A machine learning route between band mapping and band structure
arxiv(2022)
摘要
The electronic band structure and crystal structure are the two complementary identifiers of solid-state materials. Although convenient instruments and reconstruction algorithms have made large, empirical, crystal structure databases possible, extracting the quasiparticle dispersion (closely related to band structure) from photoemission band mapping data is currently limited by the available computational methods. To cope with the growing size and scale of photoemission data, here we develop a pipeline including probabilistic machine learning and the associated data processing, optimization and evaluation methods for band-structure reconstruction, leveraging theoretical calculations. The pipeline reconstructs all 14 valence bands of a semiconductor and shows excellent performance on benchmarks and other materials datasets. The reconstruction uncovers previously inaccessible momentum-space structural information on both global and local scales, while realizing a path towards integration with materials science databases. Our approach illustrates the potential of combining machine learning and domain knowledge for scalable feature extraction in multidimensional data.
更多查看译文
关键词
band mapping,machine learning
AI 理解论文
溯源树
样例
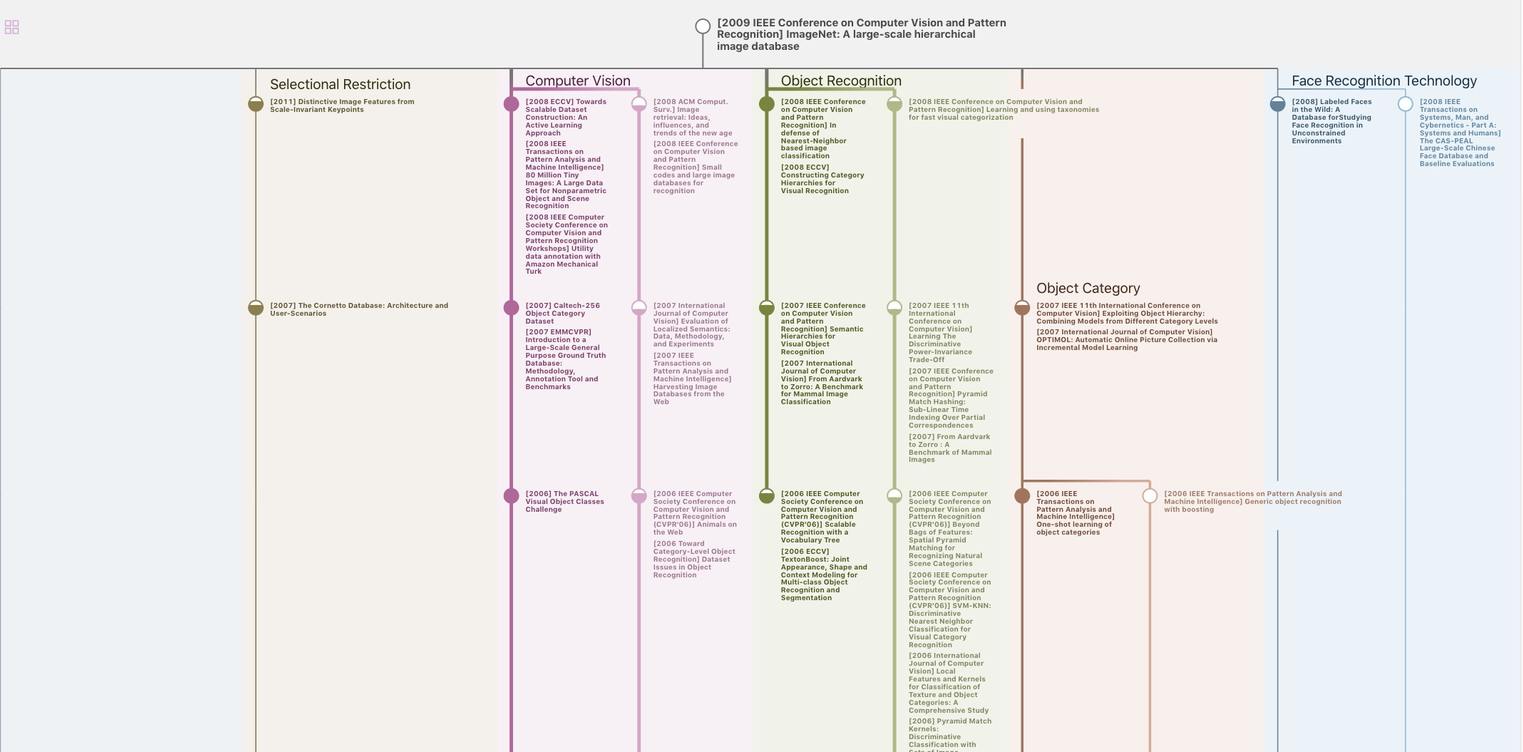
生成溯源树,研究论文发展脉络
Chat Paper
正在生成论文摘要