Towards Assessment of Randomized Mechanisms for Certifying Adversarial Robustness
arxiv(2020)
摘要
As a certified defensive technique, randomized smoothing has received considerable attention due to its scalability to large datasets and neural networks. However, several important questions remain unanswered, such as (i) whether the Gaussian mechanism is an appropriate option for certifying $\ell_2$-norm robustness, and (ii) whether there is an appropriate randomized mechanism to certify $\ell_\infty$-norm robustness on high-dimensional datasets. To shed light on these questions, we introduce a generic framework that connects the existing frameworks to assess randomized mechanisms. Under our framework, we define the magnitude of the noise required by a mechanism to certify a certain extent of robustness as the metric for assessing the appropriateness of the mechanism. We also derive lower bounds on the metric as the criteria for assessment. Assessment of Gaussian and Exponential mechanisms is achieved by comparing the magnitude of noise needed by these mechanisms and the criteria, and we conclude that the Gaussian mechanism is an appropriate option to certify both $\ell_2$-norm and $\ell_\infty$-norm robustness. The veracity of our framework is verified by evaluations on CIFAR10 and ImageNet.
更多查看译文
关键词
adversarial robustness,randomized mechanisms
AI 理解论文
溯源树
样例
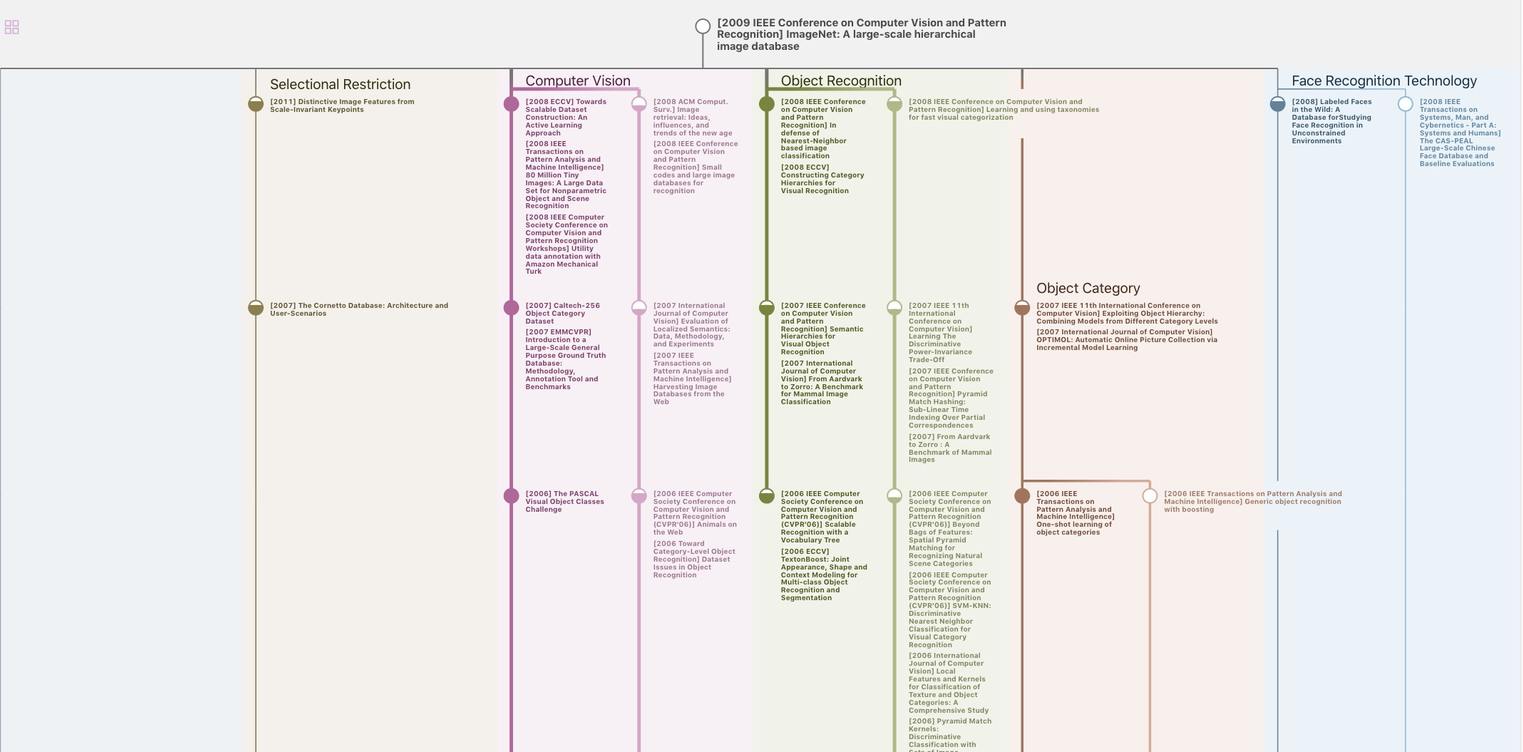
生成溯源树,研究论文发展脉络
Chat Paper
正在生成论文摘要