Compact Neural Representation Using Attentive Network Pruning
arxiv(2020)
摘要
Deep neural networks have evolved to become power demanding and consequently difficult to apply to small-size mobile platforms. Network parameter reduction methods have been introduced to systematically deal with the computational and memory complexity of deep networks. We propose to examine the ability of attentive connection pruning to deal with redundancy reduction in neural networks as a contribution to the reduction of computational demand. In this work, we describe a Top-Down attention mechanism that is added to a Bottom-Up feedforward network to select important connections and subsequently prune redundant ones at all parametric layers. Our method not only introduces a novel hierarchical selection mechanism as the basis of pruning but also remains competitive with previous baseline methods in the experimental evaluation. We conduct experiments using different network architectures on popular benchmark datasets to show high compression ratio is achievable with negligible loss of accuracy.
更多查看译文
关键词
compact,network
AI 理解论文
溯源树
样例
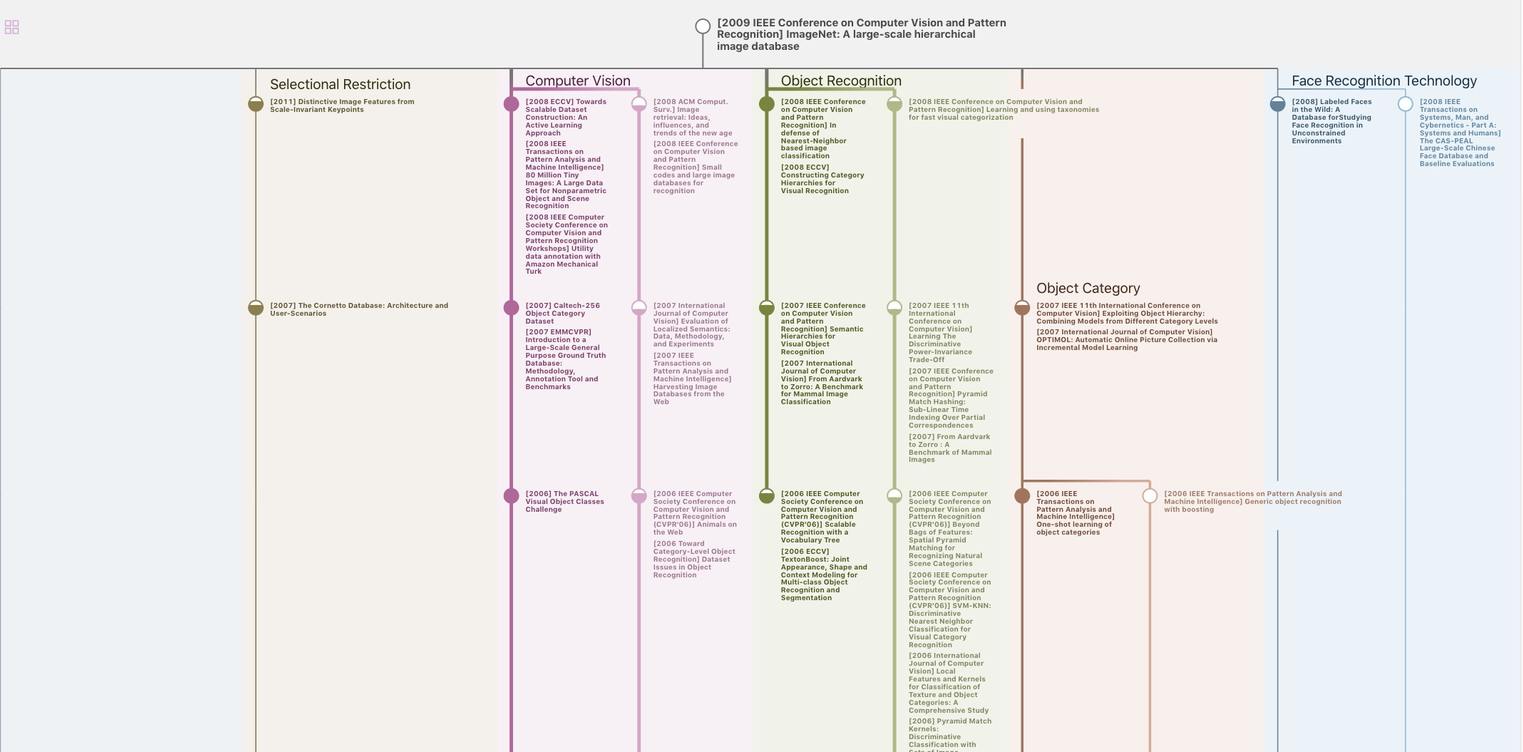
生成溯源树,研究论文发展脉络
Chat Paper
正在生成论文摘要