RotEqNet: Rotation-equivariant network for fluid systems with symmetric high-order tensors
Journal of Computational Physics(2022)
摘要
In the recent application of scientific modeling, machine learning models are largely applied to facilitate computational simulations of fluid systems. Rotation symmetry is a general property for most symmetric fluid systems. However, in general, current machine learning methods have no theoretical guarantee of Rotation symmetry. By observing an important property of contraction and rotation operation on high order symmetric tensors, we prove that the rotation operation is preserved via tensor contraction. Based on this theoretical justification, in this paper, we introduce Rotation-Equivariant Network (RotEqNet) to guarantee the property of rotation-equivariance for high order tensors in fluid systems. We implement RotEqNet and evaluate our claims with four case studies on various fluid systems. The property of error reduction and rotation-equivariance is verified in these case studies. Results are showing the high superiority of RotEqNet compared to traditional machine learning methods.
更多查看译文
关键词
Machine learning,Tensor analysis,Rotation-equivariant,Fluid systems
AI 理解论文
溯源树
样例
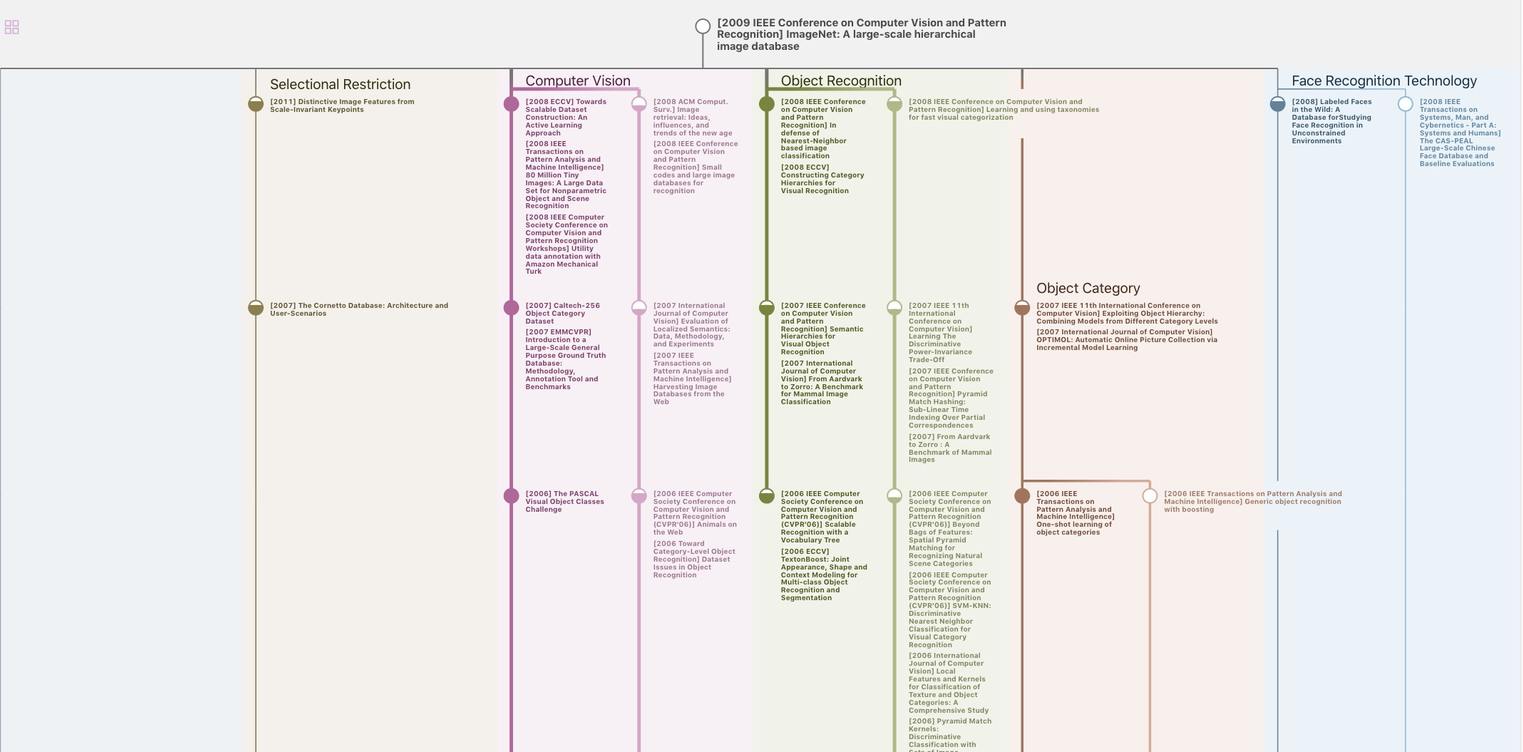
生成溯源树,研究论文发展脉络
Chat Paper
正在生成论文摘要