Fully-Neural Approach To Heavy Vehicle Detection On Bridges Using A Single Strain Sensor
2020 IEEE INTERNATIONAL CONFERENCE ON ACOUSTICS, SPEECH, AND SIGNAL PROCESSING(2020)
摘要
Bridge weigh-in-motion (BWIM) is a technique for detecting heavy vehicles that may cause serious damage to real bridges. BWIM is realized by analyzing the strain signals observed at places on the bridge in terms of bridge-component responses to the axle loads. In current practice, a BWIM system requires multiple strain sensors to collect vehicle properties including speed and axle positions for accurate load estimation, which may limit the system's life-span. Furthermore, BWIM should consider a wide variety of waveforms, which may be caused by vehicle acceleration and/or the various traveling positions in lanes. In this paper, we propose a novel BWIM mechanism, which employs a deep convolutional neural network (CNN). The CNN is able to learn actual traffic conditions and achieve accurate load estimation by using only a single strain sensor. The training dataset is collected from a distant load meter, by consulting traffic surveillance cameras and identifying similar vehicles. After the system initialization, the CNN requires no additional sensors (or cameras) for axle detection, which may reduce the costs of both installation and system maintenance.
更多查看译文
关键词
Neural network, bridge weigh-in-motion, structural health monitoring, strain signal, single sensor
AI 理解论文
溯源树
样例
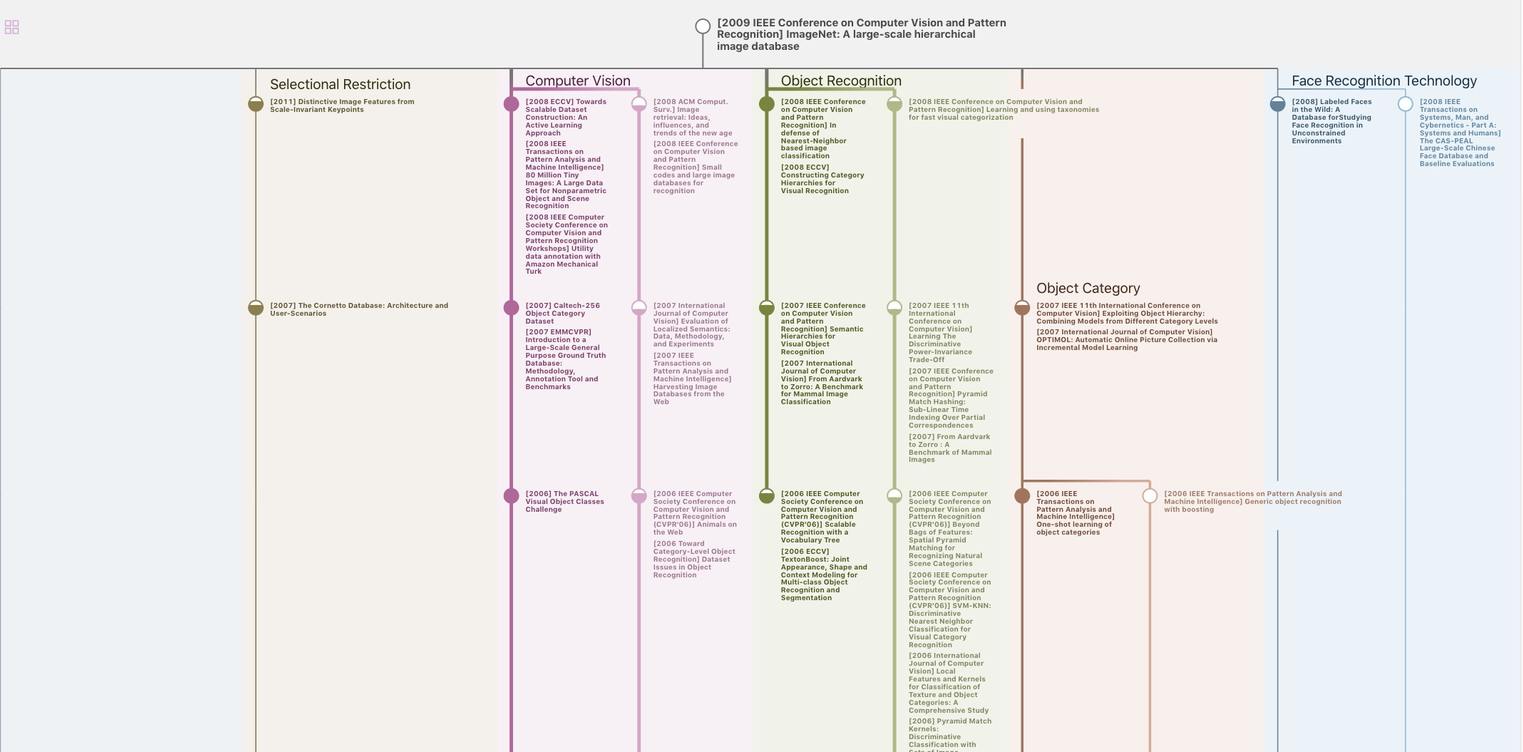
生成溯源树,研究论文发展脉络
Chat Paper
正在生成论文摘要