Sampling bias mitigation for species occurrence modeling using machine learning methods
Ecological Informatics(2020)
摘要
The identification and mitigation of sampling biases is commonly overseen in species distribution modeling, even though bias can seriously compromise the validity of modeling outcomes. Here we propose methods to 1) detect and mitigate spatial and detection sampling biases in the use of machine learning methods for modeling species occurrence and 2) assess the magnitude of bias and the effectiveness of bias mitigation on modeling prediction, variable importance, and model performance. We illustrate these techniques through the calibration of boosted decision trees for the prediction of annual occurrences of Aedes albopictus, an invasive disease vector, in South-East Pennsylvania between 2001 and 2015. Methods consist of the application of spatial filters and the assignment of sampling reliability weights to observed locations. We tested the performance of spatial bias mitigation by comparing the frequency distribution obtained for predictors before and after filtering with the distribution that would be obtained under an ideal sampling design. We also tested the performance of detection bias mitigation by comparing the importance of variables representing detection bias before and after the assignment of reliability weights. Results show that spatial filtering reduced differences between the frequency distribution obtained with the unfiltered data and the distribution that would be obtained under a reference sampling design. The assignment of sampling reliability weights to observations reduced the relative influence of detection bias on fitted models. The mitigation of spatial bias had a larger effect on modeling prediction and accuracy estimates compared to detection bias mitigation. Spatial sampling bias mitigation largely tended to reduce the number of years of predicted A. albopictus occurrence while detection bias mitigation tended to increase it. Our results highlight the importance of identifying, quantifying and mitigating observation biases as a standard practice in the use of machine learning methods for species occurrence modeling because biases can compromise the reliability of modeling outcomes and interpretation.
更多查看译文
关键词
Species occupancy,Imperfect detection,Geographic filtering,Spatial and detection sampling bias,Boosted regression trees,Species distribution modeling,Aedes albopictus
AI 理解论文
溯源树
样例
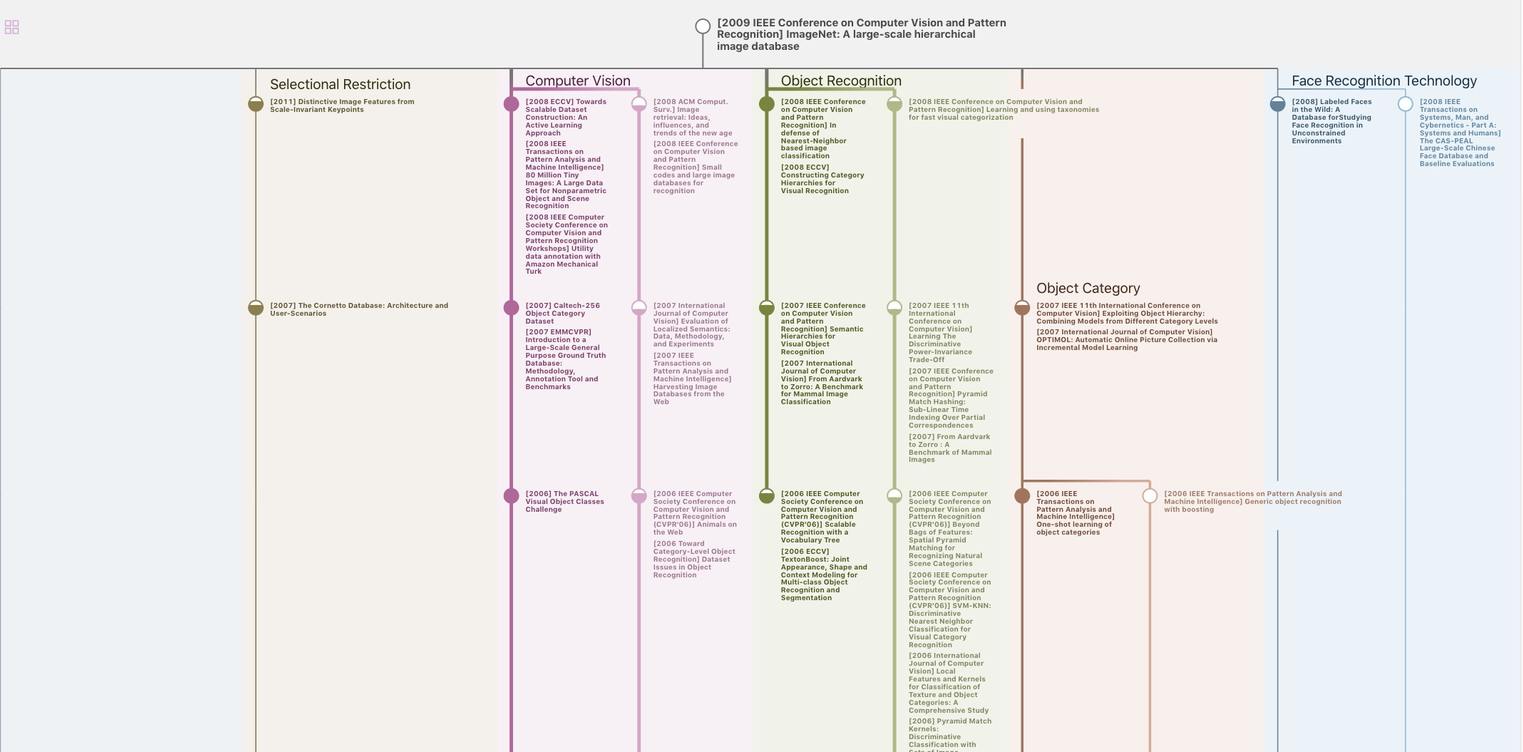
生成溯源树,研究论文发展脉络
Chat Paper
正在生成论文摘要