Identifying Statistical Bias in Dataset Replication
ICML(2020)
摘要
Dataset replication is a useful tool for assessing whether improvements in test accuracy on a specific benchmark correspond to improvements in models' ability to generalize reliably. In this work, we present unintuitive yet significant ways in which standard approaches to dataset replication introduce statistical bias, skewing the resulting observations. We study ImageNet-v2, a replication of the ImageNet dataset on which models exhibit a significant (11-14%) drop in accuracy, even after controlling for a standard human-in-the-loop measure of data quality. We show that after correcting for the identified statistical bias, only an estimated $3.6\% \pm 1.5\%$ of the original $11.7\% \pm 1.0\%$ accuracy drop remains unaccounted for. We conclude with concrete recommendations for recognizing and avoiding bias in dataset replication. Code for our study is publicly available at http://github.com/MadryLab/dataset-replication-analysis .
更多查看译文
关键词
statistical bias,replication
AI 理解论文
溯源树
样例
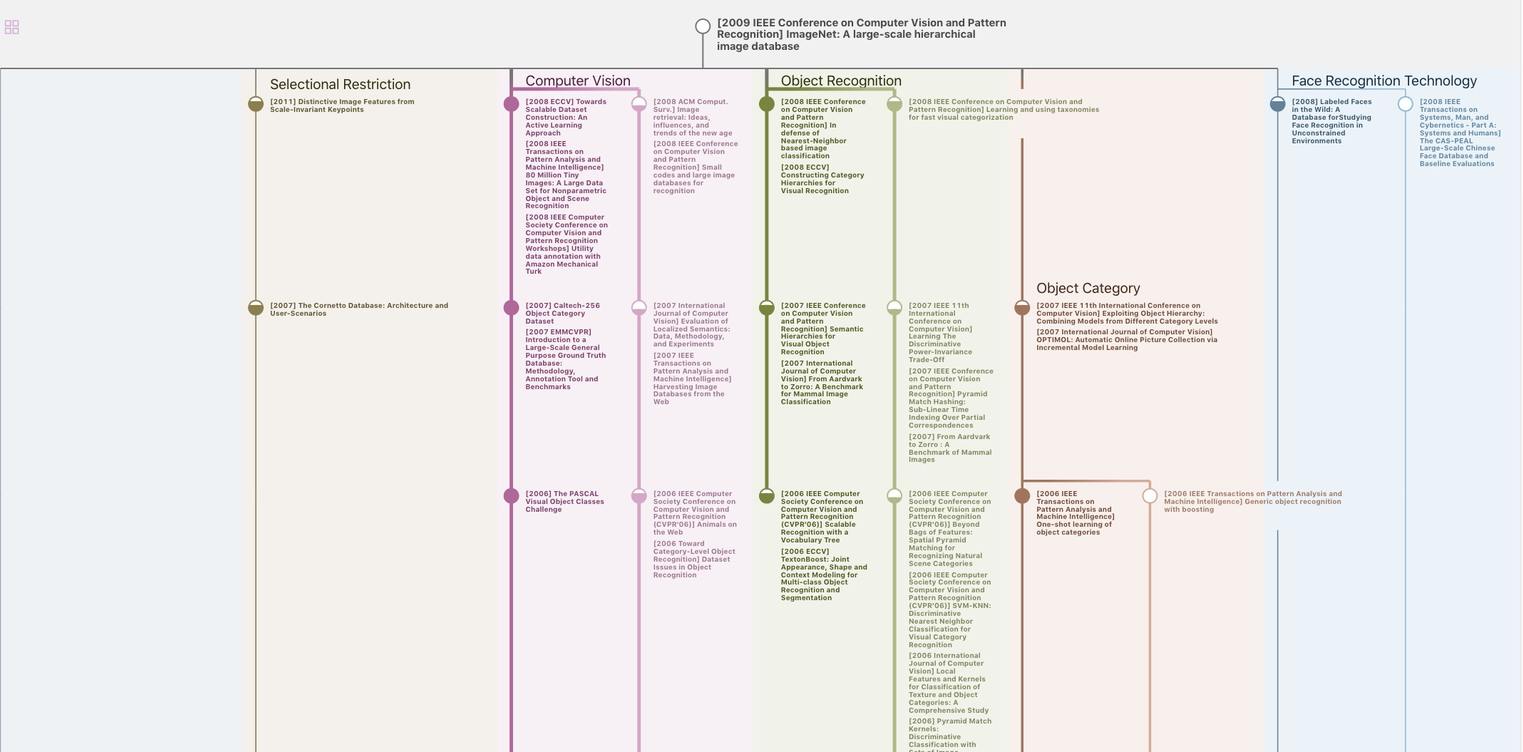
生成溯源树,研究论文发展脉络
Chat Paper
正在生成论文摘要