Infinite-Dimensional Gradient-Based Descent For Alpha-Divergence Minimisation
ANNALS OF STATISTICS(2021)
摘要
This paper introduces the (alpha, Gamma)-descent, an iterative algorithm which operates on measures and performs alpha-divergence minimisation in a Bayesian framework. This gradient-based procedure extends the commonly-used variational approximation by adding a prior on the variational parameters in the form of a measure. We prove that for a rich family of functions Gamma, this algorithm leads at each step to a systematic decrease in the alpha-divergence and derive convergence results. Our framework recovers the Entropic Mirror Descent algorithm and provides an alternative algorithm that we call the Power Descent. Moreover, in its stochastic formulation, the (alpha, Gamma)-descent allows to optimise the mixture weights of any given mixture model without any information on the underlying distribution of the variational parameters. This renders our method compatible with many choices of parameters updates and applicable to a wide range of Machine Learning tasks. We demonstrate empirically on both toy and real-world examples the benefit of using the Power Descent and going beyond the Entropic Mirror Descent framework, which fails as the dimension grows.
更多查看译文
关键词
Variational inference, alpha-divergence, Kullback-Leibler divergence, Mirror Descent
AI 理解论文
溯源树
样例
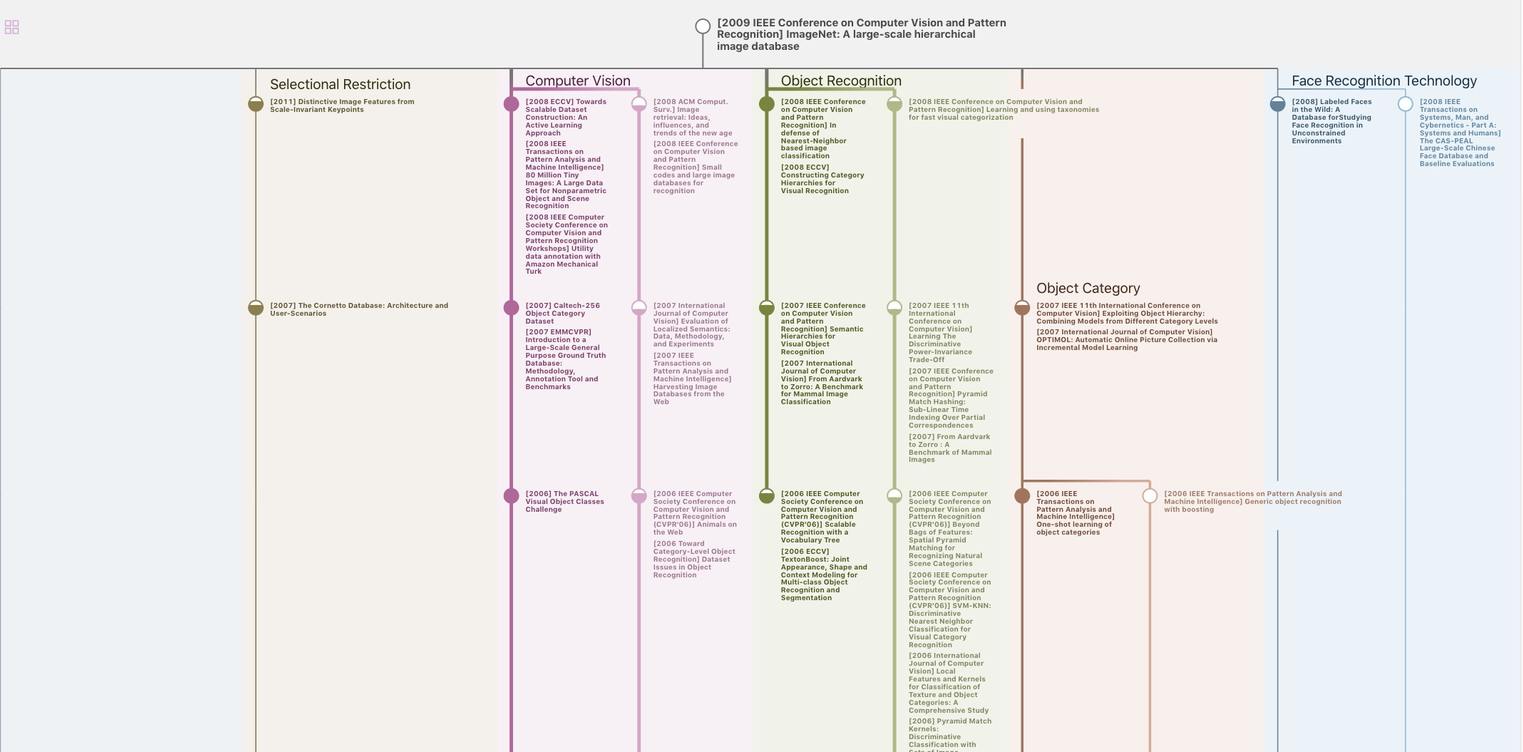
生成溯源树,研究论文发展脉络
Chat Paper
正在生成论文摘要