Combining Supervised and Unsupervised Machine Learning Methods for Phenotypic Functional Genomics Screening.
SLAS DISCOVERY(2020)
摘要
There has been an increase in the use of machine learning and artificial intelligence (AI) for the analysis of image-based cellular screens. The accuracy of these analyses, however, is greatly dependent on the quality of the training sets used for building the machine learning models. We propose that unsupervised exploratory methods should first be applied to the data set to gain a better insight into the quality of the data. This improves the selection and labeling of data for creating training sets before the application of machine learning. We demonstrate this using a high-content genome-wide small interfering RNA screen. We perform an unsupervised exploratory data analysis to facilitate the identification of four robust phenotypes, which we subsequently use as a training set for building a high-quality random forest machine learning model to differentiate four phenotypes with an accuracy of 91.1% and a kappa of 0.85. Our approach enhanced our ability to extract new knowledge from the screen when compared with the use of unsupervised methods alone.
更多查看译文
关键词
artificial intelligence,supervised machine learning,classification,phenotypic profiles
AI 理解论文
溯源树
样例
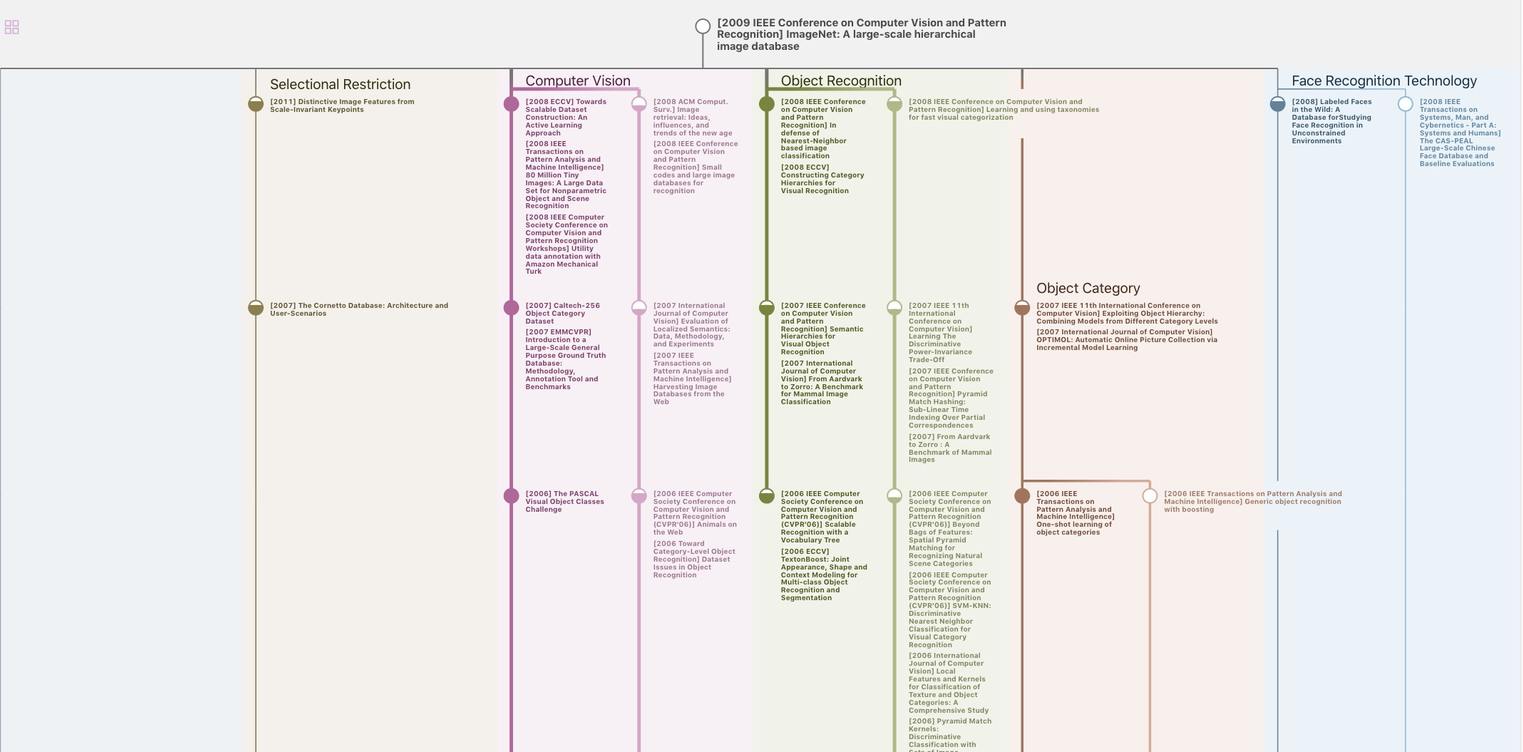
生成溯源树,研究论文发展脉络
Chat Paper
正在生成论文摘要