Many-to-Many Voice Transformer Network
IEEE/ACM Transactions on Audio, Speech, and Language Processing(2021)
摘要
This paper proposes a voice conversion (VC) method based on a sequence-to-sequence (S2S) learning framework, which enables simultaneous conversion of the voice characteristics, pitch contour, and duration of input speech. We previously proposed an S2S-based VC method using a transformer network architecture called the voice transformer network (VTN). The original VTN was designed to learn only a mapping of speech feature sequences from one speaker to another. Here, the main idea we propose is an extension of the original VTN that can simultaneously learn mappings among multiple speakers. This extension, called the many-to-many VTN, enables us to fully use available training data collected from multiple speakers by capturing common latent features that can be shared across different speakers. It also allows us to introduce a training loss called the identity mapping loss to ensure that the input feature sequence will remain unchanged when the source and target speaker indices are the same. Using this particular loss for model training has been found to be extremely effective in improving the performance of the model at test time. We conducted speaker identity conversion experiments and found that our model obtained higher sound quality and speaker similarity than baseline methods. We also found that our model, with a slight modification to its architecture, can handle any-to-many conversion tasks reasonably well.
更多查看译文
关键词
Attention,many-to-many VC,sequence-to-sequence learning,voice conversion (VC),transformer network
AI 理解论文
溯源树
样例
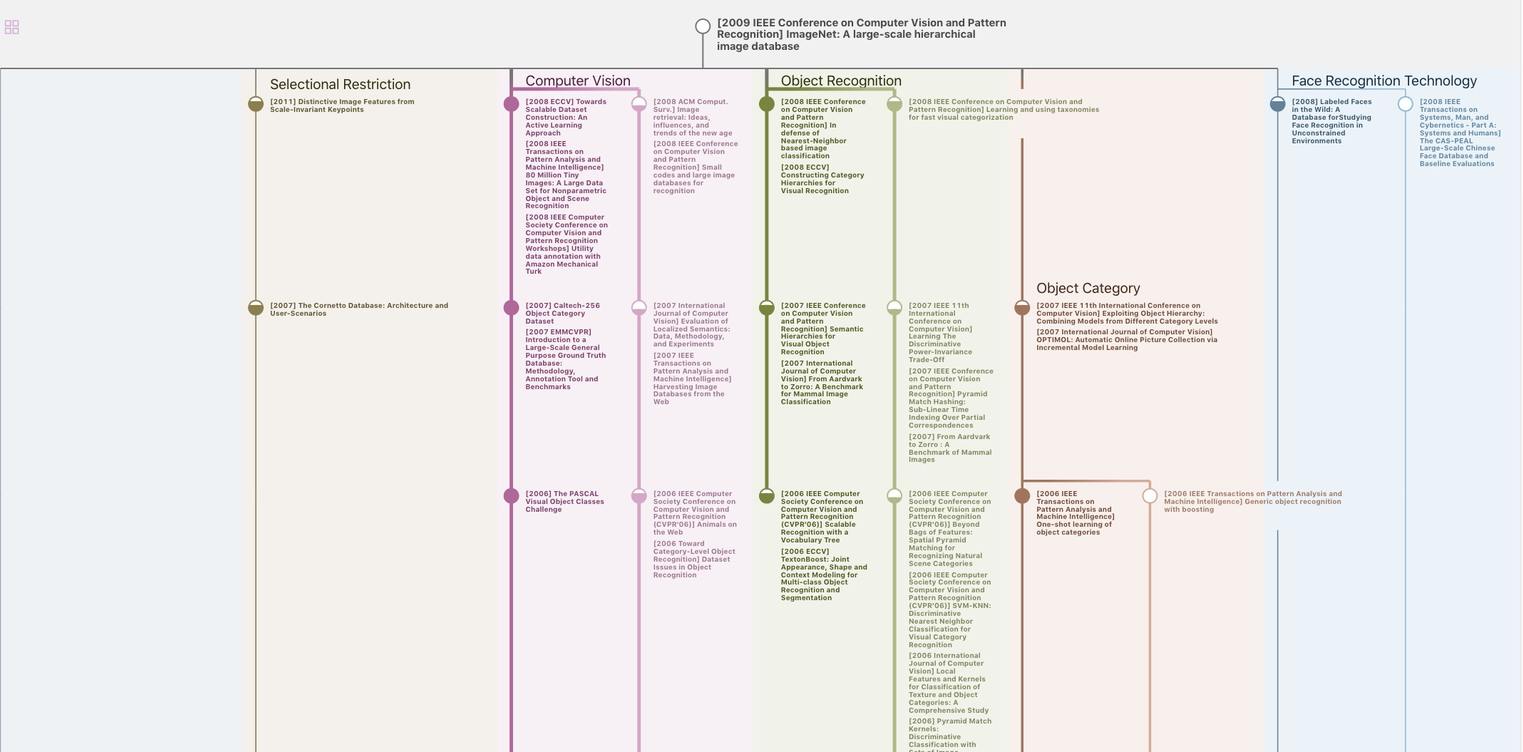
生成溯源树,研究论文发展脉络
Chat Paper
正在生成论文摘要