Sparsity-Based Audio Declipping Methods: Selected Overview, New Algorithms, and Large-Scale Evaluation
arxiv(2021)
摘要
Recent advances in audio declipping have substantially improved the state of the art. Yet, practitioners need guidelines to choose a method, and while existing benchmarks have been instrumental in advancing the field, larger-scale experiments are needed to guide such choices. First, we show that the clipping levels in existing small-scale benchmarks are moderate and call for benchmarks with more perceptually significant clipping levels. We then propose a general algorithmic framework for declipping that covers existing and new combinations of variants of state-of-the-art techniques exploiting time-frequency sparsity: synthesis vs. analysis sparsity, with plain or structured sparsity. Finally, we systematically compare these combinations and a selection of state-of-the-art methods. Using a large-scale numerical benchmark and a smaller scale formal listening test, we provide guidelines for various clipping levels, both for speech and various musical genres. The code is made publicly available for the purpose of reproducible research and benchmarking.
更多查看译文
关键词
Audio declipping,listening test,sparsity,structured sparsity,time-frequency
AI 理解论文
溯源树
样例
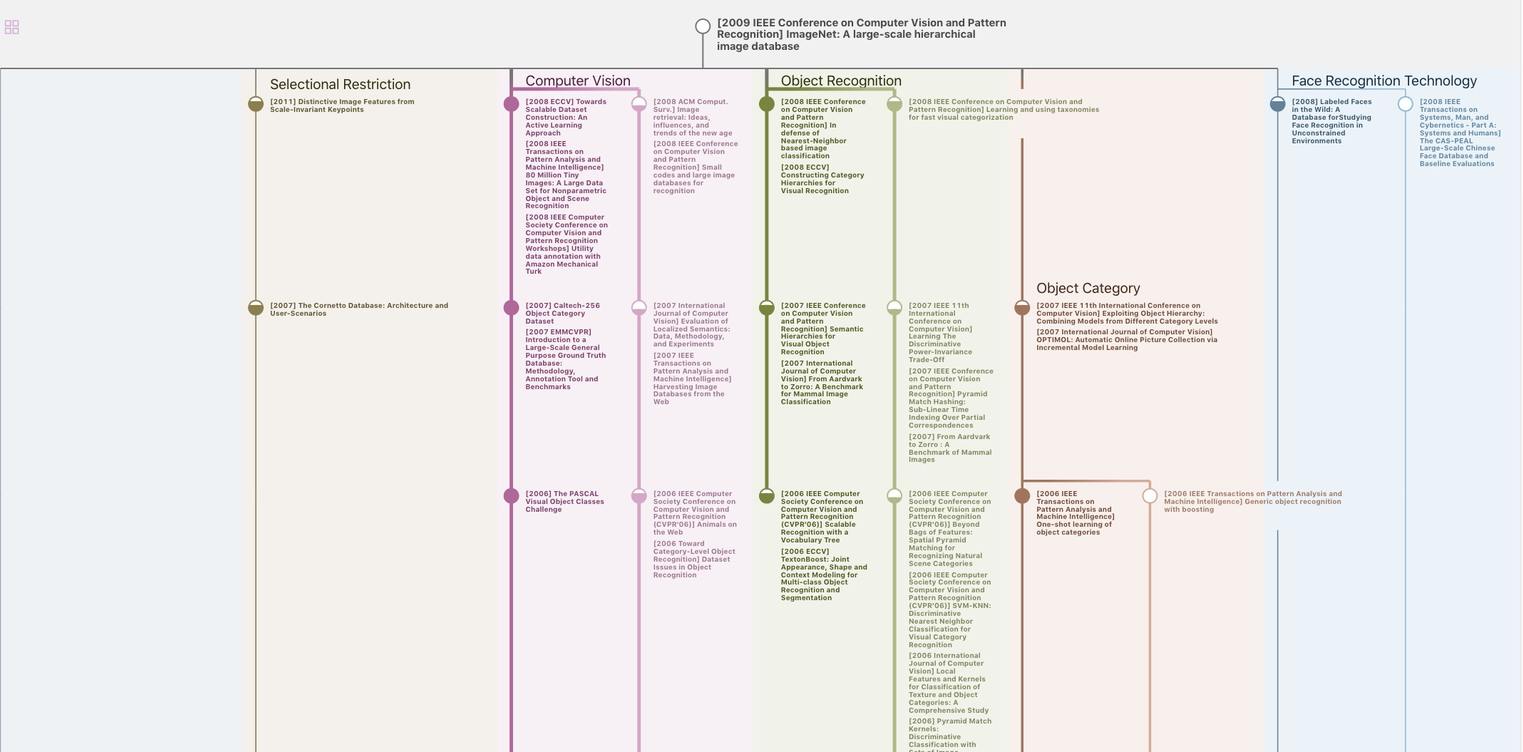
生成溯源树,研究论文发展脉络
Chat Paper
正在生成论文摘要