Why distillation helps: a statistical perspective
arxiv(2020)
摘要
Knowledge distillation is a technique for improving the performance of a simple "student" model by replacing its one-hot training labels with a distribution over labels obtained from a complex "teacher" model. While this simple approach has proven widely effective, a basic question remains unresolved: why does distillation help? In this paper, we present a statistical perspective on distillation which addresses this question, and provides a novel connection to extreme multiclass retrieval techniques. Our core observation is that the teacher seeks to estimate the underlying (Bayes) class-probability function. Building on this, we establish a fundamental bias-variance tradeoff in the student's objective: this quantifies how approximate knowledge of these class-probabilities can significantly aid learning. Finally, we show how distillation complements existing negative mining techniques for extreme multiclass retrieval, and propose a unified objective which combines these ideas.
更多查看译文
关键词
distillation
AI 理解论文
溯源树
样例
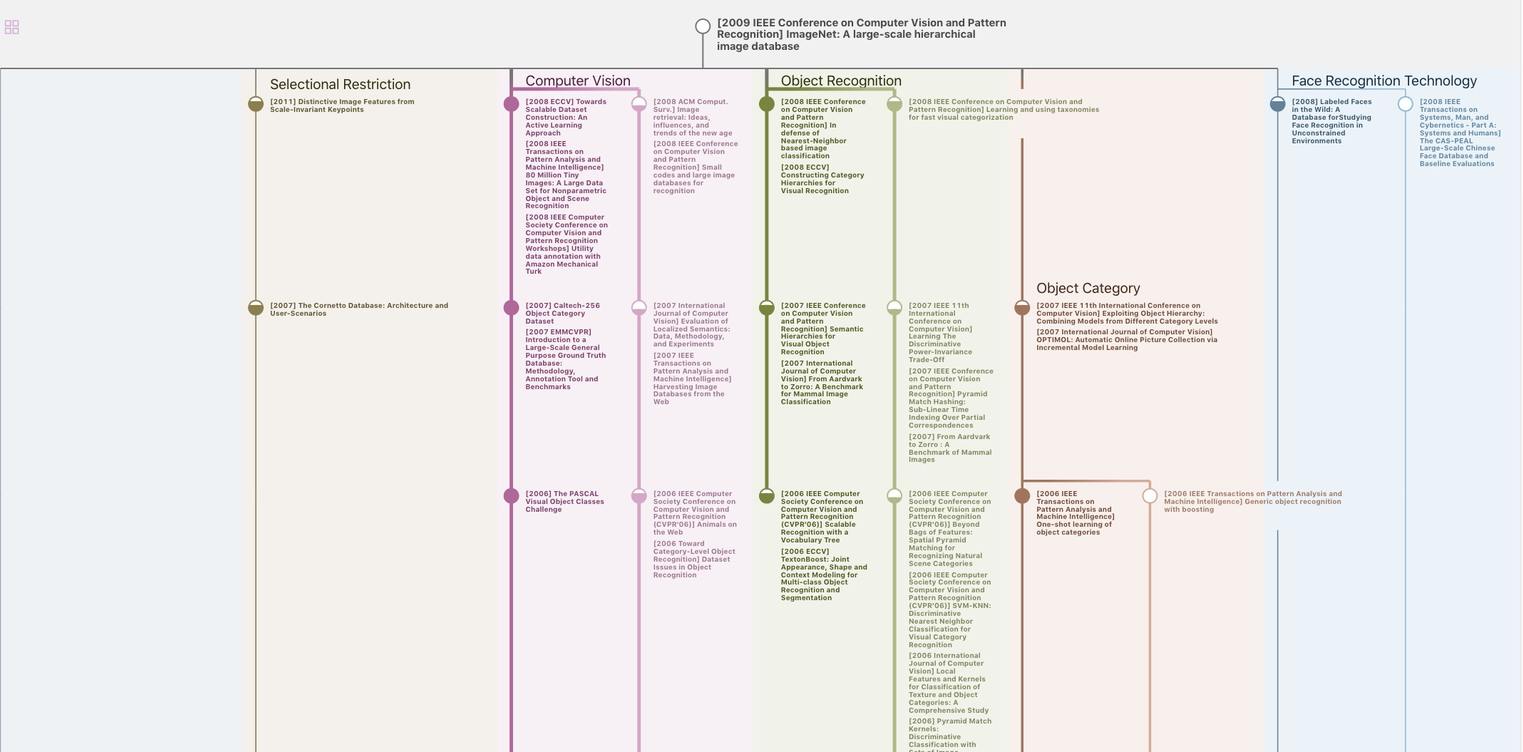
生成溯源树,研究论文发展脉络
Chat Paper
正在生成论文摘要