Enhancing The Labelling Of Audio Samples For Automatic Instrument Classification Based On Neural Networks
2020 IEEE INTERNATIONAL CONFERENCE ON ACOUSTICS, SPEECH, AND SIGNAL PROCESSING(2020)
摘要
The polyphonic OpenMIC-2018 dataset is based on weak and incomplete labels. The automatic classification of sound events, based on the VGGish bottleneck layer as proposed before by the AudioSet, implies the classification of only one second at a time, making it hard to find the label of that exact moment. To answer this question, this paper proposes PureMic, a new strongly labelled dataset (SLD) that isolates 1000 single instrument clips manually labelled. Moreover, the proposed model classifies clips over time and also enhances the labelling robustness of a high number of unlabelled samples in OpenMIC-2018 due to its ability of classification over time. In the paper we disambiguate and report the automatic labelling of previously unlabelled samples. Our proposed new labels achieves a mean average precision (mAP) of 0.701 for OpenMIC test data, outperforming its baseline (0.66). We released our code online in order to follow the proposed implementation(1).
更多查看译文
关键词
OpenMIC-2018, AudioSet, PureMic, Instrument classification, Instrument labelling, Deep learning
AI 理解论文
溯源树
样例
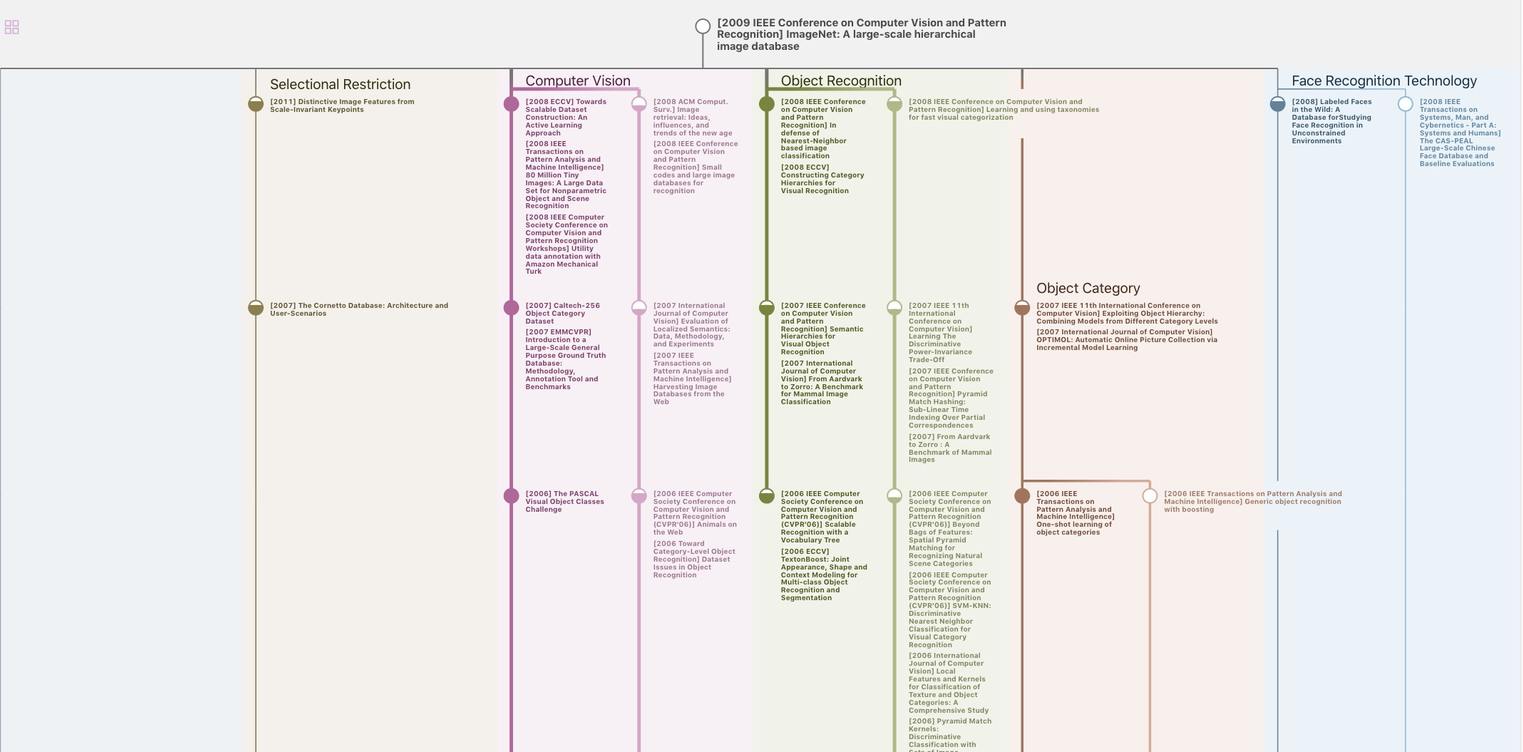
生成溯源树,研究论文发展脉络
Chat Paper
正在生成论文摘要