Investigating bias in deep face analysis: The KANFace dataset and empirical study
Image and Vision Computing(2020)
摘要
Deep learning-based methods have pushed the limits of the state-of-the-art in face analysis. However, despite their success, these models have raised concerns regarding their bias towards certain demographics. This bias is inflicted both by limited diversity across demographics in the training set, as well as the design of the algorithms. In this work, we investigate the demographic bias of deep learning models in face recognition, age estimation, gender recognition and kinship verification. To this end, we introduce the most comprehensive, large-scale dataset of facial images and videos to date. It consists of 40K still images and 44K sequences (14.5M video frames in total) captured in unconstrained, real-world conditions from 1,045 subjects. The data are manually annotated in terms of identity, exact age, gender and kinship. The performance of state-of-the-art models is scrutinized and demographic bias is exposed by conducting a series of experiments. Lastly, a method to debias network embeddings is introduced and tested on the proposed benchmarks.
更多查看译文
关键词
Dataset bias,Face recognition,Age estimation,Gender recognition,Kinship verification
AI 理解论文
溯源树
样例
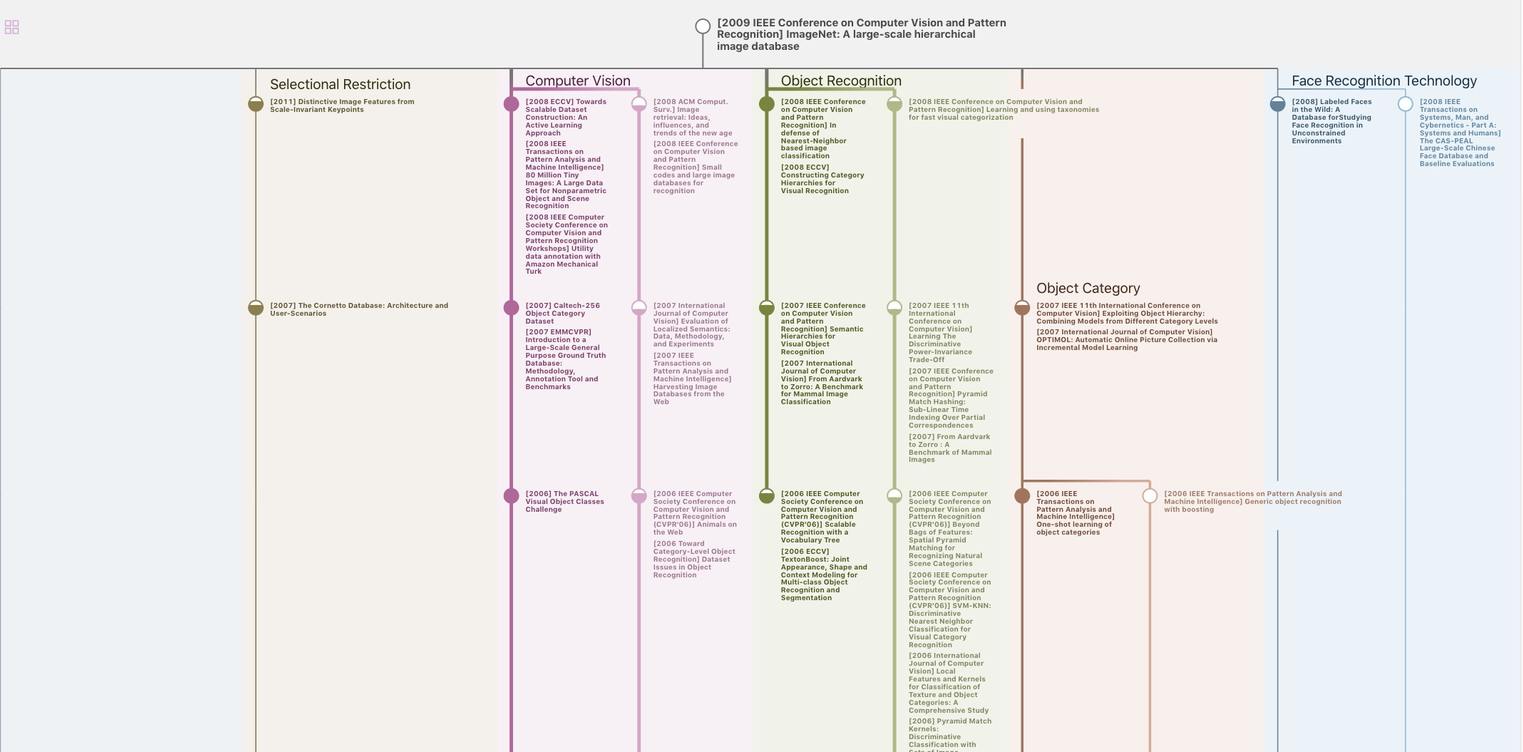
生成溯源树,研究论文发展脉络
Chat Paper
正在生成论文摘要