Blind Ct Image Quality Assessment Via Deep Learning Framework
2019 IEEE NUCLEAR SCIENCE SYMPOSIUM AND MEDICAL IMAGING CONFERENCE (NSS/MIC)(2019)
摘要
Computed tomography (CT) images will be severely damaged from low-mAs acquisition conditions. Seriously degraded CT images may lead to diagnostic bias in clinics. It is vital to assess CT image quality before diagnosis. However, lack of high-quality reference CT images in clinical practice makes the full-reference (FR)- and reduced-reference (RR)- image quality assessment (IQA) models difficult for CT image quality assessment. In addition, convolutional neural network (CNN) has been proven effective for natural image quality assessment. Meanwhile these CNN-based methods require a large amount of manually labeled data for network training to get satisfactory results. It should be noted that the pre-collected dataset is limited and manual labeling is time-consuming and labor-intensive. In this work, we develop a new no-reference (NR)-IQA strategy in a deep learning framework to assess CT image quality effectively. Specifically, at first, we use the FR-IQA metric, i.e., PSNR and SSIM, to comprehensively assess CT image quality objectively which is served as label for NR-IOA network. The PSNR and SSIM measure the noise level and structural distortion in the CT images which are the two most vital factors affecting diagnosis. Then, we employ the labeled data to train a convolutional neural network, making it capable of providing quality measurements for each input image without reference image. The experimental results with Mayo dataset demonstrate that the present NR-IQA network can accurately predict CT image quality.
更多查看译文
关键词
deep learning,quality
AI 理解论文
溯源树
样例
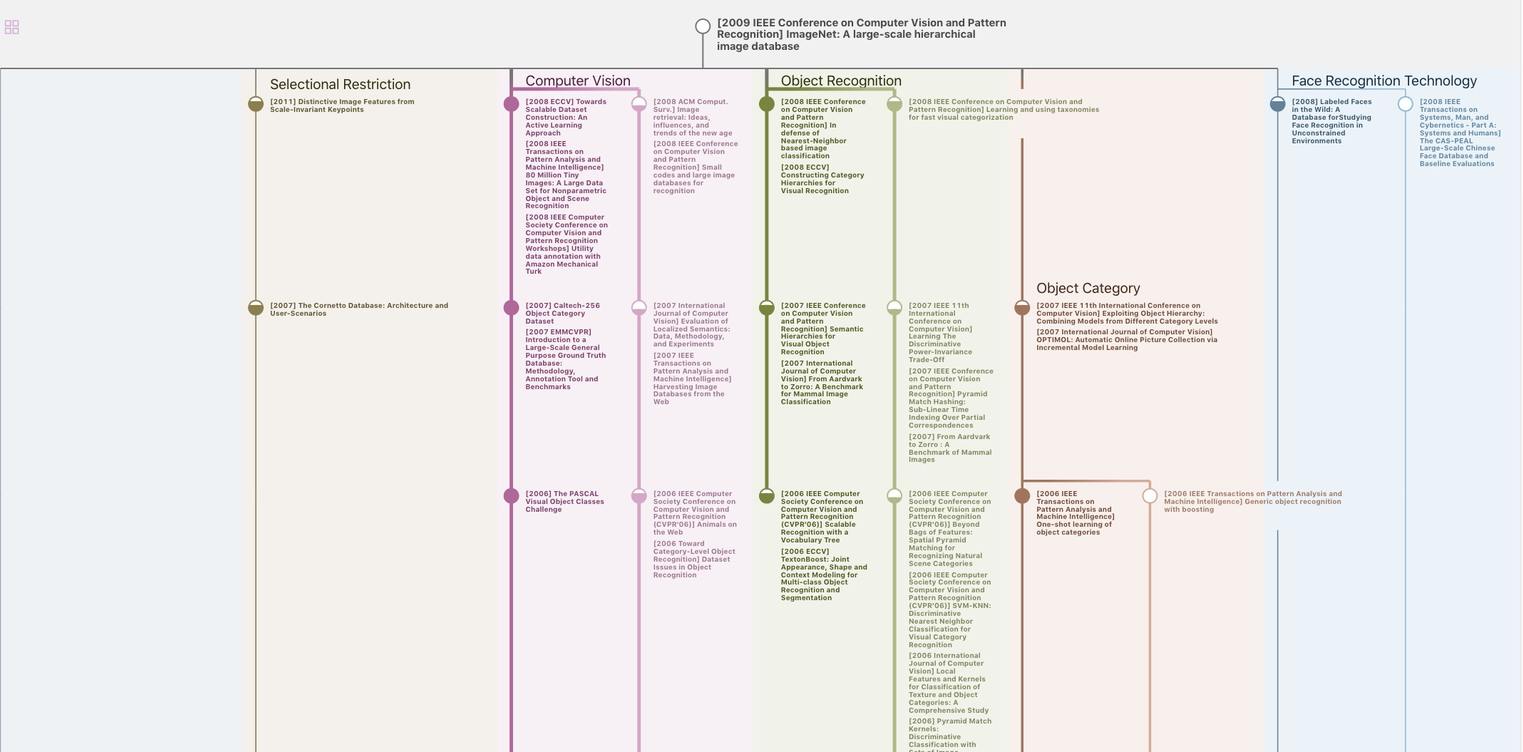
生成溯源树,研究论文发展脉络
Chat Paper
正在生成论文摘要