A Relational Gradient Descent Algorithm For Support Vector Machine Training
APOCS(2021)
摘要
We consider gradient descent like algorithms for Support Vector Machine (SVM) training when the data is in relational form. The gradient of the SVM objective can not be efficiently computed by known techniques as it suffers from the ``subtraction problem''. We first show that the subtraction problem can not be surmounted by showing that computing any constant approximation of the gradient of the SVM objective function is $\#P$-hard, even for acyclic joins. We, however, circumvent the subtraction problem by restricting our attention to stable instances, which intuitively are instances where a nearly optimal solution remains nearly optimal if the points are perturbed slightly. We give an efficient algorithm that computes a ``pseudo-gradient'' that guarantees convergence for stable instances at a rate comparable to that achieved by using the actual gradient. We believe that our results suggest that this sort of stability the analysis would likely yield useful insight in the context of designing algorithms on relational data for other learning problems in which the subtraction problem arises.
更多查看译文
关键词
relational gradient descent algorithm,support vector machine training
AI 理解论文
溯源树
样例
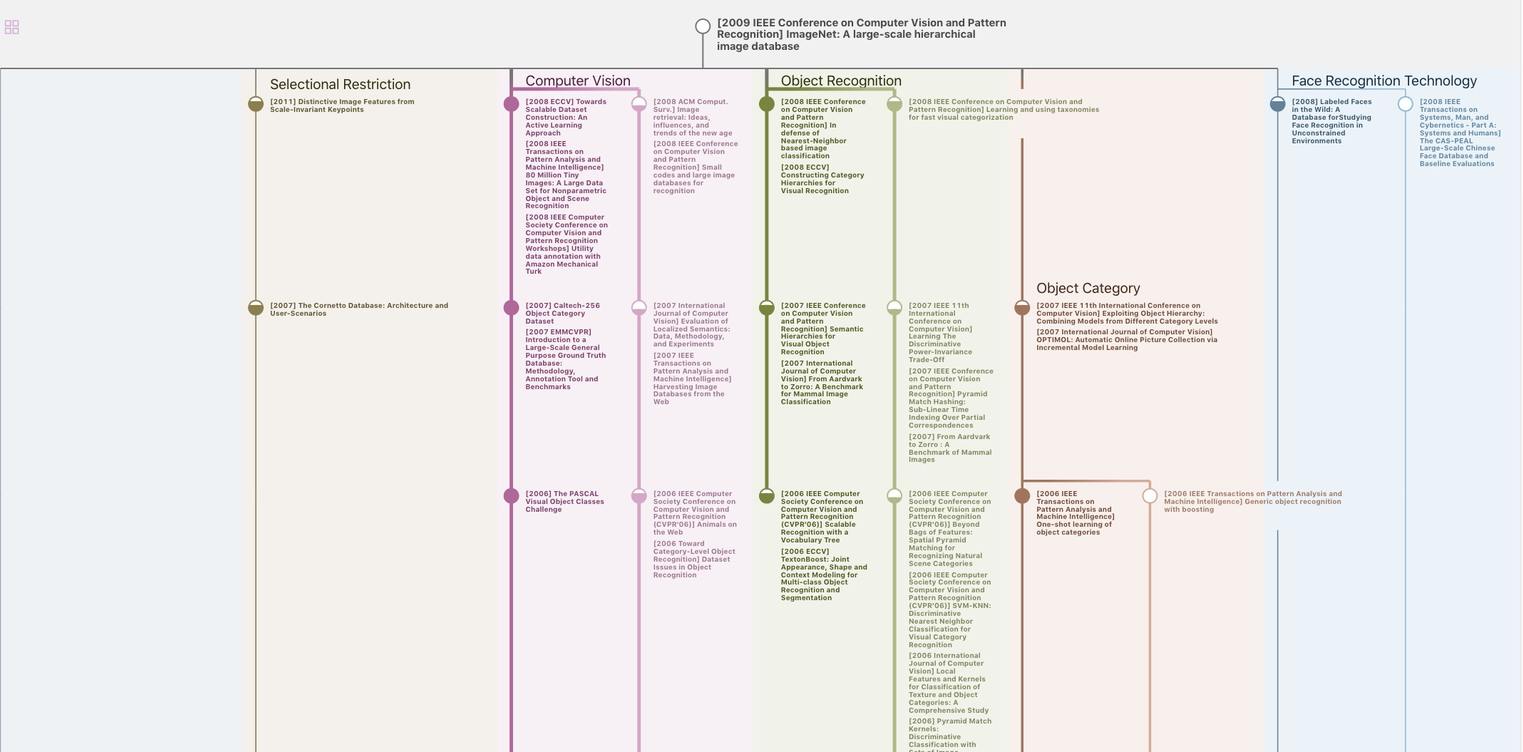
生成溯源树,研究论文发展脉络
Chat Paper
正在生成论文摘要