End-End Speech-To-Text Translation With Modality Agnostic Meta-Learning
2020 IEEE INTERNATIONAL CONFERENCE ON ACOUSTICS, SPEECH, AND SIGNAL PROCESSING(2020)
摘要
Collecting large amounts of data to train end-to-end Speech Translation (ST) models is more difficult compared to the ASR and MT tasks. Previous studies have proposed the use of transfer learning approaches to overcome the above difficulty. These approaches benefit from weakly supervised training data, such as ASR speech-to-transcript or MT text-to-text translation pairs. However, the parameters in these models are updated independently of each task, which may lead to sub-optimal solutions. In this work, we adopt a meta-learning algorithm to train a modality agnostic multi-task model that transfers knowledge from source tasks=ASR+MT to target task=ST where the ST task severely lacks data. In the meta-learning phase, parameters are updated in such a way that they act as a good initialization point for the target ST task. We evaluate the proposed meta-learning approach for ST tasks on English-German (En-De) and English-French (En-Fr) language pairs from the Multilingual Speech Translation Corpus (MuST-C). Our method outperforms the previous transfer learning approaches and sets new state-of-the-art results for En-De and En-Fr ST tasks by obtaining 9.18, and 11.76 BLEU point improvements, respectively.
更多查看译文
关键词
Speech-text translation, deep learning, meta-learning
AI 理解论文
溯源树
样例
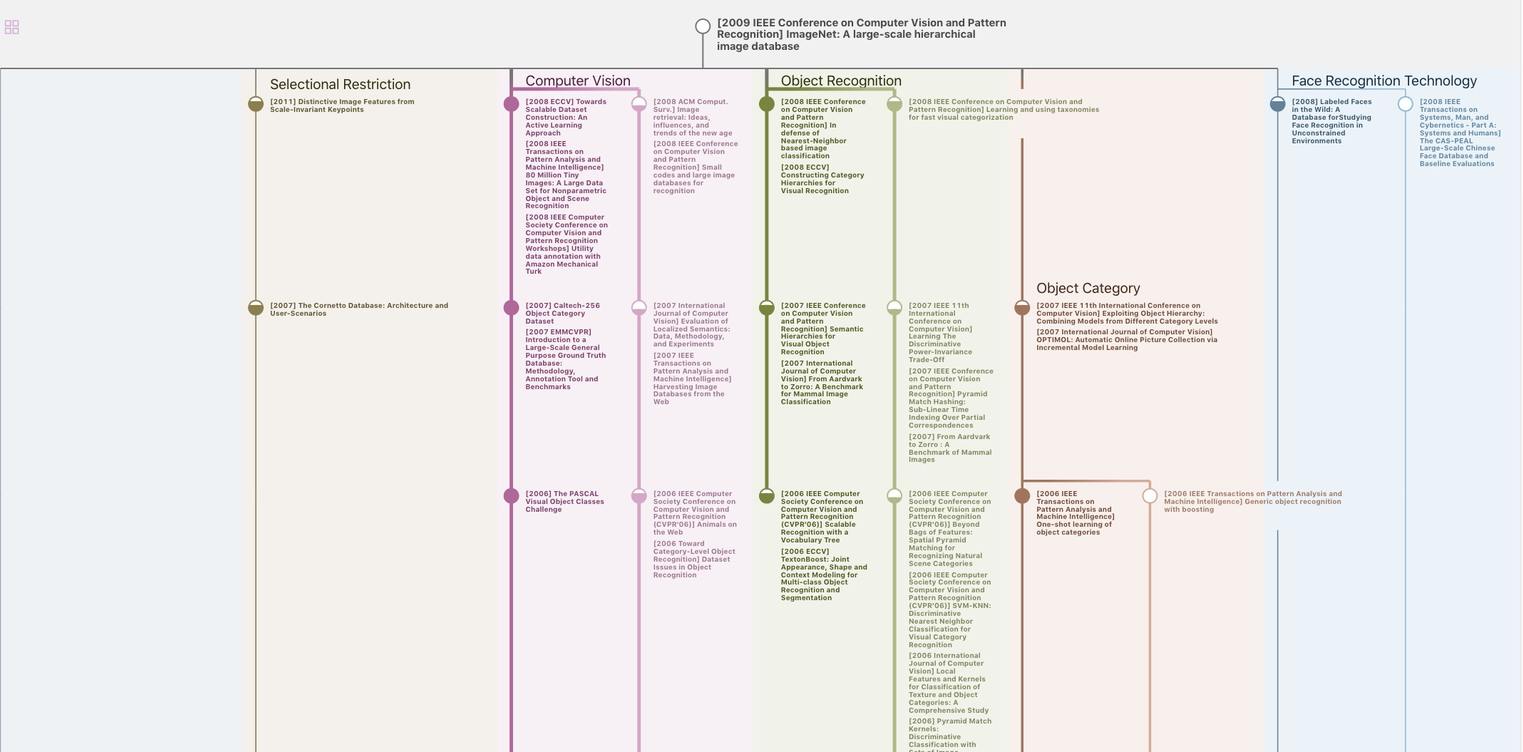
生成溯源树,研究论文发展脉络
Chat Paper
正在生成论文摘要