MOReL : Model-Based Offline Reinforcement Learning
NIPS 2020(2020)
摘要
In offline reinforcement learning (RL), the goal is to learn a successful policy using only a dataset of historical interactions with the environment, without any additional online interactions. This serves as an extreme test for an agent's ability to effectively use historical data, which is critical for efficient RL. Prior work in offline RL has been confined almost exclusively to model-free RL approaches. In this work, we present MOReL, an algorithmic framework for model-based RL in the offline setting. This framework consists of two steps: (a) learning a pessimistic MDP model using the offline dataset; (b) learning a near-optimal policy in the learned pessimistic MDP. The construction of the pessimistic MDP is such that for any policy, the performance in the real environment is lower bounded by the performance in the pessimistic MDP. This enables the pessimistic MDP to serve as a good surrogate for the purposes of policy evaluation and learning. Overall, MOReL is amenable to detailed theoretical analysis, enables easy and transparent design of practical algorithms, and leads to state-of-the-art results on widely studied offline RL benchmark tasks.
更多查看译文
关键词
reinforcement,learning,model-based
AI 理解论文
溯源树
样例
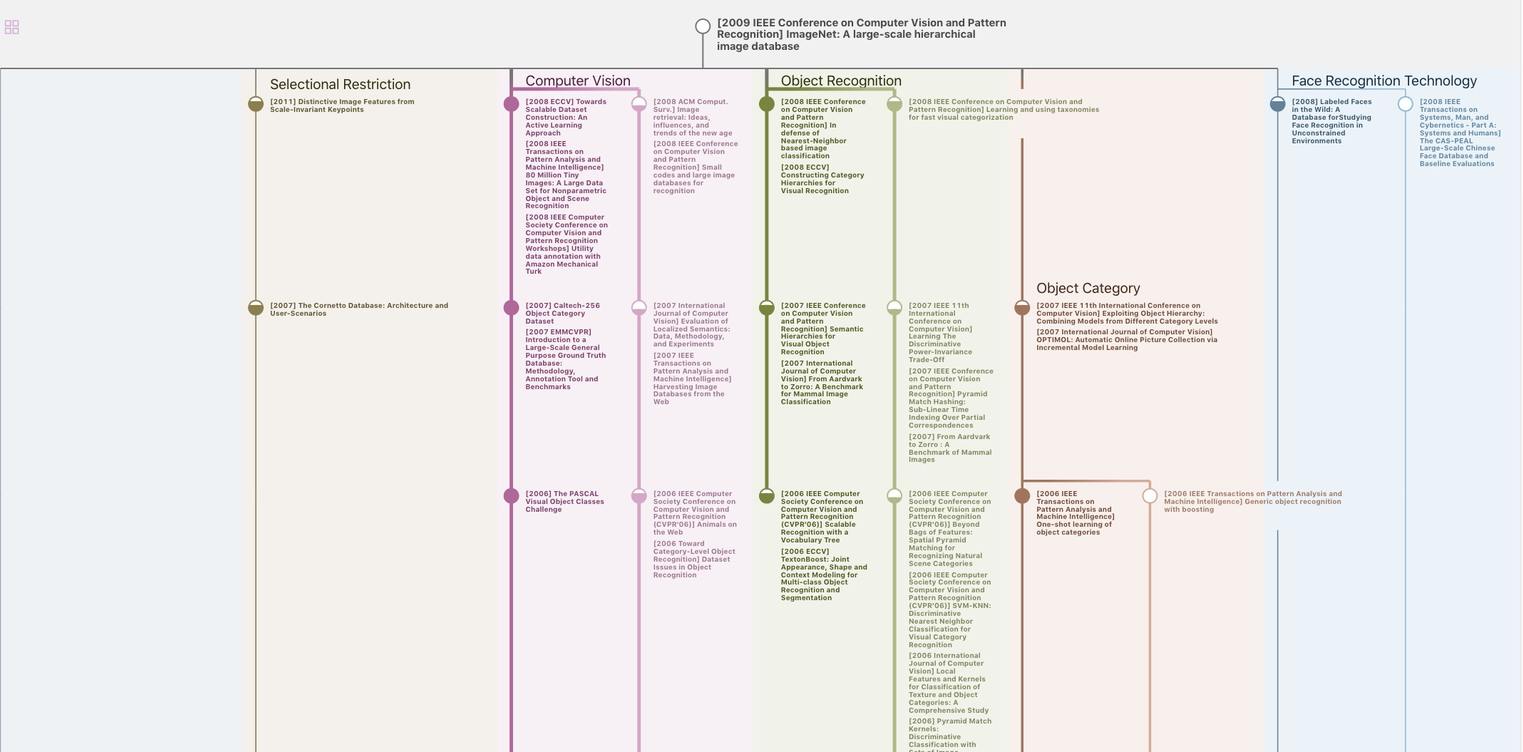
生成溯源树,研究论文发展脉络
Chat Paper
正在生成论文摘要