On Network Science and Mutual Information for Explaining Deep Neural Networks
ICASSP(2020)
摘要
In this paper, we present a new approach to interpret deep learning models. By coupling mutual information with network science, we explore how information flows through feedforward networks. We show that efficiently approximating mutual information allows us to create an information measure that quantifies how much information flows between any two neurons of a deep learning model. To that end, we propose NIF, Neural Information Flow, a technique for codifying information flow that exposes deep learning model internals and provides feature attributions.
更多查看译文
关键词
deep learning, information theory, network science, interpretability
AI 理解论文
溯源树
样例
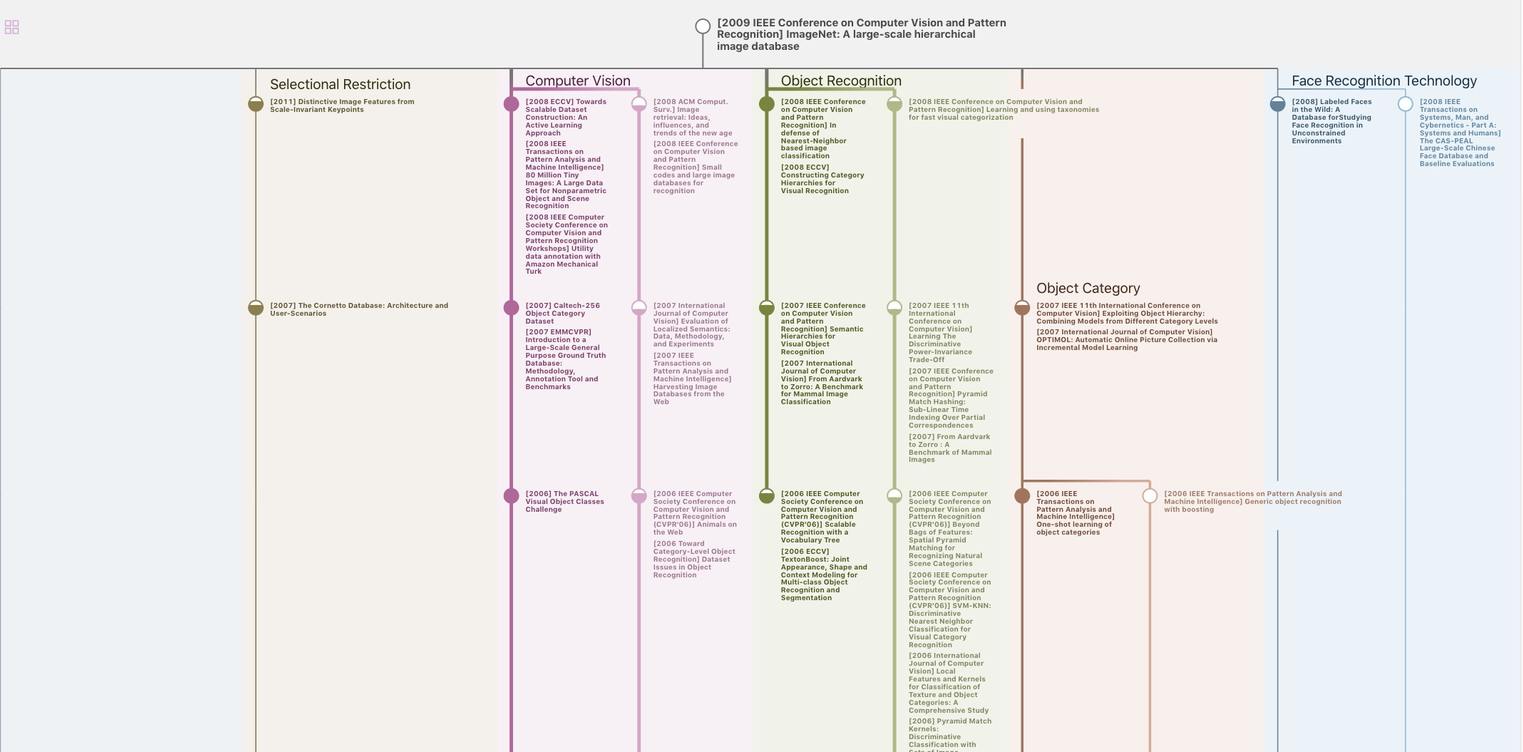
生成溯源树,研究论文发展脉络
Chat Paper
正在生成论文摘要