Revisiting Fast Spectral Clustering with Anchor Graph
ICASSP(2020)
摘要
Many anchor-graph-based spectral clustering methods have been proposed to accelerate spectral clustering for large scale problems. In this paper, we revisit the popular large-scale spectral clustering method based on the anchor graph which is equivalent to the spectral decomposition on a similar matrix obtained using a second-order transition probability. However, due to the special structure of the bipartite graph, there is no stable distribution of the random walk process. The even-order transition probabilities may only a side view of the bipartite structure, resulting in breaking the independence of data points and leading to undesired artifacts for boundary samples. Therefore, we propose a Fast Spectral Clustering based on the Random Walk Laplacian (FRWL) method. The random walk Laplacian balances explicitly the popularity of anchors and the independence of data points, which keeps the structure of boundary samples. The experimental results demonstrate the efficiency and effectiveness of our method.
更多查看译文
关键词
Spectral Clustering, large scale clustering, anchor-based graph, random walk Laplacian
AI 理解论文
溯源树
样例
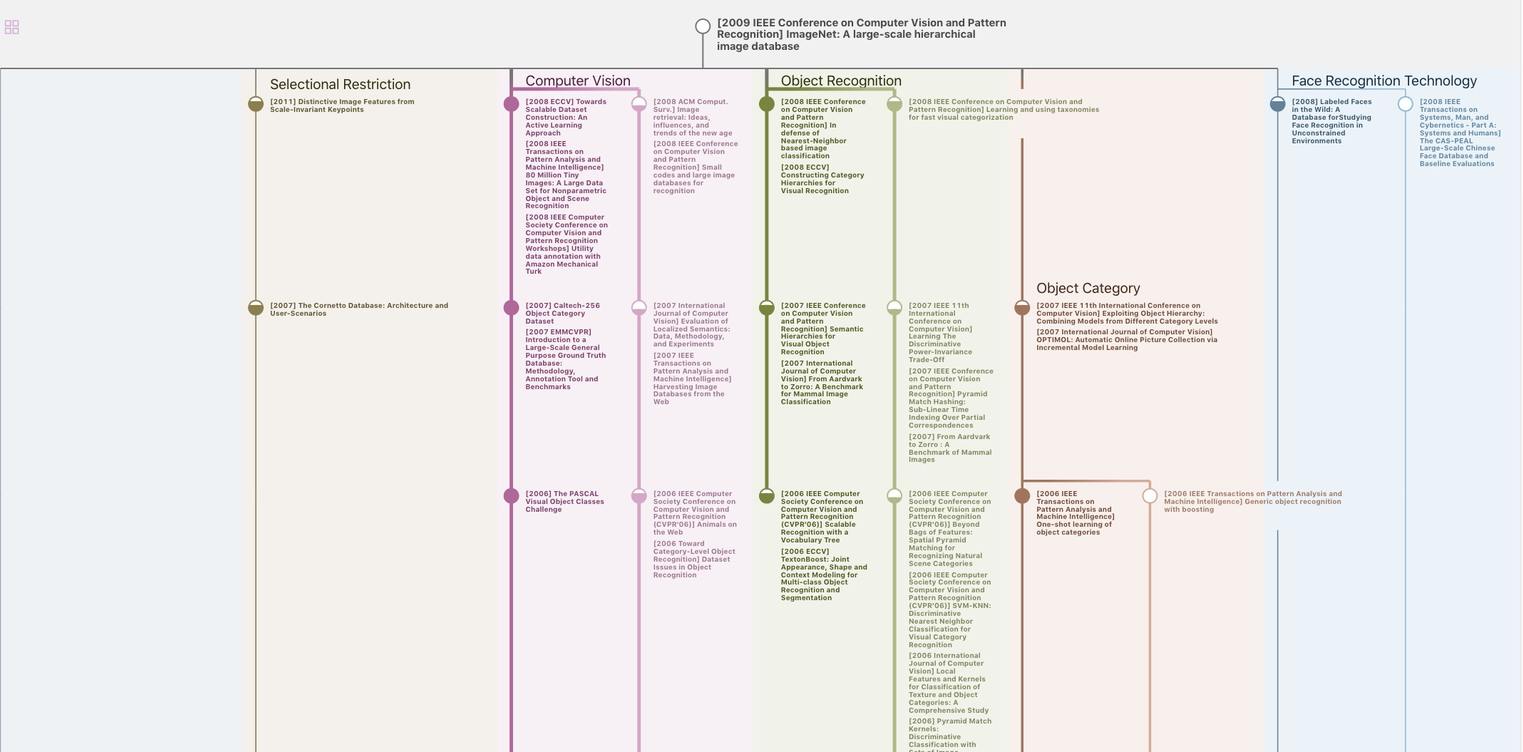
生成溯源树,研究论文发展脉络
Chat Paper
正在生成论文摘要