Spatial Gating Strategies For Graph Recurrent Neural Networks
2020 IEEE INTERNATIONAL CONFERENCE ON ACOUSTICS, SPEECH, AND SIGNAL PROCESSING(2020)
摘要
Graph Recurrent Neural Networks (GRNNs) are a neural network architecture devised to learn from graph processes, which are time sequences of graph signals. Similarly to traditional recurrent neural networks, GRNNs experience the problem of vanishing/exploding gradients when learning long term causal dependencies. When these dependencies do not depend on the graph, this issue is solved by the addition of time gates (long short-term memory architectures). However, in graph processes long term dependencies are directly influenced by the graph structure, and can be stronger or weaker across certain node paths. To address this, we propose two spatial gating strategies for GRNNs leveraging the node and edge structure of the graph. Node and edge-gated GRNNs are shown to outperform other GRNNs in a synthetic as well as a real-world problem of earthquake epicenter prediction.
更多查看译文
关键词
gating, graph recurrent neural networks, graph convolutions, graph signal processing, graph neural networks
AI 理解论文
溯源树
样例
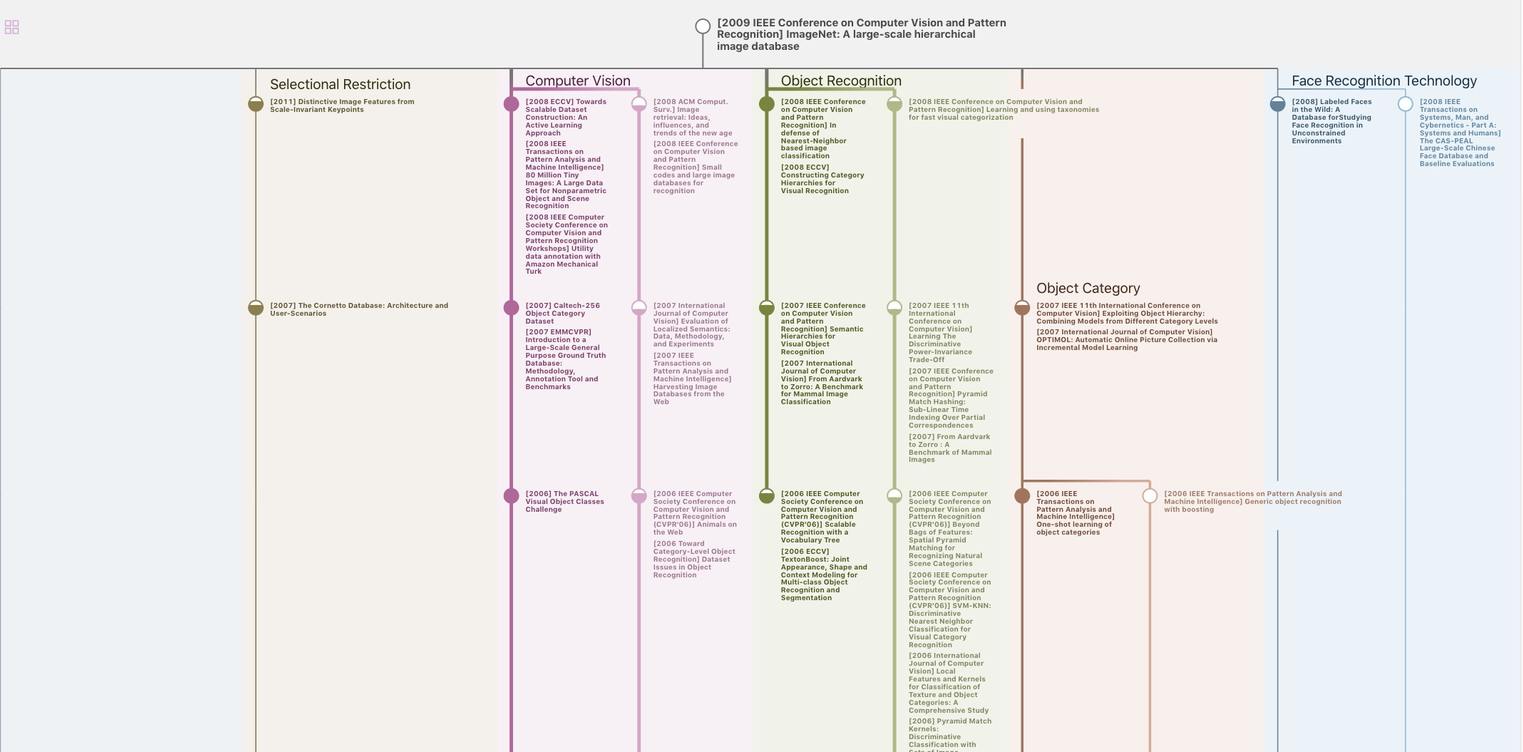
生成溯源树,研究论文发展脉络
Chat Paper
正在生成论文摘要