Modeling Human Dynamics and Lifestyle Using Digital Traces
arxiv(2020)
摘要
Human behavior drives a range of complex social, urban, and economic systems, yet understanding its structure and dynamics at the individual level remains an open question. From credit card transactions to communications data, human behavior appears to exhibit bursts of activity driven by task prioritization and periodicity, however, current research does not offer generative models capturing these mechanisms. We propose a multivariate, periodic Hawkes process (MPHP) model that captures -- at the individual level -- the temporal clustering of human activity, the interdependence structure and co-excitation of different activities, and the periodic effects of weekly rhythms. We also propose a scalable parameter estimation technique for this model using maximum-aposteriori expectation-maximization that additionally provides estimation of latent variables revealing branching structure of an individual's behavior patterns. We apply the model to a large dataset of credit card transactions, and demonstrate the MPHP outperforms a non-homogeneous Poisson model and LDA in both statistical fit for the distribution of inter-event times and an activity prediction task.
更多查看译文
AI 理解论文
溯源树
样例
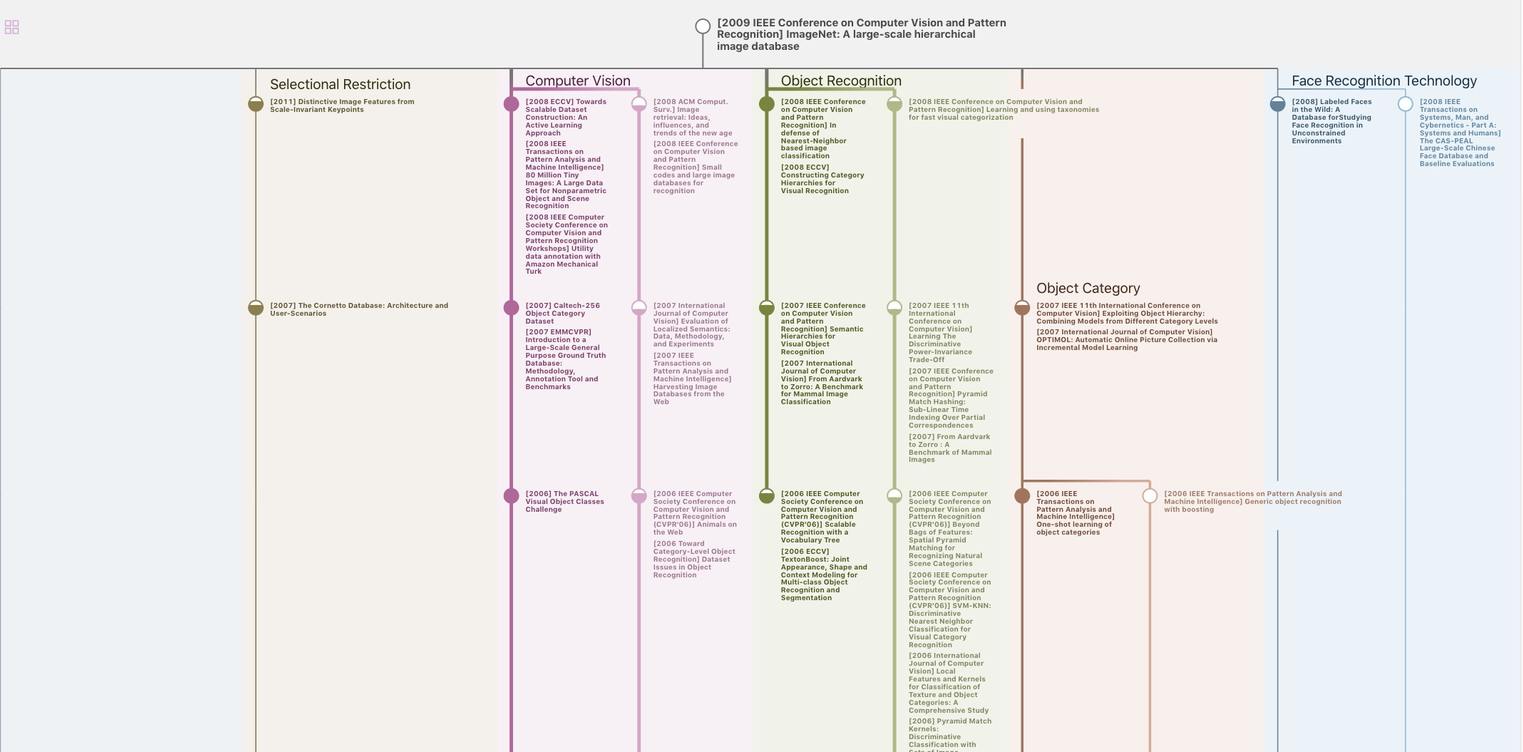
生成溯源树,研究论文发展脉络
Chat Paper
正在生成论文摘要