3D Scene Geometry-Aware Constraint for Camera Localization with Deep Learning
ICRA(2020)
摘要
Camera localization is a fundamental and key component of autonomous driving vehicles and mobile robots to localize themselves globally for further environment perception, path planning and motion control. Recently end-to-end approaches based on convolutional neural network have been much studied to achieve or even exceed 3D-geometry based traditional methods. In this work, we propose a compact network for absolute camera pose regression. Inspired from those traditional methods, a 3D scene geometry-aware constraint is also introduced by exploiting all available information including motion, depth and image contents. We add this constraint as a regularization term to our proposed network by defining a pixel-level photometric loss and an image-level structural similarity loss. To benchmark our method, different challenging scenes including indoor and outdoor environment are tested with our proposed approach and state-of-the-arts. And the experimental results demonstrate significant performance improvement of our method on both prediction accuracy and convergence efficiency.
更多查看译文
关键词
3D scene geometry-aware constraint,camera localization,deep learning,fundamental component,autonomous driving vehicles,path planning,motion control,end-to-end approaches,convolutional neural network,3D-geometry based traditional methods,compact network,absolute camera,image contents,image-level structural similarity loss,challenging scenes
AI 理解论文
溯源树
样例
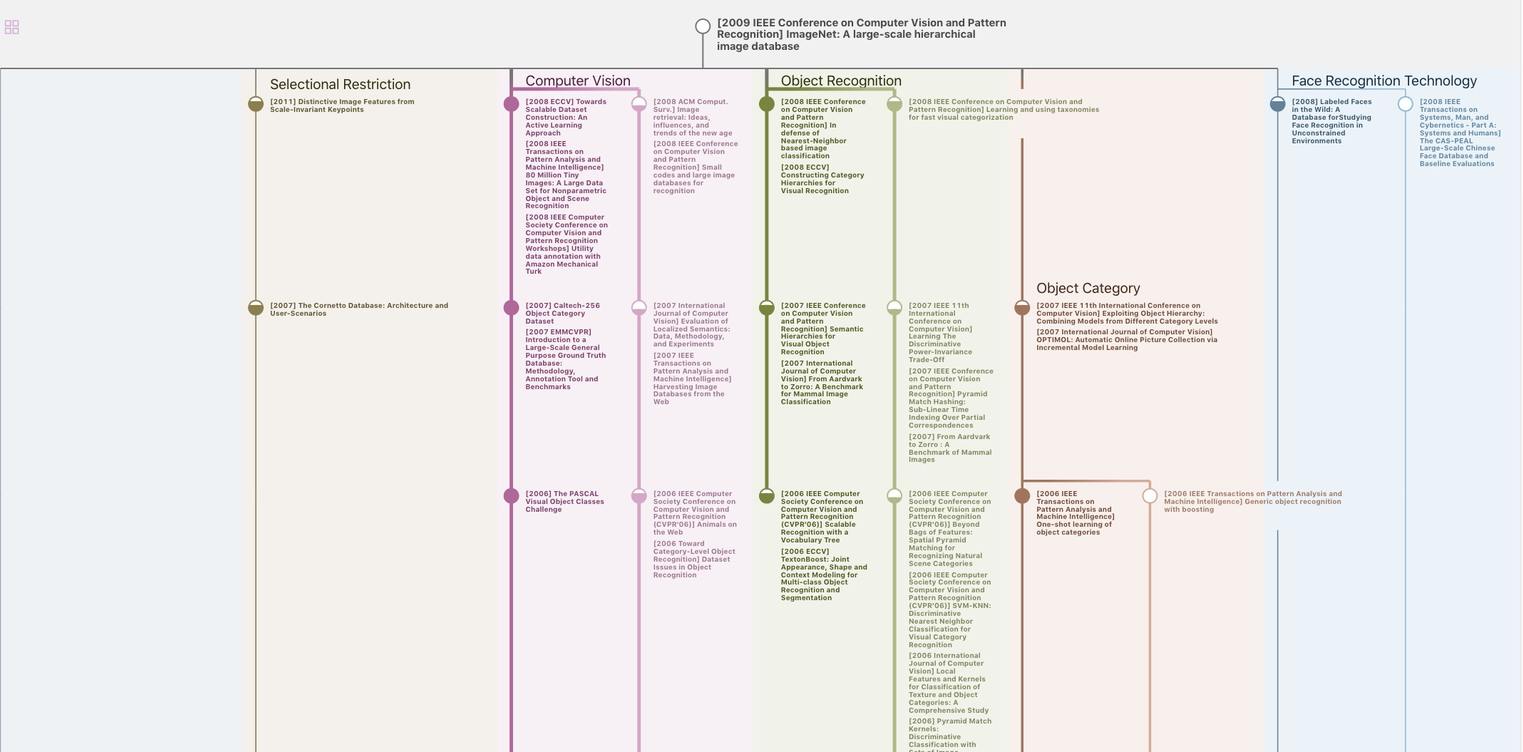
生成溯源树,研究论文发展脉络
Chat Paper
正在生成论文摘要