Joint Sparse Recovery Using Deep Unfolding With Application To Massive Random Access
2020 IEEE INTERNATIONAL CONFERENCE ON ACOUSTICS, SPEECH, AND SIGNAL PROCESSING(2020)
摘要
We propose a learning-based joint sparse recovery method for the multiple measurement vector (MMV) problem using deep unfolding. We unfold an iterative alternating direction method of multipliers (ADM) algorithm for MMV joint sparse recovery algorithm into a trainable deep network. This ADM algorithm is first obtained by modifying the squared error penalty function of an existing ADM algorithm to a back-projected squared error penalty function. Numerical results for a massive random access system show that our proposed modification to the MMV-ADM method and deep unfolding provide significant improvement in convergence and estimation performance.
更多查看译文
关键词
squared error penalty function,massive random access system,MMV-ADM method,deep unfolding,learning-based joint sparse recovery method,multiple measurement vector problem,iterative alternating direction method,multipliers algorithm,MMV joint sparse recovery algorithm,trainable deep network,ADM algorithm
AI 理解论文
溯源树
样例
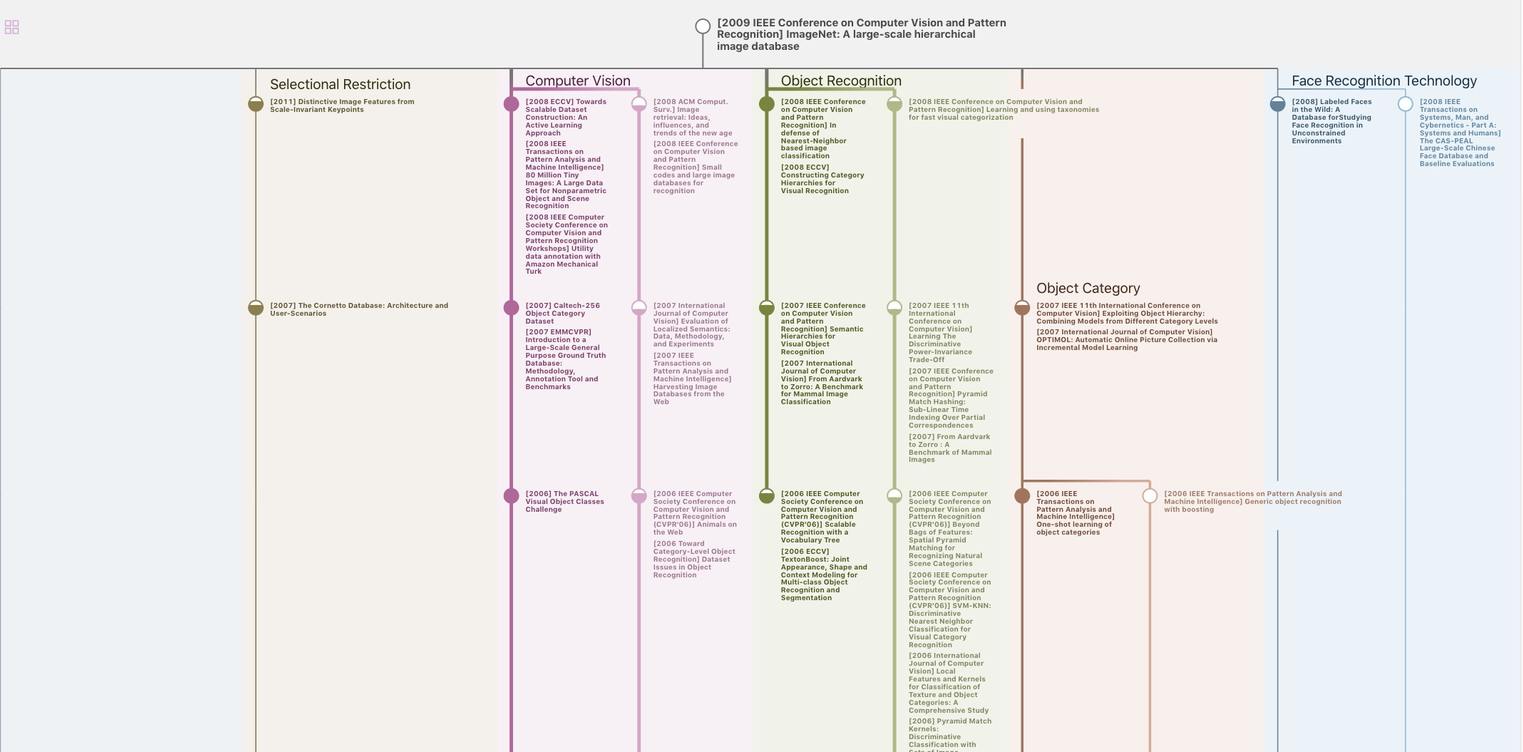
生成溯源树,研究论文发展脉络
Chat Paper
正在生成论文摘要