Ciagan: Conditional Identity Anonymization Generative Adversarial Networks
2020 IEEE/CVF CONFERENCE ON COMPUTER VISION AND PATTERN RECOGNITION (CVPR)(2020)
摘要
The unprecedented increase in the usage of computer vision technology in society goes hand in hand with an increased concern in data privacy. In many real-world scenarios like people tracking or action recognition, it is important to be able to process the data while taking careful consideration in protecting people's identity. We propose and develop CIAGAN, a model for image and video anonymization based on conditional generative adversarial networks. Our model is able to remove the identifying characteristics of faces and bodies while producing high-quality images and videos that can be used for any computer vision task, such as detection or tracking. Unlike previous methods, we have MI control over the de-identification (anonymization) procedure, ensuring both anonymization as well as diversity. We compare our method to several baselines and achieve state-of-the-art results. To facilitate further research, we make available the code and the mod els at https://github.com/dvl-tum/ciagan.
更多查看译文
关键词
video anonymization,identifying characteristics,high-quality images,computer vision task,de-identification procedure,conditional identity anonymization generative adversarial networks,computer vision technology,data privacy,action recognition,CIAGAN,image anonymization
AI 理解论文
溯源树
样例
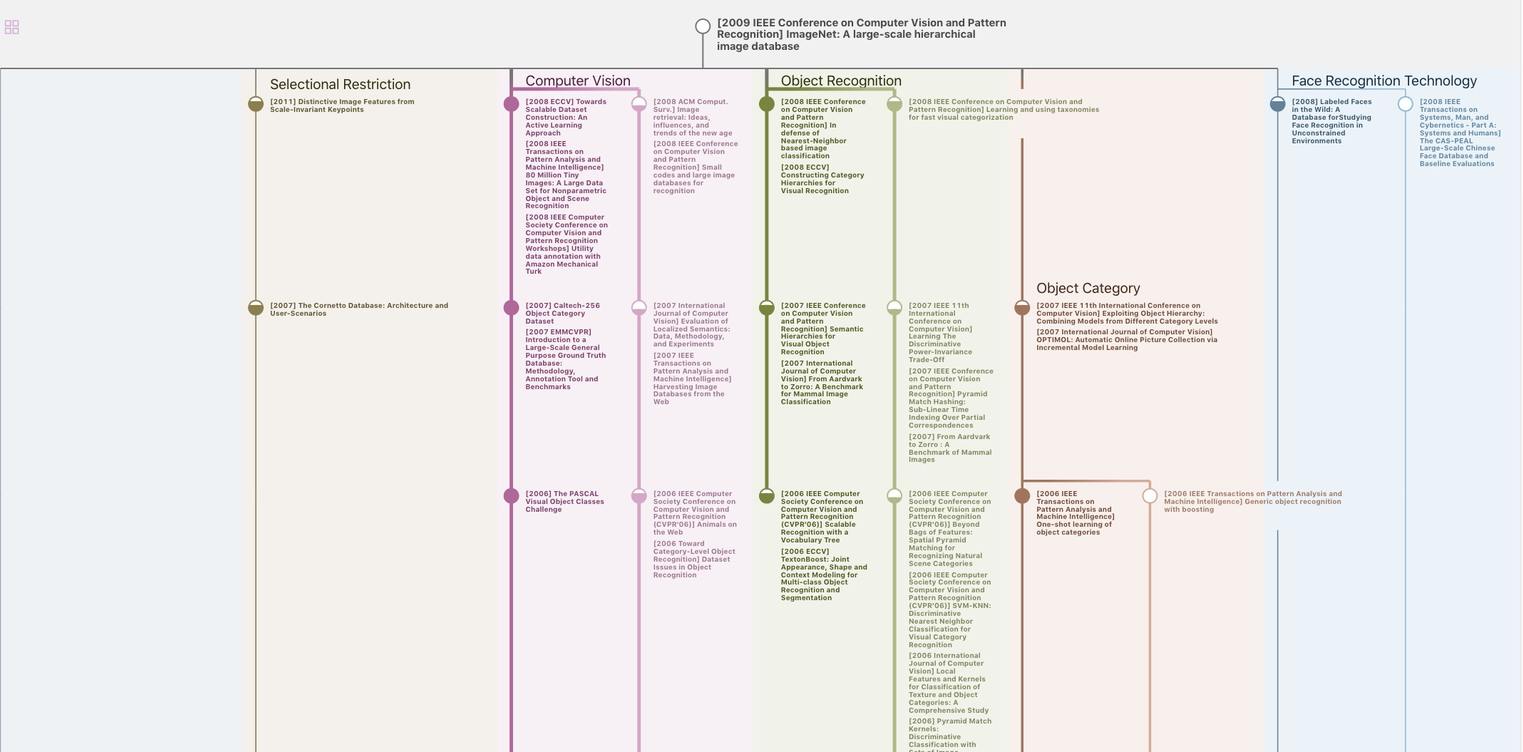
生成溯源树,研究论文发展脉络
Chat Paper
正在生成论文摘要