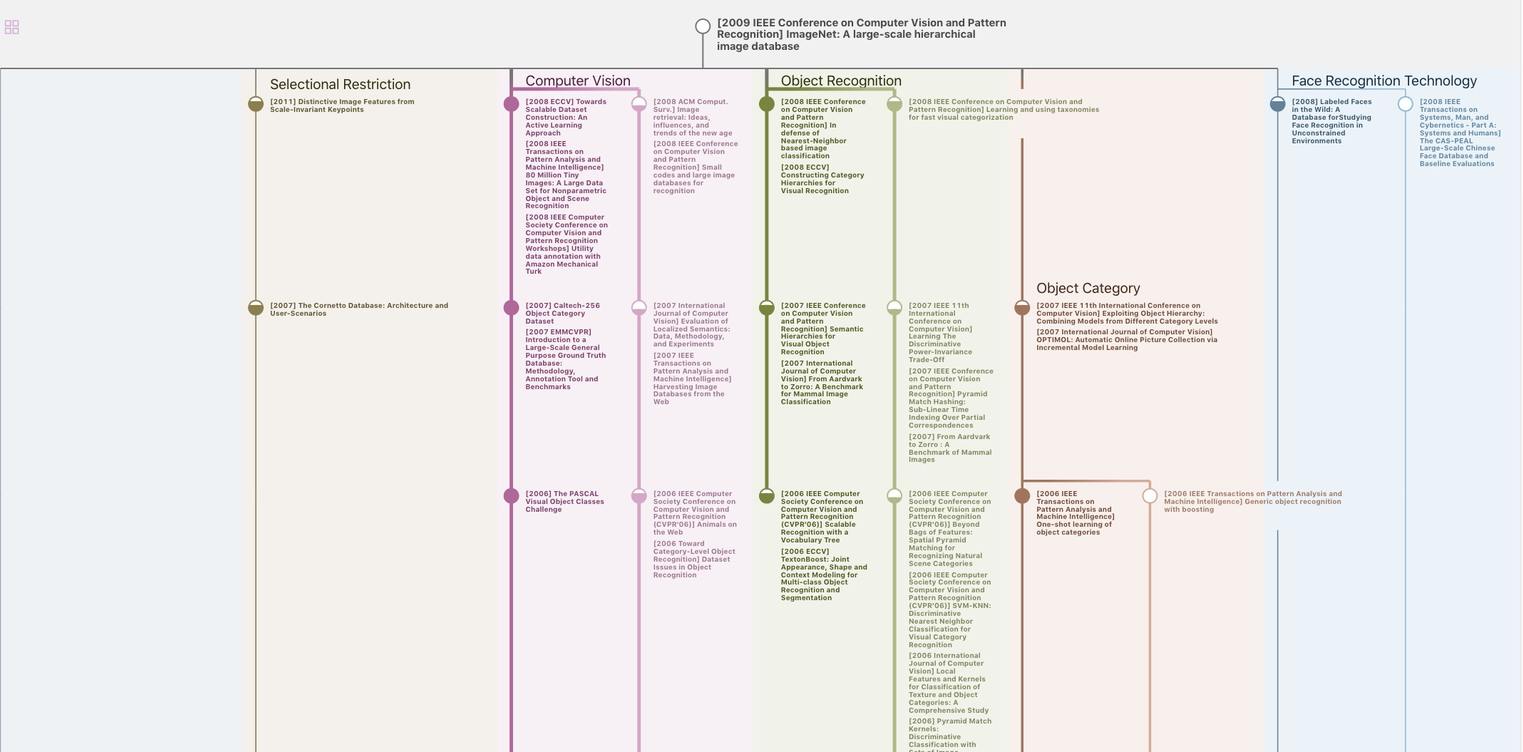
生成溯源树,研究论文发展脉络
Chat Paper
正在生成论文摘要