Variational Autoencoder-Based Dimensionality Reduction For High-Dimensional Small-Sample Data Classification
INTERNATIONAL JOURNAL OF COMPUTATIONAL INTELLIGENCE AND APPLICATIONS(2020)
摘要
Classification problems in which the number of features (dimensions) is unduly higher than the number of samples (observations) is an essential research and application area in a variety of domains, especially in computational biology. It is also known as a high-dimensional small-sample-size (HDSSS) problem. Various dimensionality reduction methods have been developed, but they are not potent with the small-sample-sized high-dimensional datasets and suffer from overfitting and high-variance gradients. To overcome the pitfalls of sample size and dimensionality, this study employed variational autoencoder (VAE), which is a dynamic framework for unsupervised learning in recent years. The objective of this study is to investigate a reliable classification model for high-dimensional and small-sample-sized datasets with minimal error. Moreover, it evaluated the strength of different architectures of VAE on the HDSSS datasets. In the experiment, six genomic microarray datasets from Kent Ridge Biomedical Dataset Repository were selected, and several choices of dimensions (features) were applied for data preprocessing. Also, to evaluate the classification accuracy and to find a stable and suitable classifier, nine state-of-the-art classifiers that have been successful for classification tasks in high-dimensional data settings were selected. The experimental results demonstrate that the VAE can provide superior performance compared to traditional methods such as PCA, fastlCA, FA, NMF, and LDA in terms of accuracy and AUROC.
更多查看译文
关键词
High-dimensional and small-sample size dataset, variational autoencoder, classification, computational biology, deep learning
AI 理解论文
溯源树
样例
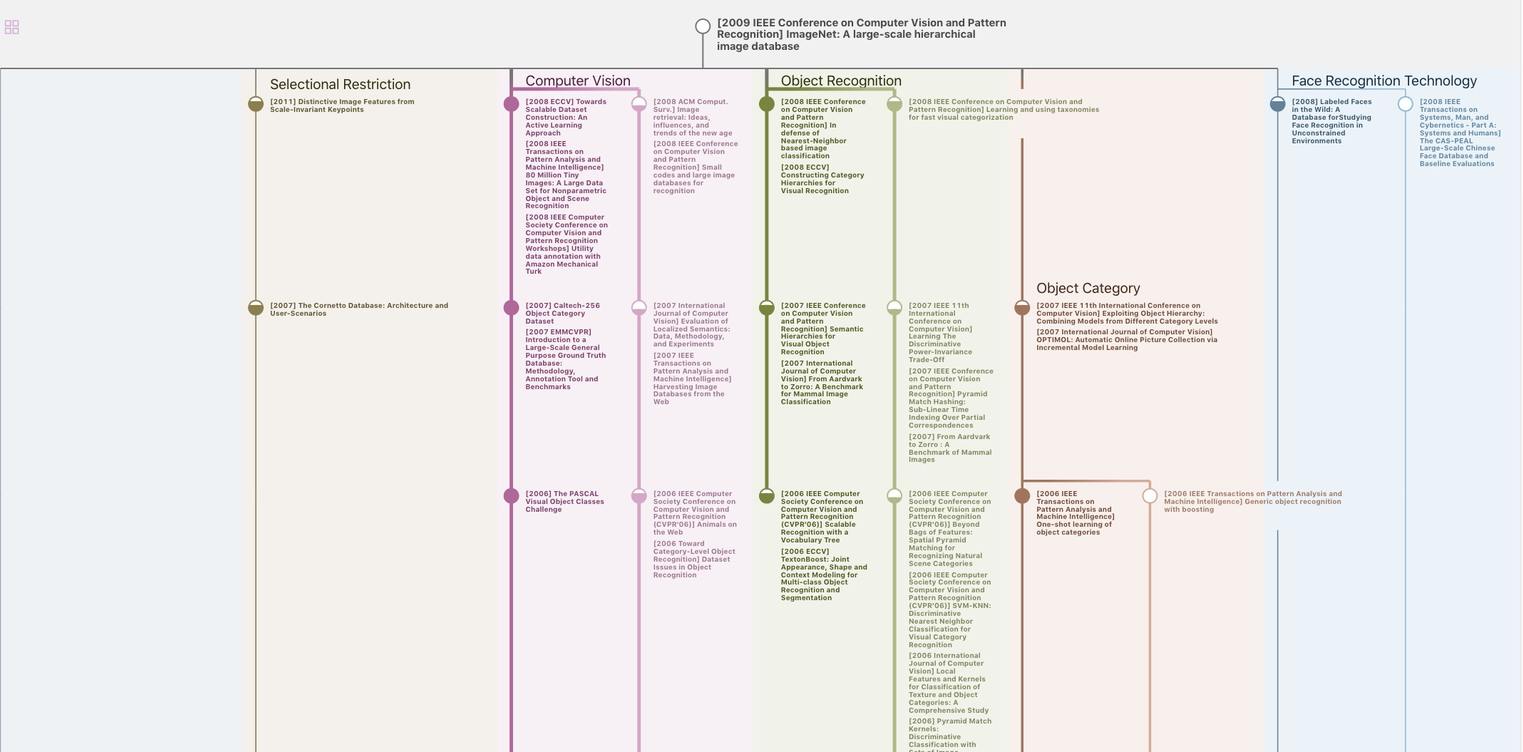
生成溯源树,研究论文发展脉络
Chat Paper
正在生成论文摘要