The Inverse G-Wishart distribution and variational message passing
AUSTRALIAN & NEW ZEALAND JOURNAL OF STATISTICS(2021)
摘要
Message passing on a factor graph is a powerful paradigm for the coding of approximate inference algorithms for arbitrarily large graphical models. The notion of a factor graph fragment allows for compartmentalisation of algebra and computer code. We show that the Inverse G-Wishart family of distributions enables fundamental variational message passing factor graph fragments to be expressed elegantly and succinctly. Such fragments arise in models for which approximate inference concerning covariance matrix or variance parameters is made, and are ubiquitous in contemporary statistics and machine learning.
更多查看译文
关键词
approximate Bayesian inference, G-Wishart distribution, mean-field variational Bayes, scalable statistical methodology
AI 理解论文
溯源树
样例
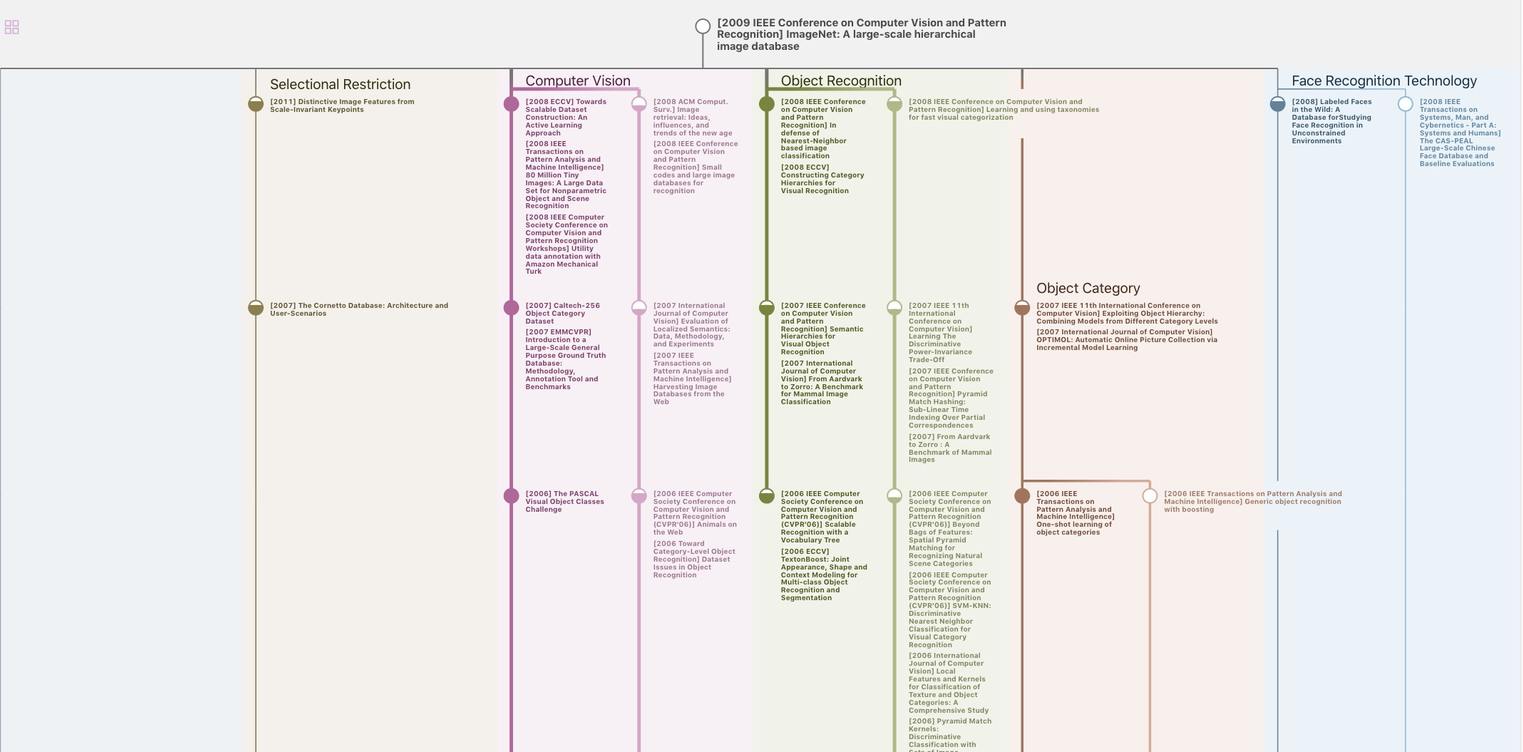
生成溯源树,研究论文发展脉络
Chat Paper
正在生成论文摘要