Process Knowledge Driven Change Point Detection for Automated Calibration of Discrete Event Simulation Models Using Machine Learning
arxiv(2020)
摘要
Initial development and subsequent calibration of discrete event simulation models for complex systems require accurate identification of dynamically changing process characteristics. Existing data driven change point methods (DD-CPD) assume changes are extraneous to the system, thus cannot utilize available process knowledge. This work proposes a unified framework for process-driven multi-variate change point detection (PD-CPD) by combining change point detection models with machine learning and process-driven simulation modeling. The PD-CPD, after initializing with DD-CPD's change point(s), uses simulation models to generate system level outputs as time-series data streams which are then used to train neural network models to predict system characteristics and change points. The accuracy of the predictive models measures the likelihood that the actual process data conforms to the simulated change points in system characteristics. PD-CPD iteratively optimizes change points by repeating simulation and predictive model building steps until the set of change point(s) with the maximum likelihood is identified. Using an emergency department case study, we show that PD-CPD significantly improves change point detection accuracy over DD-CPD estimates and is able to detect actual change points.
更多查看译文
关键词
discrete event simulation models,discrete event simulation,machine learning,calibration
AI 理解论文
溯源树
样例
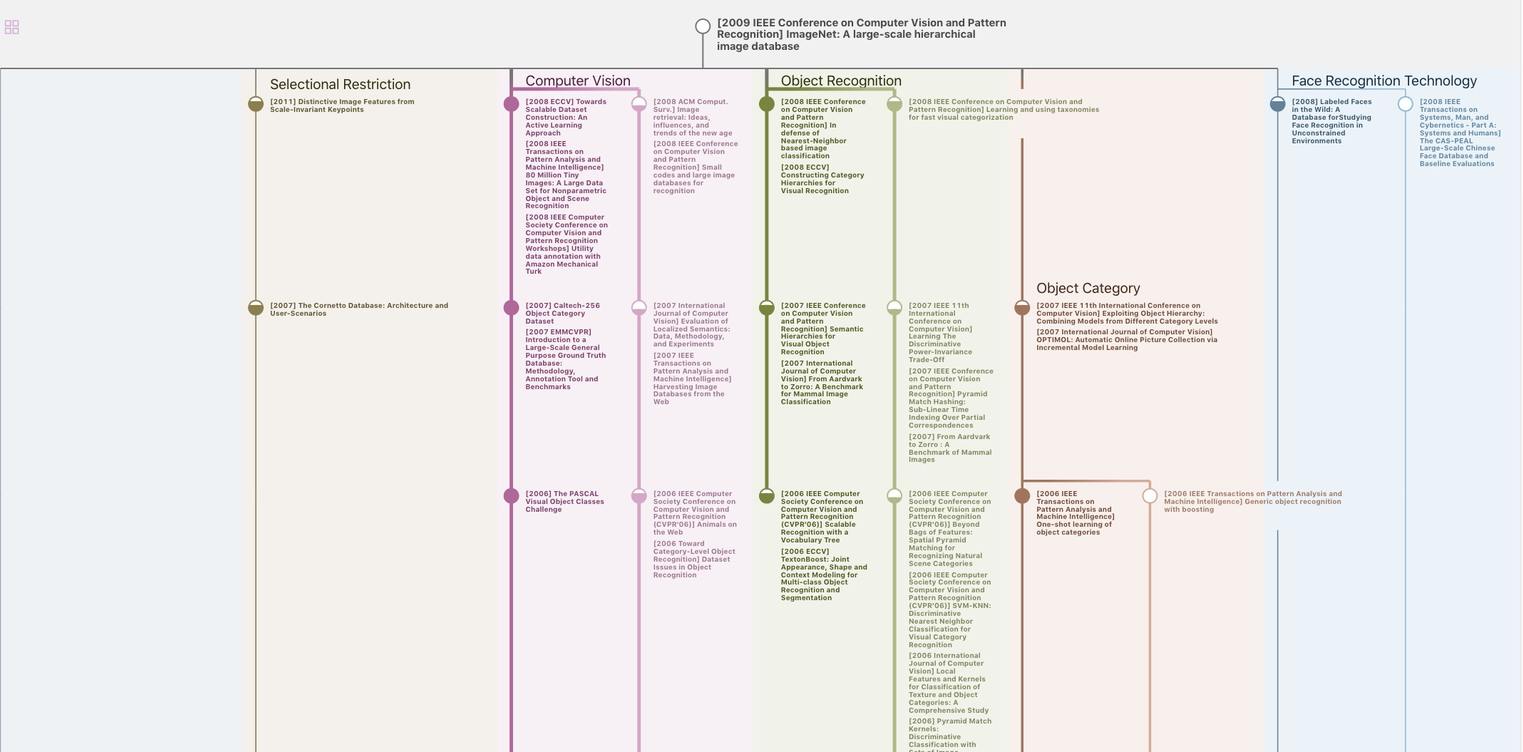
生成溯源树,研究论文发展脉络
Chat Paper
正在生成论文摘要