Real-time Facial Expression Recognition “In The Wild” by Disentangling 3D Expression from Identity
2020 15th IEEE International Conference on Automatic Face and Gesture Recognition (FG 2020)(2020)
摘要
Human emotions analysis has been the focus of many studies, especially in the field of Affective Computing, and is important for many applications, e.g. human-computer intelligent interaction, stress analysis, interactive games, animations, etc. Solutions for automatic emotion analysis have also benefited from the development of deep learning approaches and the availability of vast amount of visual facial data on the internet. This paper proposes a novel method for human emotion recognition from a single RGB image. We construct a largescale dataset of facial videos (FaceVid), rich in facial dynamics, identities, expressions, appearance and 3D pose variations. We use this dataset to train a deep Convolutional Neural Network for estimating expression parameters of a 3D Morphable Model and combine it with an effective back-end emotion classifier. Our proposed framework runs at 50 frames per second and is capable of robustly estimating parameters of 3D expression variation and accurately recognizing facial expressions from in the-wild images. We present extensive experimental evaluation that shows that the proposed method outperforms the compared techniques in estimating the 3D expression parameters and achieves state-of-the-art performance in recognising the basic emotions from facial images, as well as recognising stress from facial videos.
更多查看译文
关键词
real-time facial expression recognition,human emotions analysis,affective computing,deep learning,visual facial data,human emotion recognition,single RGB image,facial videos,facial dynamics,3D pose variations,deep convolutional neural network,3D morphable model,facial expressions,facial images,in the-wild images,back-end emotion classifier,facial identities,facial appearance,facial 3D expression variation
AI 理解论文
溯源树
样例
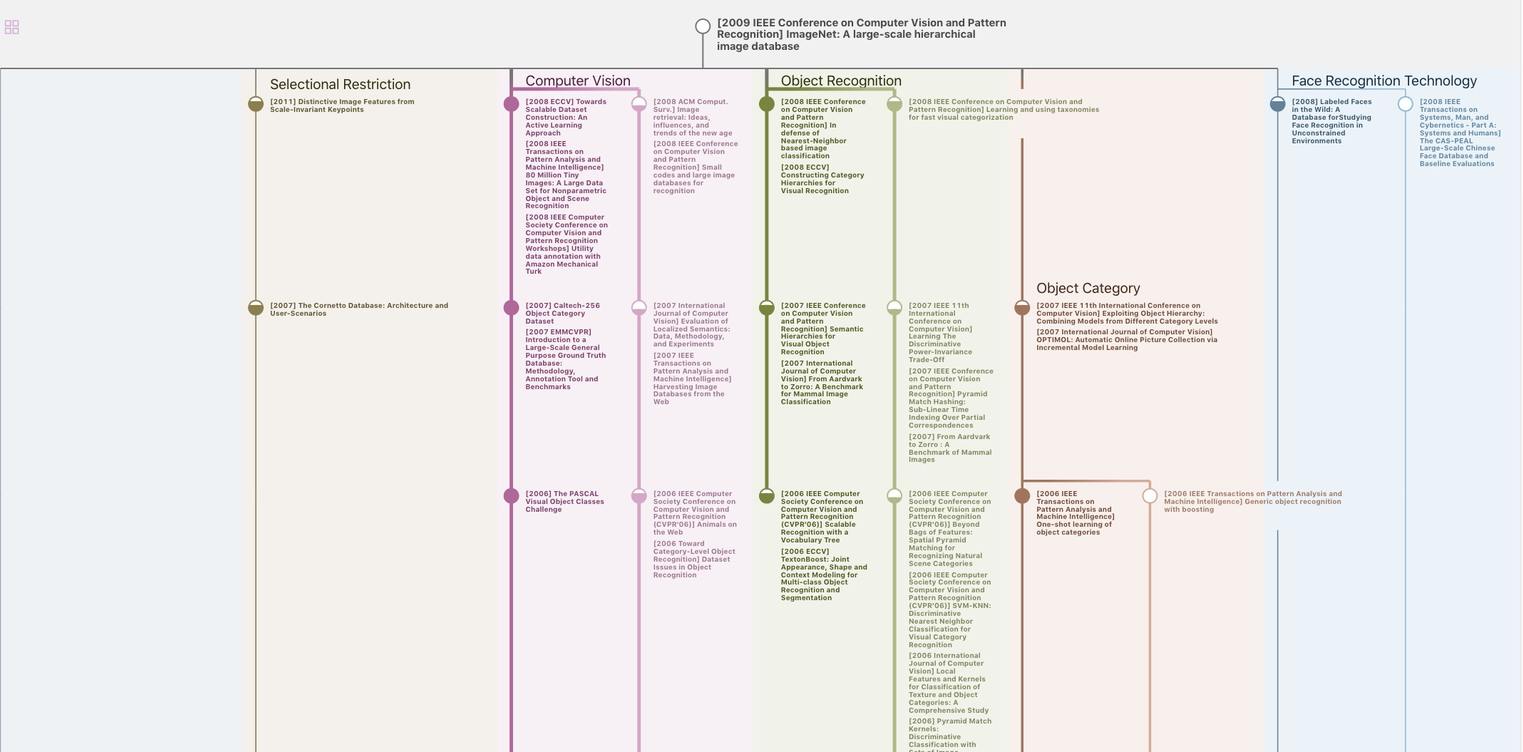
生成溯源树,研究论文发展脉络
Chat Paper
正在生成论文摘要