Memory Controlled Sequential Self Attention for Sound Recognition
INTERSPEECH(2020)
摘要
In this paper we investigate the importance of the extent of memory in sequential self attention for sound recognition. We propose to use a memory controlled sequential self attention mechanism on top of a convolutional recurrent neural network (CRNN) model for polyphonic sound event detection (SED). Experiments on the URBAN-SED dataset demonstrate the impact of the extent of memory on sound recognition performance with the self attention induced SED model. We extend the proposed idea with a multi-head self attention mechanism where each attention head processes the audio embedding with explicit attention width values. The proposed use of memory controlled sequential self attention offers a way to induce relations among frames of sound event tokens. We show that our memory controlled self attention model achieves an event based F -score of 33.92% on the URBAN-SED dataset, outperforming the F -score of 20.10% reported by the model without self attention.
更多查看译文
关键词
Memory controlled self attention, sound recognition, multi-head attention
AI 理解论文
溯源树
样例
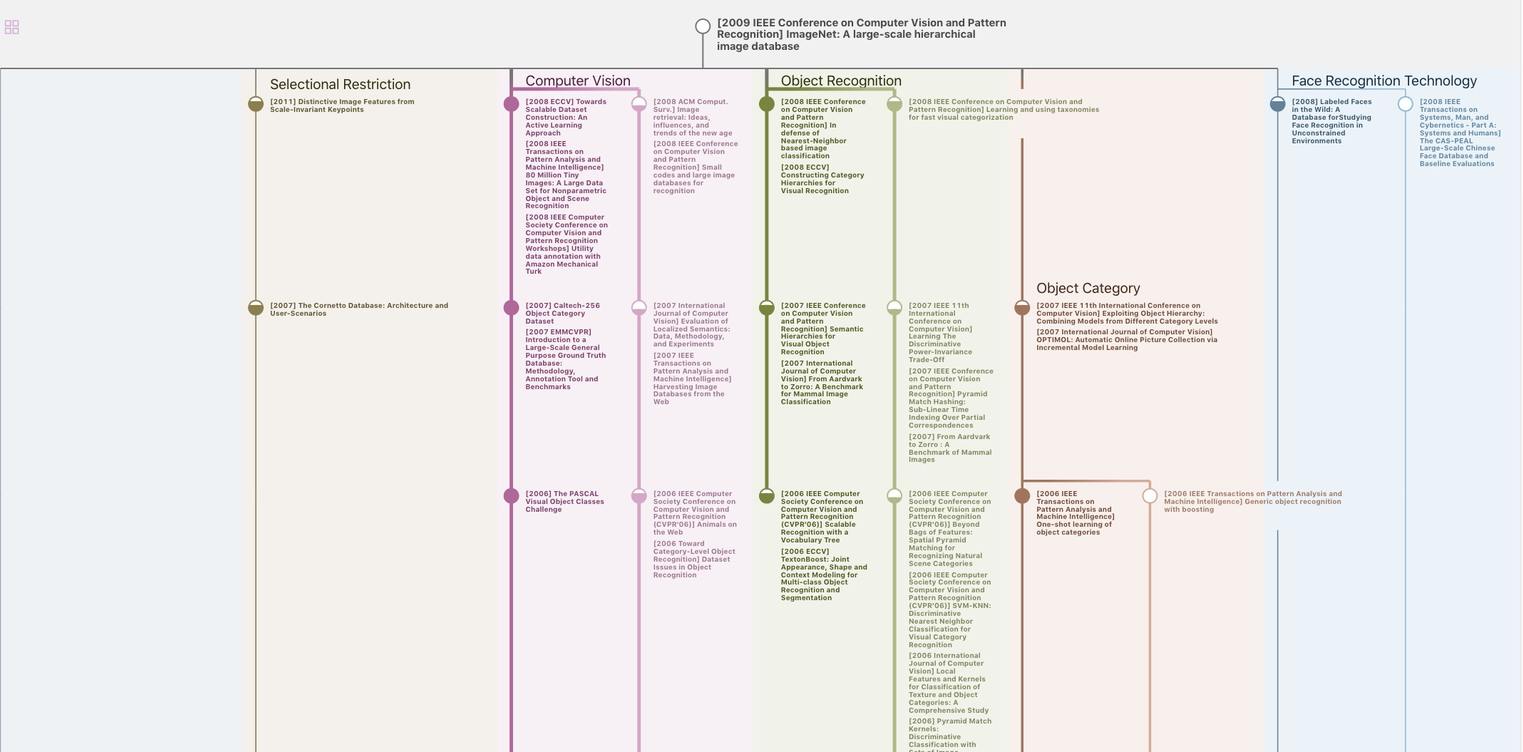
生成溯源树,研究论文发展脉络
Chat Paper
正在生成论文摘要