Benchmarking End-To-End Learning Of Mimo Physical-Layer Communication
2020 IEEE GLOBAL COMMUNICATIONS CONFERENCE (GLOBECOM)(2020)
摘要
End-to-end data-driven machine learning (ML) of multiple-input multiple-output (MIMO) systems has been shown to have the potential of exceeding the performance of engineered M IMO transceivers, without any a priori knowledge of communication-theoretic principles. In this work, we aim to understand to what extent and for which scenarios this claim holds true when comparing with fair benchmarks. We study closed-loop MIMO, open-loop MIMO, and multi-user MIMO (MU-MIMO) and show that the gains of ML-based communication in the former two cases can be to a large extent ascribed to implicitly learned geometric shaping and bit and power allocation, not to learning new spatial encoders. For MU-MIMO, we demonstrate the feasibility of a novel method with centralized learning and decentralized executing, outperforming conventional zero-forcing. For each scenario, we provide explicit descriptions as well as open-source implementations of the selected neural-network architectures.
更多查看译文
关键词
learning,end-to-end,physical-layer
AI 理解论文
溯源树
样例
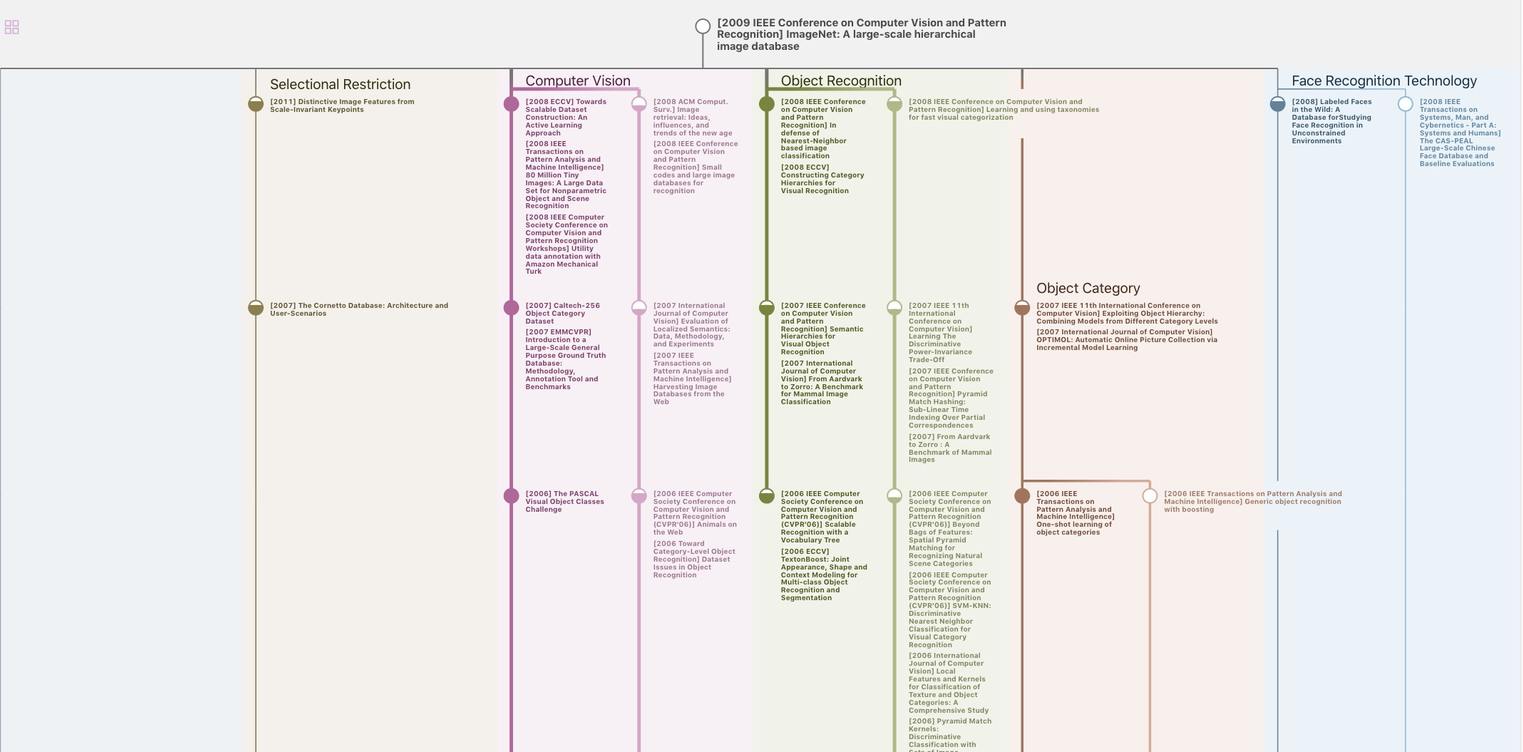
生成溯源树,研究论文发展脉络
Chat Paper
正在生成论文摘要