Robust Tensor Decomposition for Image Representation Based on Generalized Correntropy
Periodicals(2021)
摘要
AbstractTraditional tensor decomposition methods, e.g., two dimensional principal component analysis and two dimensional singular value decomposition, that minimize mean square errors, are sensitive to outliers. To overcome this problem, in this paper we propose a new robust tensor decomposition method using generalized correntropy criterion (Corr-Tensor). A Lagrange multiplier method is used to effectively optimize the generalized correntropy objective function in an iterative manner. The Corr-Tensor can effectively improve the robustness of tensor decomposition with the existence of outliers without introducing any extra computational cost. Experimental results demonstrated that the proposed method significantly reduces the reconstruction error on face reconstruction and improves the accuracies on handwritten digit recognition and facial image clustering.
更多查看译文
关键词
Tensors, Covariance matrices, Principal component analysis, Kernel, Linear programming, Robustness, Image reconstruction, Tensor decomposition, generalized correntropy, 2DSVD, reconstruction, recognition, clustering
AI 理解论文
溯源树
样例
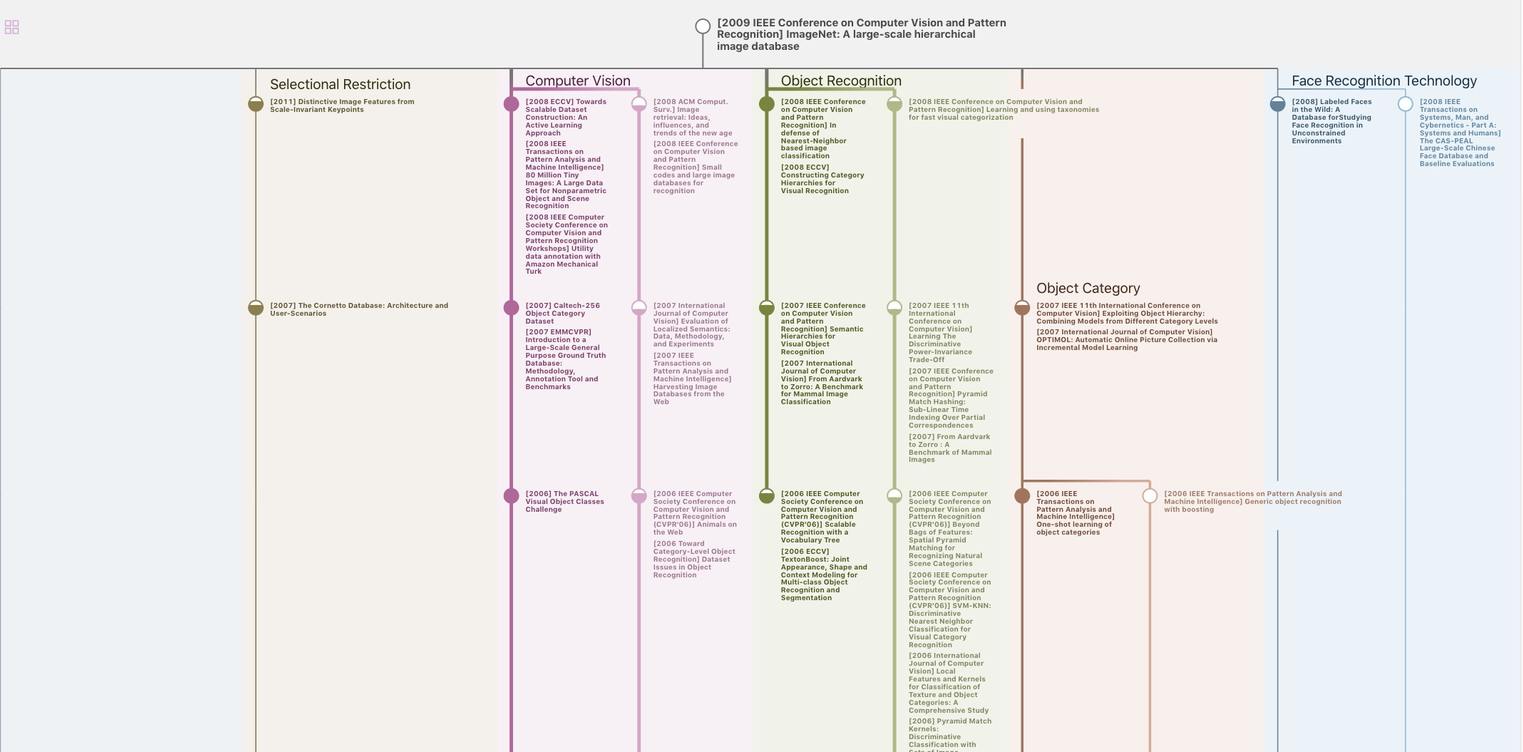
生成溯源树,研究论文发展脉络
Chat Paper
正在生成论文摘要