Machine-Learning-Based Hybrid Random-Fuzzy Uncertainty Quantification for EMC and SI Assessment
IEEE Transactions on Electromagnetic Compatibility(2020)
摘要
Modeling the effects of uncertainty is of crucial importance in the signal integrity and Electromagnetic Compatibility assessment of electronic products. In this article, a novel machine-learning-based approach for uncertainty quantification problems involving both random and epistemic variables is presented. The proposed methodology leverages evidence theory to represent probabilistic and epistemic uncertainties in a common framework. Then, Bayesian optimization is used to efficiently propagate this hybrid uncertainty on the performance of the system under study. Two suitable application examples validate the accuracy and efficiency of the proposed method.
更多查看译文
关键词
Bayesian optimization,epistemic uncertainty,random-fuzzy problems
AI 理解论文
溯源树
样例
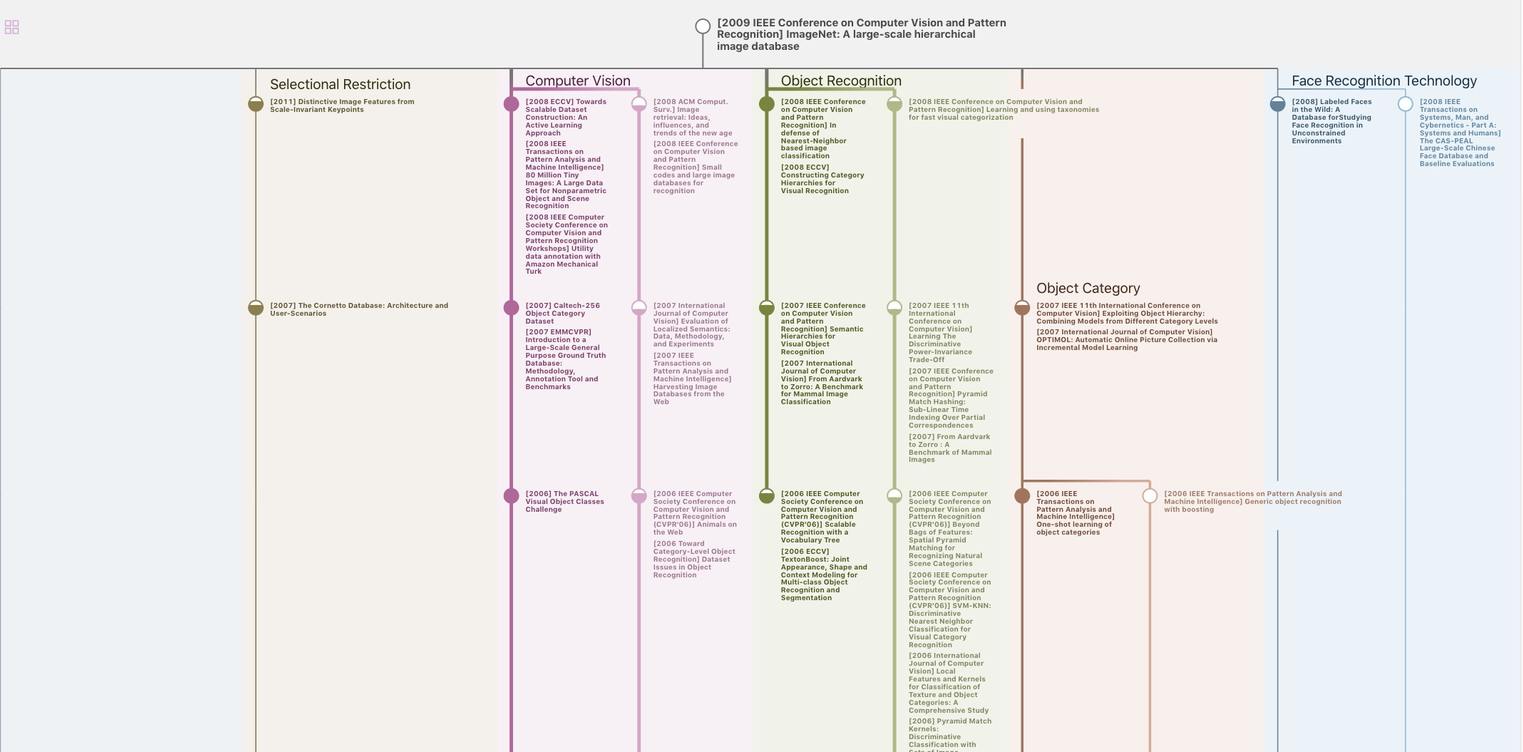
生成溯源树,研究论文发展脉络
Chat Paper
正在生成论文摘要