Eeg Feature Selection Using Orthogonal Regression: Application to Emotion Recognition
2020 IEEE INTERNATIONAL CONFERENCE ON ACOUSTICS, SPEECH, AND SIGNAL PROCESSING(2020)
摘要
A common drawback of the EEG applications is that the volume conduction of human head leads to lots of redundant information in EEG recordings. To reduce the redundancy and choose informative EEG features, in this paper, we propose an EEG feature selection technique, termed as Feature Selection with Orthogonal Regression (FSOR). Compared with classical feature selection methods, for nonlinear and nonstationary EEG signals, FSOR can employ orthogonal regression to preserve more discriminative information in the subspace. To verify the EEG feature selection performance, we collected a multichannel EEG dataset for emotion recognition and compared FSOR with two popular feature selection methods. The experimental results demonstrate the advantage of FSOR method over others for reducing the redundant information among the EEG relevant features. Additionally, we found that the absolute power ratio of beta wave to theta wave is the most discriminative feature, and beta band is the critical band for emotion recognition.
更多查看译文
关键词
EEG feature selection, discriminative feature, redundant information, orthogonal regression, embedded approaches
AI 理解论文
溯源树
样例
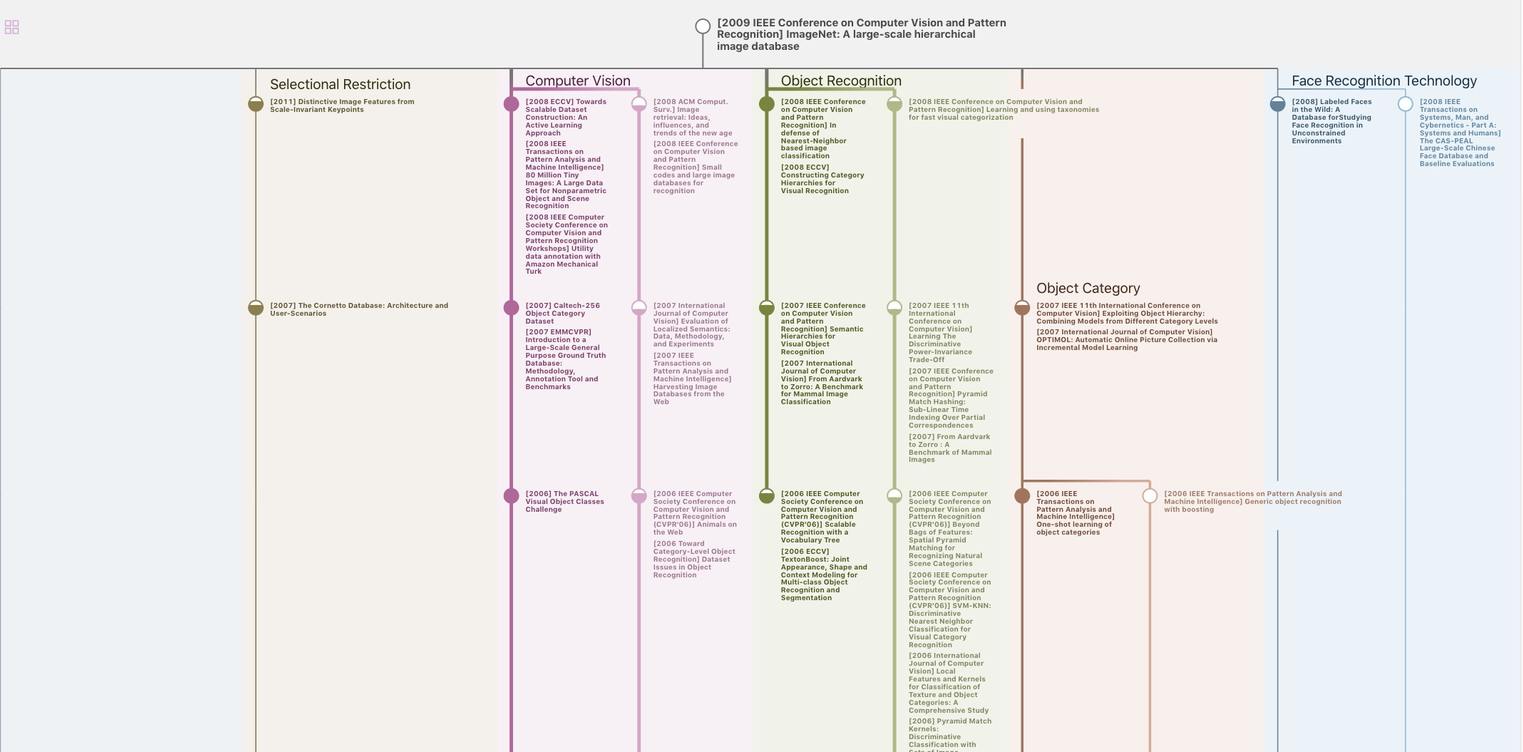
生成溯源树,研究论文发展脉络
Chat Paper
正在生成论文摘要