Bayesian Subspace HMM for the Zerospeech 2020 Challenge
arxiv(2020)
摘要
In this paper we describe our submission to the Zerospeech 2020 challenge, where the participants are required to discover latent representations from unannotated speech, and to use those representations to perform speech synthesis, with synthesis quality used as a proxy metric for the unit quality. In our system, we use the Bayesian Subspace Hidden Markov Model (SHMM) for unit discovery. The SHMM models each unit as an HMM whose parameters are constrained to lie in a low dimensional subspace of the total parameter space which is trained to model phonetic variability. Our system compares favorably with the baseline on the human-evaluated character error rate while maintaining significantly lower unit bitrate.
更多查看译文
关键词
bayesian subspace hmm,zerospeech
AI 理解论文
溯源树
样例
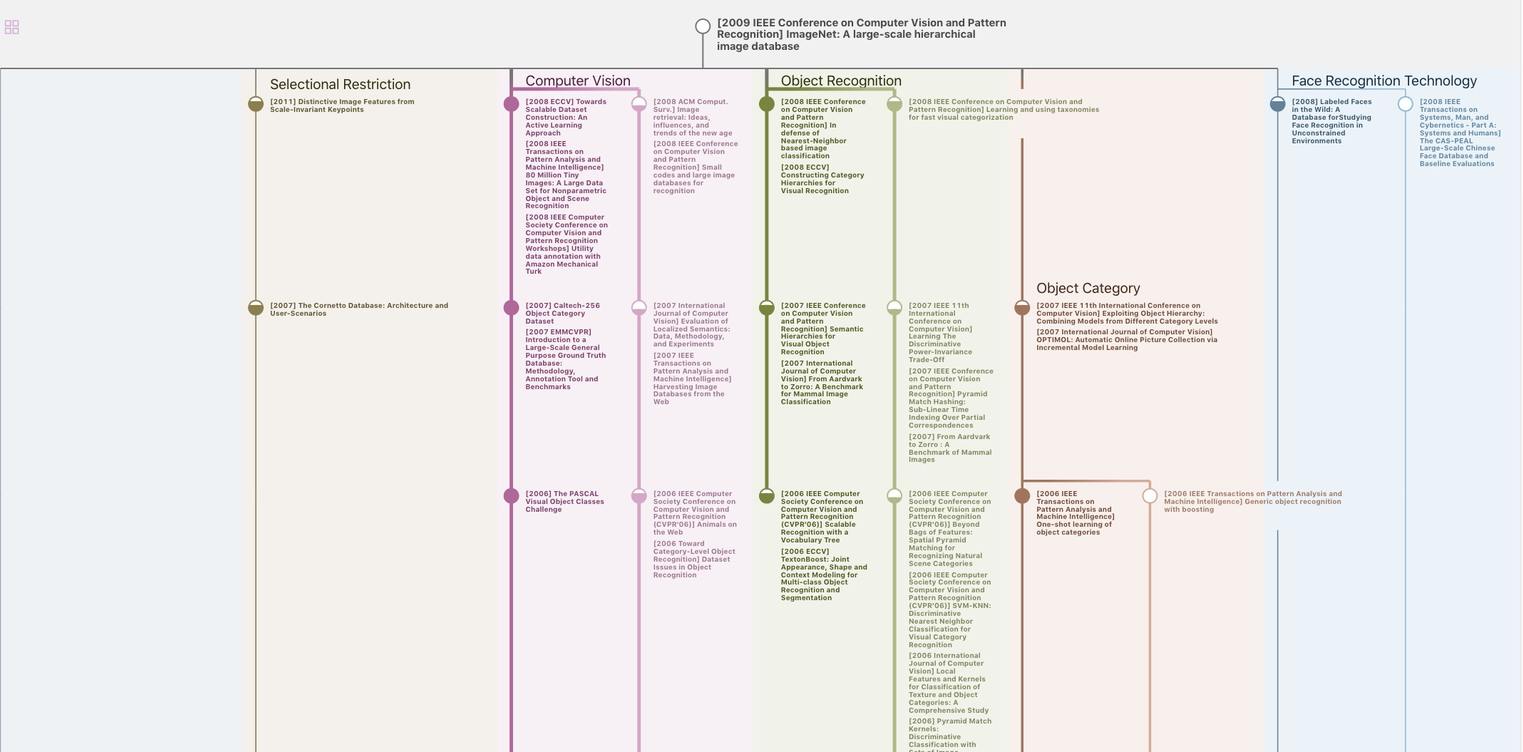
生成溯源树,研究论文发展脉络
Chat Paper
正在生成论文摘要