Augmented Adversarial Training for Cross-Modal Retrieval
IEEE Transactions on Multimedia(2021)
摘要
Cross-modal retrieval has received considerable attention in recent years. The core of cross-modal retrieval is to find a representation space to align data from different modalities according to their semantics. In this paper, we propose a cross-modal retrieval method that aligns data from different modalities by transferring one source modality to another target modality with augmented adversarial training. To preserve the semantic meaning in the modality transfer process, we employ the idea of conditional GANs and augment it. The key idea is to incorporate semantic information from the label space into the adversarial training process by sampling more semantic relevant and irrelevant source-target sample pairs. The augmented sample pairs improve the alignment from two aspects. First, relevant source-target sample pairs provide more training samples, leading to a better guidance of the alignment of fake targets and true paired targets. Second, relevant and irrelevant source-target sample pairs teach the discriminator to better distinguish true relevant pairs from fake relevant pairs, which guides the generator to better transfer from the source modality to the target modality. Extensive experiments compared with state-of-the-art methods show the promising power of our approach.
更多查看译文
关键词
Cross-modal retrieval,data alignment,adversa-rial training
AI 理解论文
溯源树
样例
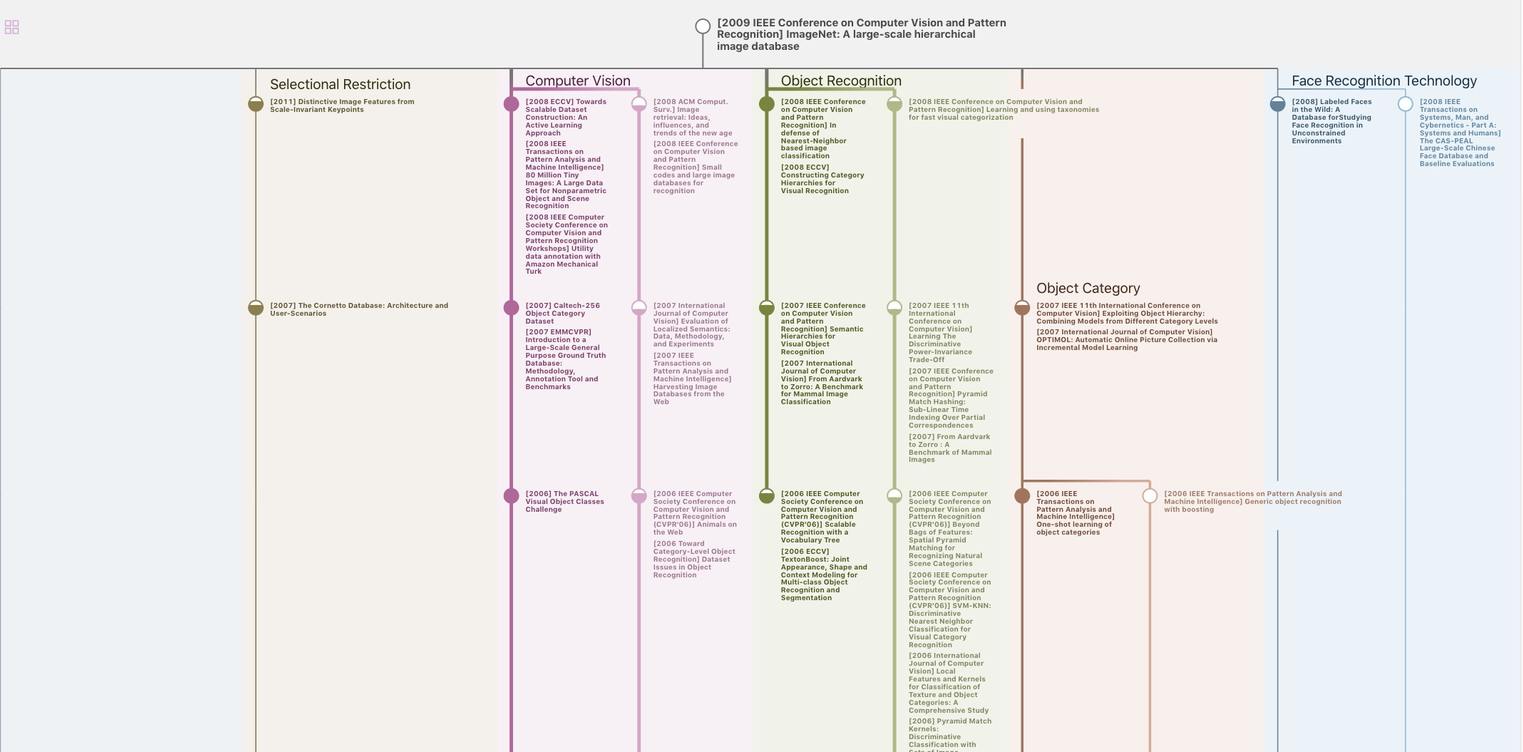
生成溯源树,研究论文发展脉络
Chat Paper
正在生成论文摘要