A Novel Graph-Based Multi-Modal Fusion Encoder For Neural Machine Translation
58TH ANNUAL MEETING OF THE ASSOCIATION FOR COMPUTATIONAL LINGUISTICS (ACL 2020)(2020)
摘要
Multi-modal neural machine translation (NMT) aims to translate source sentences into a target language paired with images However, dominant multi-modal NMT models do not fully exploit fine-grained semantic correspondences between semantic units of different modalities, which have potential to refine multi-modal representation learning. To deal with this issue, in this paper, we propose a novel graph-based multi-modal fusion encoder for NMT. Specifically, we first represent the input sentence and image using a unified multi-modal graph, which captures various semantic relationships between multi-modal semantic units (words and visual objects). We then stack multiple graph-based multi-modal fusion layers that iteratively perform semantic interactions to learn node representations. Finally, these representations provide an attention-based context vector for the decoder. We evaluate our proposed encoder on the Multi30K datasets. Experimental results and in-depth analysis show the superiority of our multi-modal NMT model.
更多查看译文
关键词
neural machine,graph-based,multi-modal
AI 理解论文
溯源树
样例
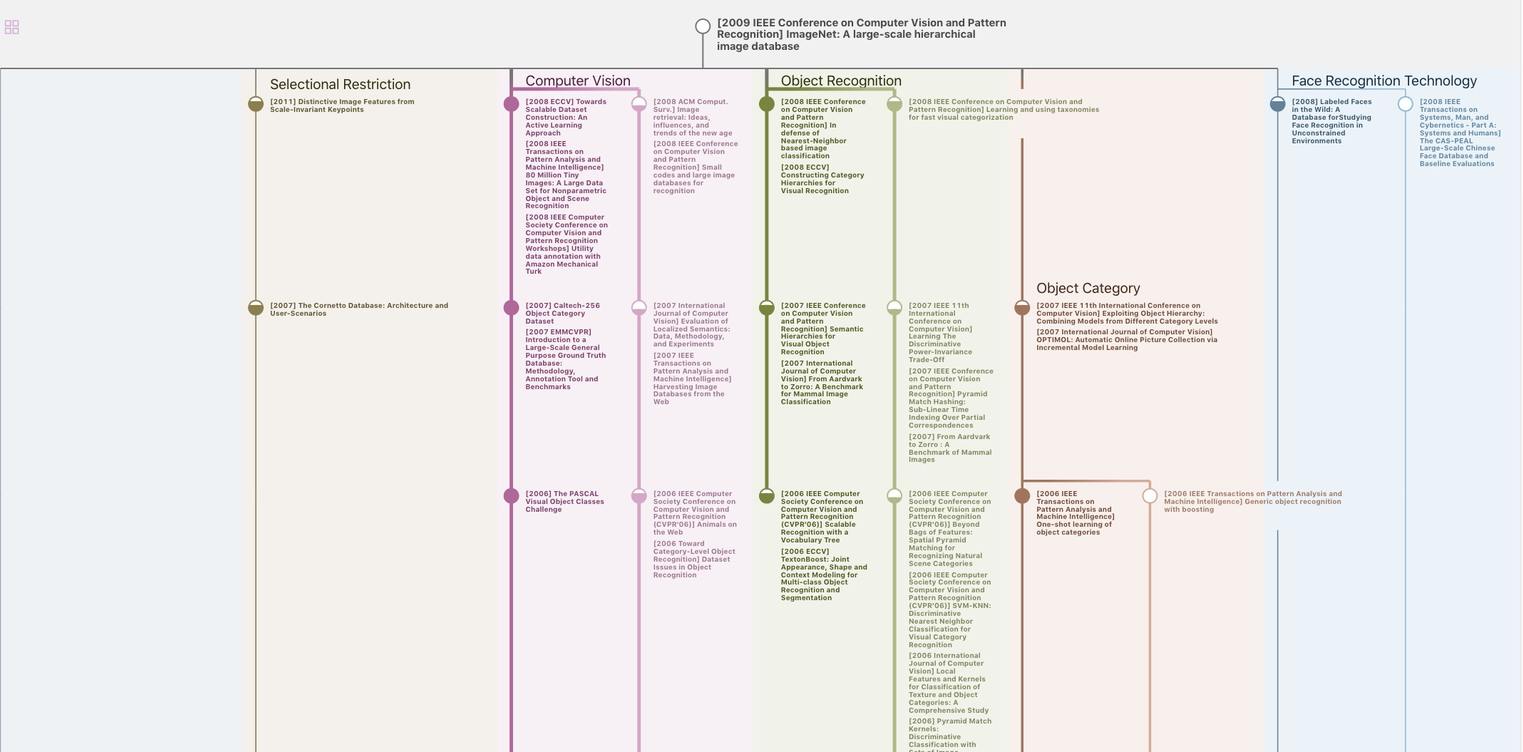
生成溯源树,研究论文发展脉络
Chat Paper
正在生成论文摘要