Generalized Unitarily Invariant Gauge Regularization for Fast Low-Rank Matrix Recovery.
IEEE Transactions on Neural Networks and Learning Systems(2021)
摘要
Spectral regularization is a widely used approach for low-rank matrix recovery (LRMR) by regularizing matrix singular values. Most of the existing LRMR solvers iteratively compute the singular values via applying singular value decomposition (SVD) on a dense matrix, which is computationally expensive and severely limits their applications to large-scale problems. To address this issue, we present a generalized unitarily invariant gauge (GUIG) function for LRMR. The proposed GUIG function does not act on the singular values; however, we show that it generalizes the well-known spectral functions, including the rank function, the Schatten- p quasi-norm, and logsum of singular values. The proposed GUIG regularization model can be formulated as a bilinear variational problem, which can be efficiently solved without computing SVD. Such a property makes it well suited for large-scale LRMR problems. We apply the proposed GUIG model to matrix completion and robust principal component analysis and prove the convergence of the algorithms. Experimental results demonstrate that the proposed GUIG method is not only more accurate but also much faster than the state-of-the-art algorithms, especially on large-scale problems.
更多查看译文
关键词
Minimization,Computational modeling,Complexity theory,Learning systems,Principal component analysis,Data models,Convergence
AI 理解论文
溯源树
样例
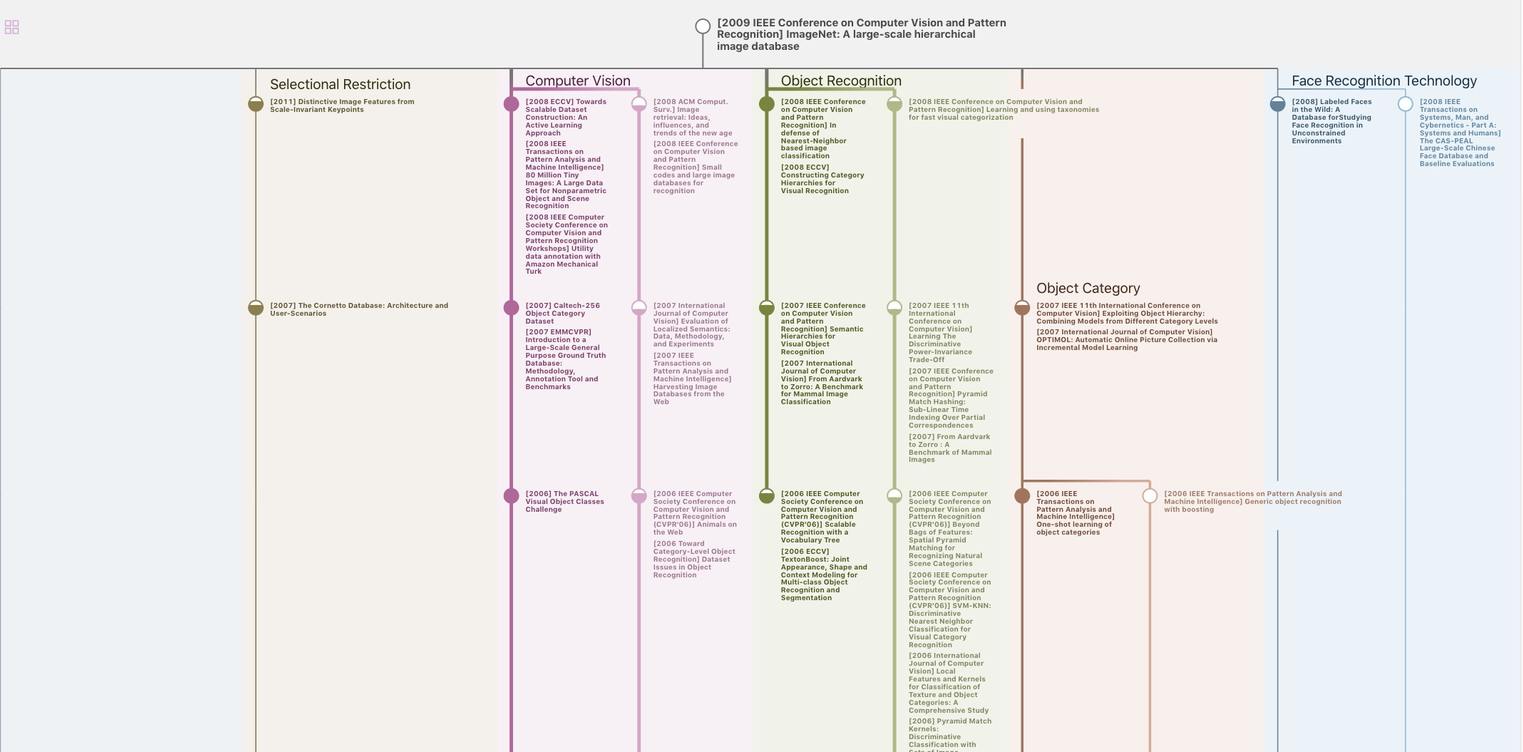
生成溯源树,研究论文发展脉络
Chat Paper
正在生成论文摘要