Structural Temporal Graph Neural Networks for Anomaly Detection in Dynamic Graphs
Conference on Information and Knowledge Management(2021)
摘要
ABSTRACTDetecting anomalies in dynamic graphs is a vital task, with numerous practical applications in areas such as security, finance, and social media. Existing network embedding based methods have mostly focused on learning good node representations, whereas largely ignoring the subgraph structural changes related to the target nodes in a given time window. In this paper, we propose StrGNN, an end-to-end structural temporal Graph Neural Network model for detecting anomalous edges in dynamic graphs. In particular, we first extract the h-hop enclosing subgraph centered on the target edge and propose a node labeling function to identify the role of each node in the subgraph. Then, we leverage the graph convolution operation and Sortpooling layer to extract the fixed-size feature from each snapshot/timestamp. Based on the extracted features, we utilize the Gated Recurrent Units to capture the temporal information for anomaly detection. We fully implement StrGNN and deploy it into a real enterprise security system, and it greatly helps detect advanced threats and optimize the incident response. Extensive experiments on six benchmark datasets also demonstrate the effectiveness of StrGNN.
更多查看译文
关键词
graph neural networks,dynamic graphs,anomaly detection
AI 理解论文
溯源树
样例
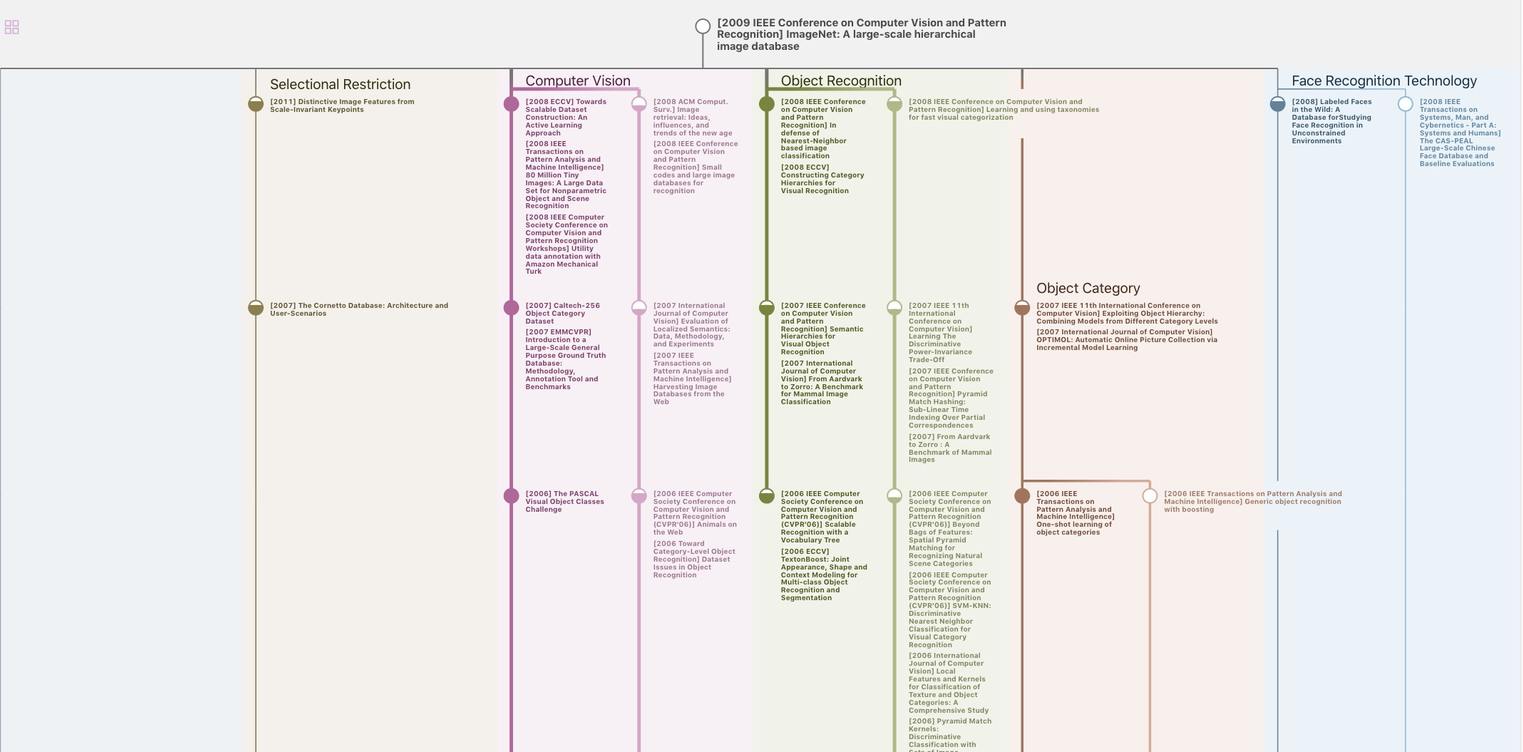
生成溯源树,研究论文发展脉络
Chat Paper
正在生成论文摘要