Federated Recommendation System via Differential Privacy
ISIT(2020)
摘要
In this paper we are interested in what we term the federated private bandits framework, that combines differential privacy with multi-agent bandit learning. We explore how differential privacy based Upper Confidence Bound (UCB) methods can be applied to multi-agent environments, and in particular to federated learning environments both in ‘master-worker’ and ‘fully decentralized’ settings. We provide theoretical analysis on the privacy and regret performance of the proposed methods and explore the tradeoffs between these two.
更多查看译文
关键词
Federated learning, multi-arm bandit, differential privacy, distributed learning
AI 理解论文
溯源树
样例
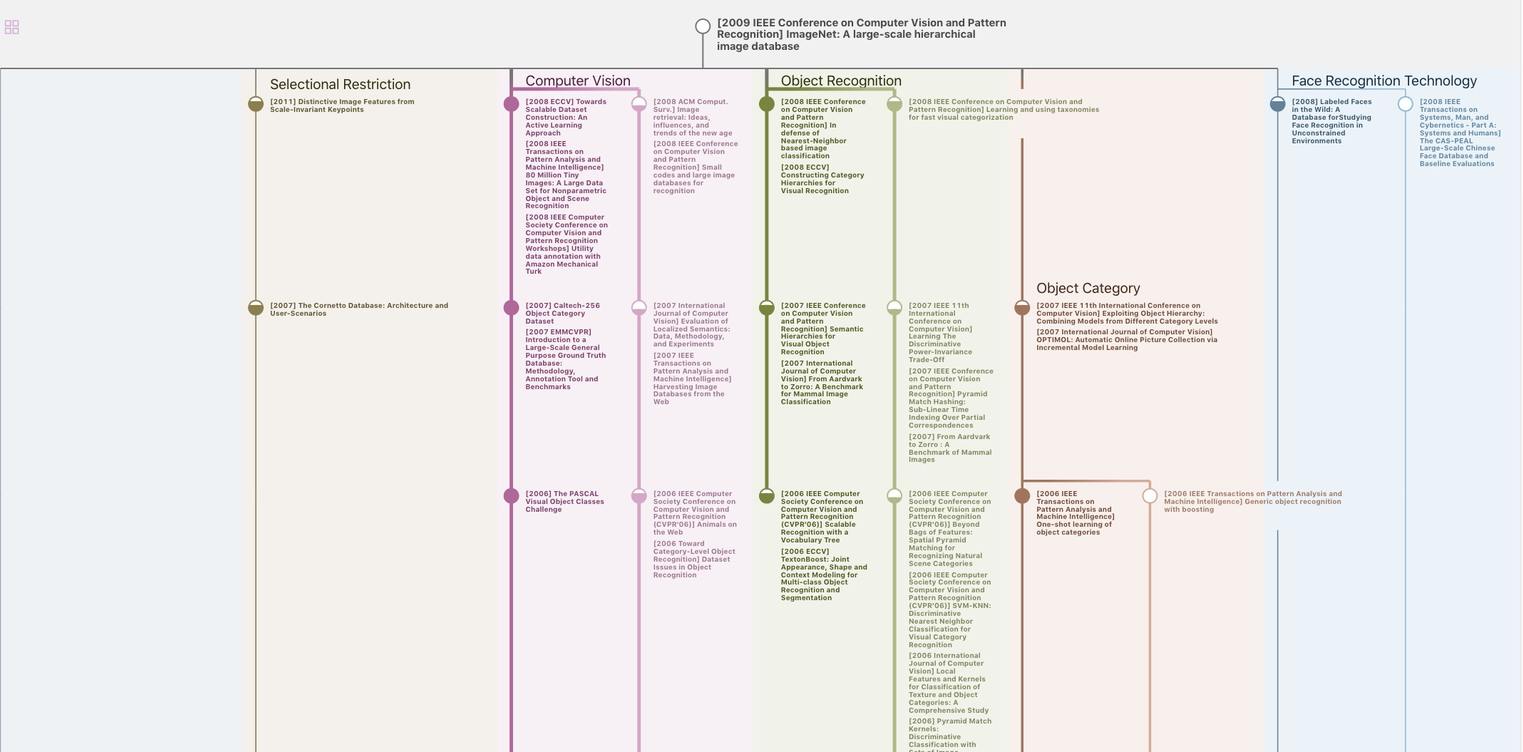
生成溯源树,研究论文发展脉络
Chat Paper
正在生成论文摘要