Vector-Quantized Autoregressive Predictive Coding
INTERSPEECH(2020)
摘要
Autoregressive Predictive Coding (APC), as a self-supervised objective, has enjoyed success in learning representations from large amounts of unlabeled data, and the learned representations are rich for many downstream tasks. However, the connection between low self-supervised loss and strong performance in downstream tasks remains unclear. In this work, we propose Vector-Quantized Autoregressive Predictive Coding (VQ-APC), a novel model that produces quantized representations, allowing us to explicitly control the amount of information encoded in the representations. By studying a sequence of increasingly limited models, we reveal the constituents of the learned representations. In particular, we confirm the presence of information with probing tasks, while showing the absence of information with mutual information, uncovering the model's preference in preserving speech information as its capacity becomes constrained. We find that there exists a point where phonetic and speaker information are amplified to maximize a self-supervised objective. As a byproduct, the learned codes for a particular model capacity correspond well to English phones.
更多查看译文
关键词
predictive,vector-quantized
AI 理解论文
溯源树
样例
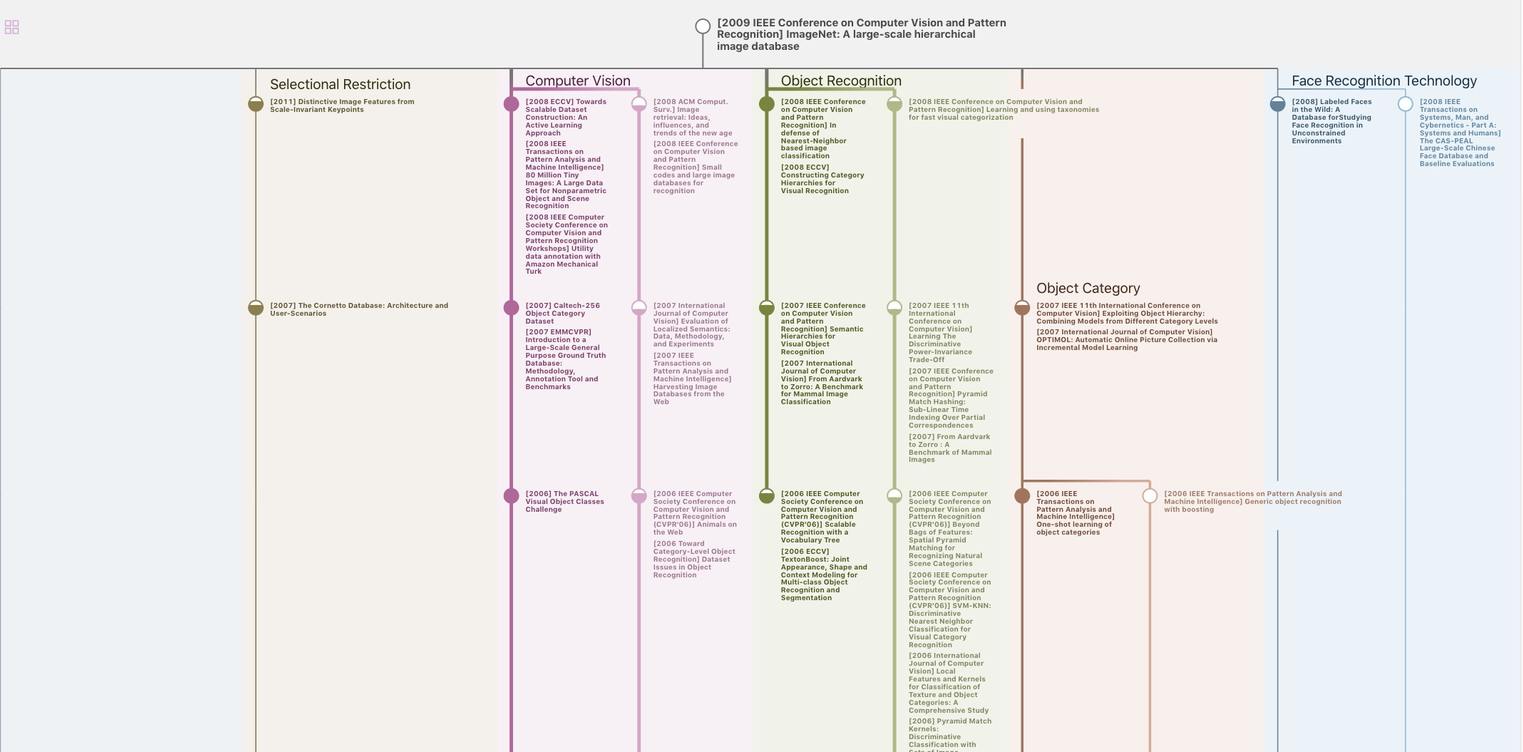
生成溯源树,研究论文发展脉络
Chat Paper
正在生成论文摘要