Leveraging Communication Topologies Between Learning Agents in Deep Reinforcement Learning
AAMAS '19: International Conference on Autonomous Agents and Multiagent Systems Auckland New Zealand May, 2020(2020)
摘要
A common technique to improve learning performance in deep reinforcement learning (DRL) and many other machine learning algorithms is to run multiple learning agents in parallel [7, 11]. A neglected component in the development of these algorithms has been how best to arrange the learning agents involved to improve distributed search [1-3, 13]. Here we draw upon results from the networked optimization literatures [4-6] suggesting that arranging learning agents in communication networks other than fully connected topologies (the implicit way agents are commonly arranged in) can improve learning. As shown in Fig. 2, our intuition is that decentralized communication topologies will lead to clusters of agents searching different parts of the landscape simultaneously.
更多查看译文
AI 理解论文
溯源树
样例
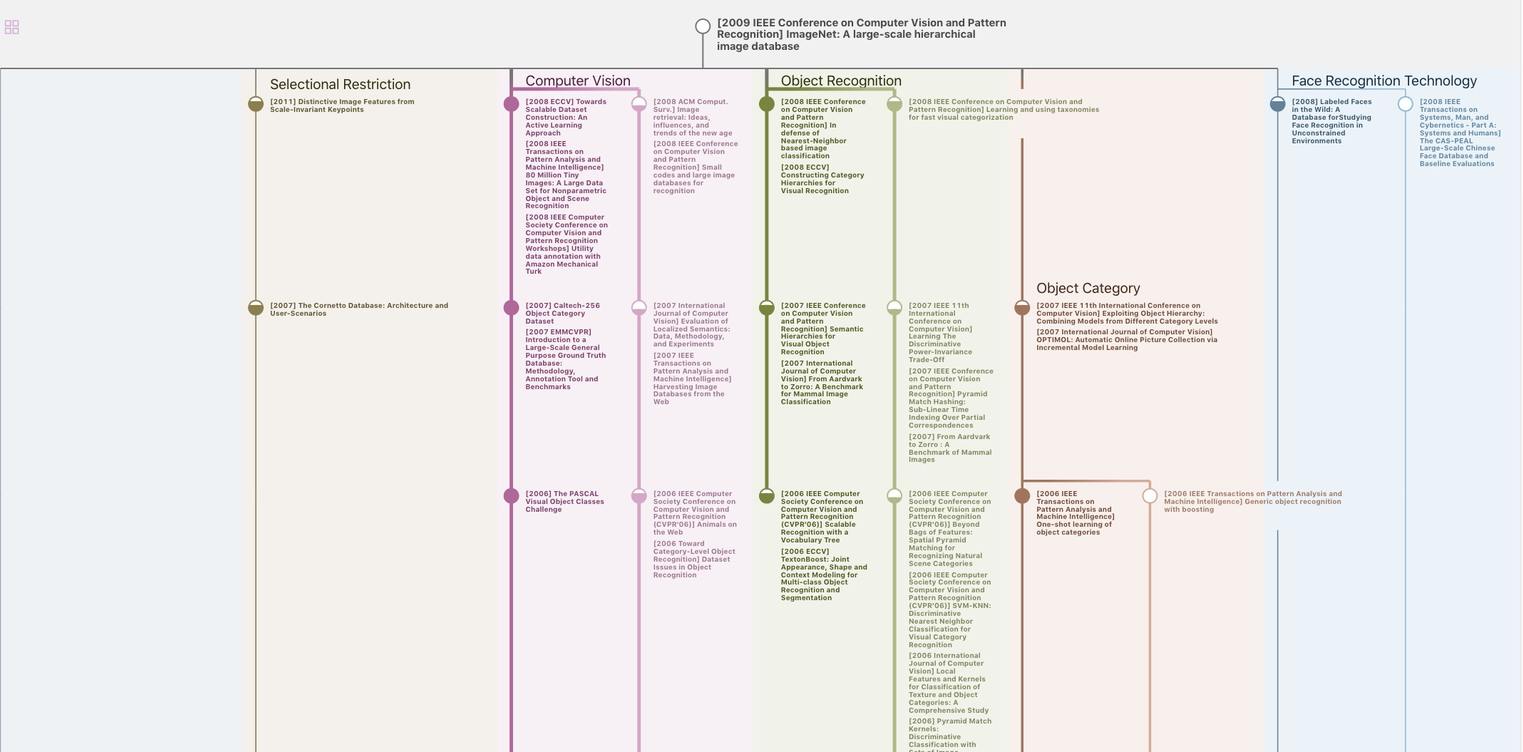
生成溯源树,研究论文发展脉络
Chat Paper
正在生成论文摘要