Few-Shot Learning of Video Action Recognition Only Based on Video Contents
2020 IEEE Winter Conference on Applications of Computer Vision (WACV)(2020)
摘要
The success of video action recognition based on Deep Neural Networks (DNNs) is highly dependent on a large number of manually labeled videos. In this paper, we introduce a supervised learning approach to recognize video actions with very few training videos. Specifically, we propose Temporal Attention Vectors (TAVs) which adapt various length videos to preserve the temporal information of the entire video. We evaluate the TAVs on UCF101 and HMDB51. Without training any deep 3D or 2D frame feature extractors on video datasets (only pre-trained on ImageNet), the TAVs only introduce 2.1M parameters but outperforms the state-of-the-art video action recognition benchmarks with very few labeled training videos (e.g. 92% on UCF101 and 59% on HMDB51, with 10 and 8 training videos per class, respectively). Furthermore, our approach can still achieve competitive results on full datasets (97.1% on UCF101 and 77% on HMDB51).
更多查看译文
关键词
UCF101,HMDB51,video datasets,TAVs,labeled training videos,shot learning,video contents,deep neural networks,supervised learning approach,video actions,temporal attention vectors,video action recognition benchmarks
AI 理解论文
溯源树
样例
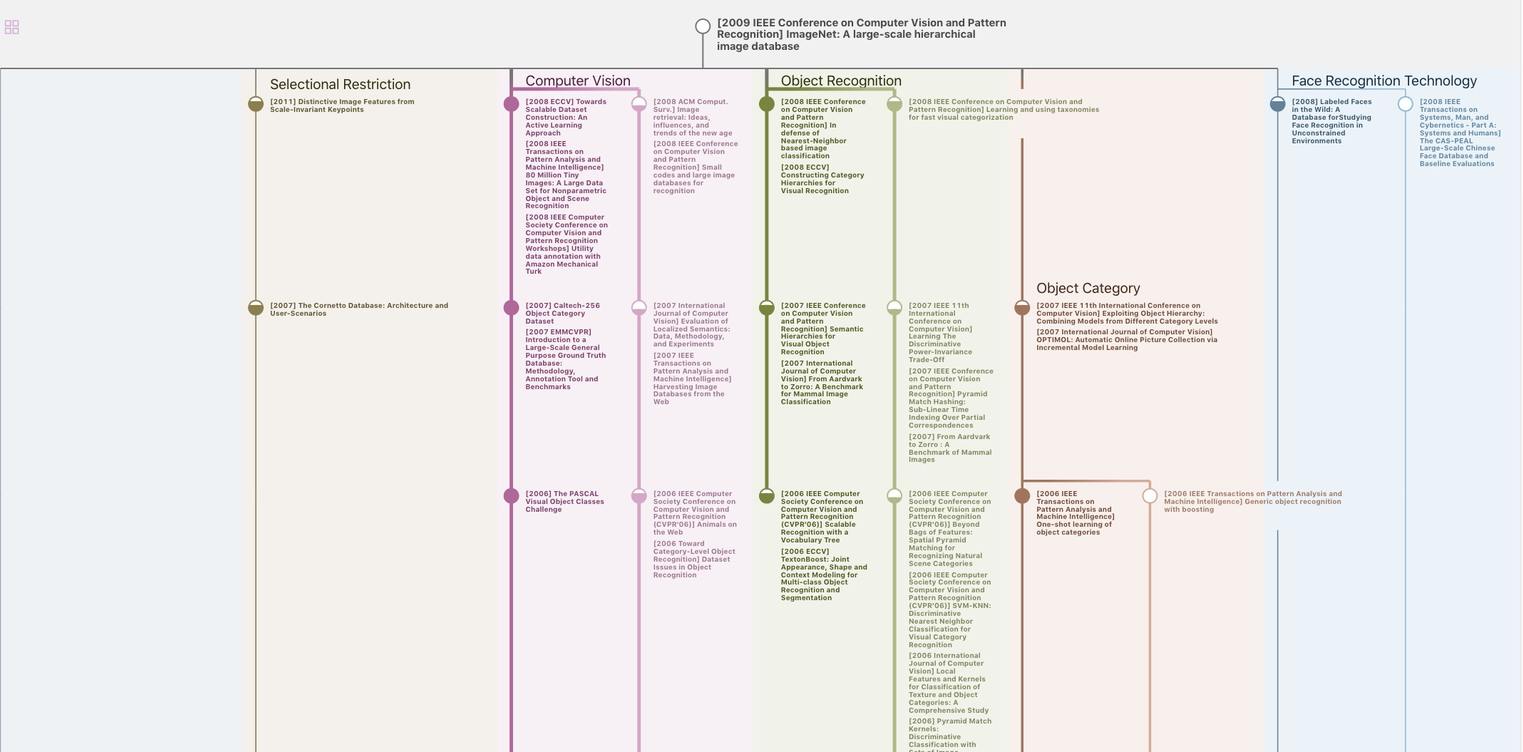
生成溯源树,研究论文发展脉络
Chat Paper
正在生成论文摘要