Safe Learning For Near-Optimal Scheduling
arxiv(2021)
摘要
In this paper, we investigate the combination of synthesis, model-based learning, and online sampling techniques to obtain safe and near-optimal schedulers for a preemptible task scheduling problem. Our algorithms can handle Markov decision processes (MDPs) that have 1020 states and beyond which cannot be handled with state-of-the art probabilistic model-checkers. We provide probably approximately correct (PAC) guarantees for learning the model. Additionally, we extend Monte-Carlo tree search with advice, computed using safety games or obtained using the earliest-deadline-first scheduler, to safely explore the learned model online. Finally, we implemented and compared our algorithms empirically against shielded deep Q-learning on large task systems.
更多查看译文
关键词
Model-based learning, Monte-Carlo tree search, Task scheduling
AI 理解论文
溯源树
样例
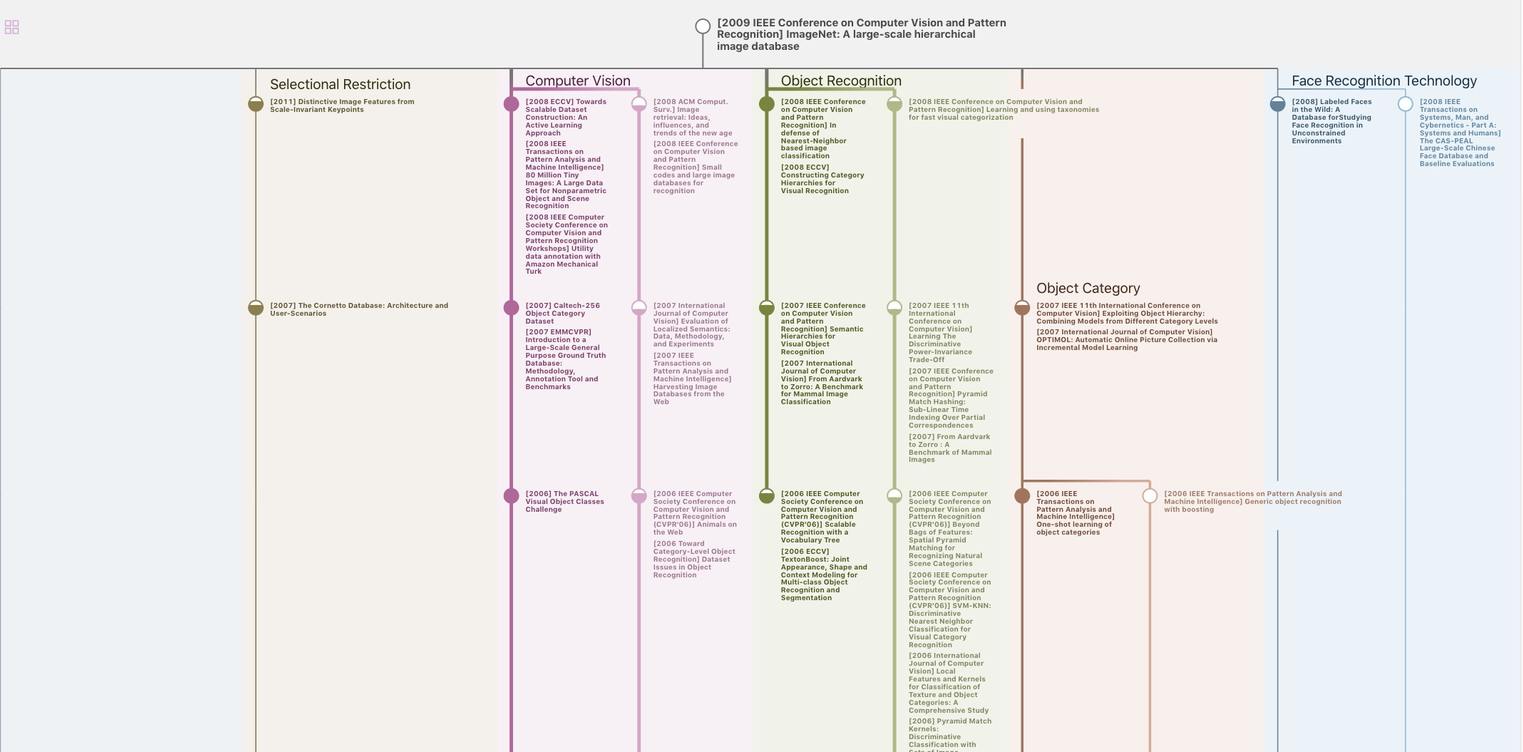
生成溯源树,研究论文发展脉络
Chat Paper
正在生成论文摘要